Projects
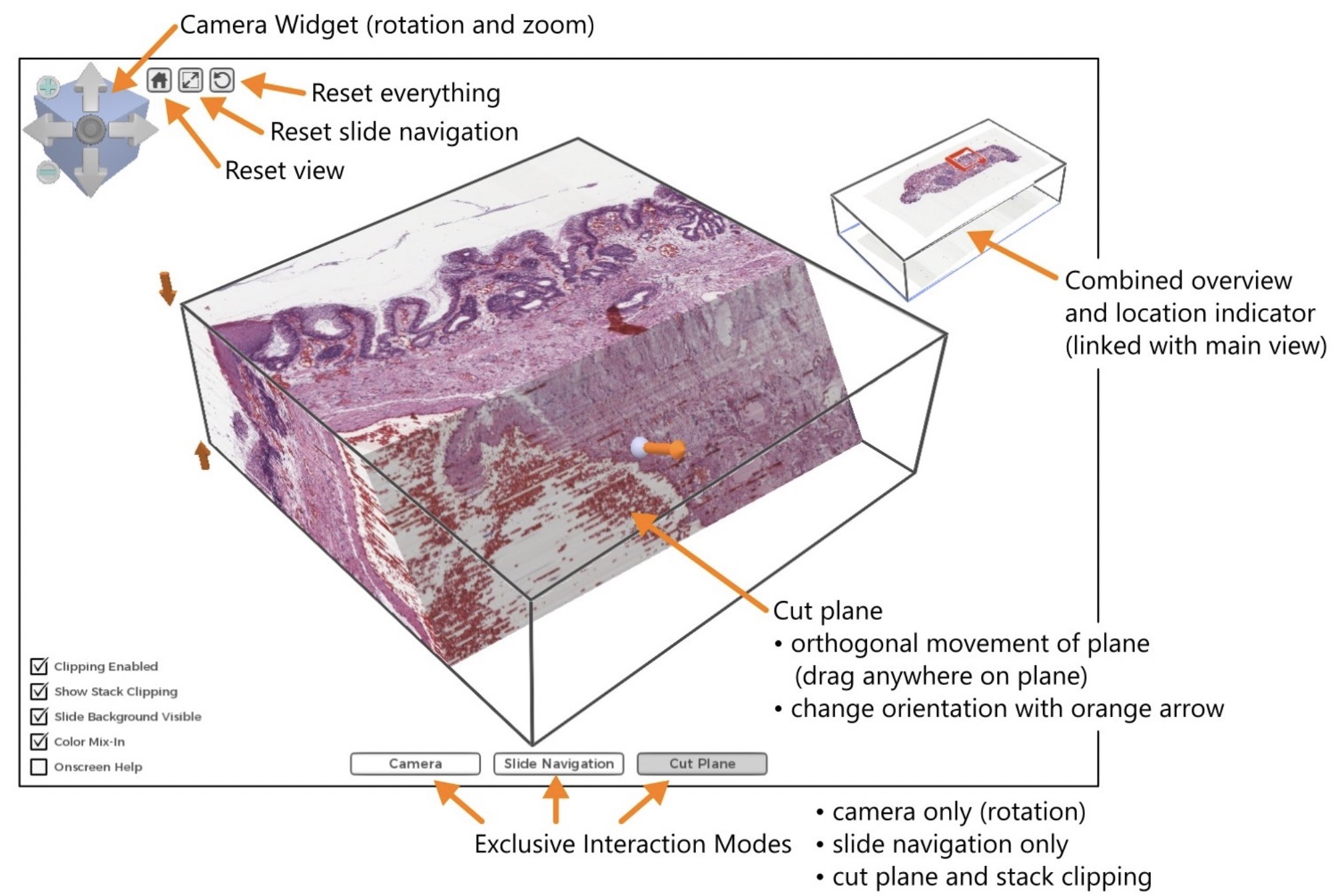
3D Histology, Interactive High-resolution Volume Rendering for Pathology
Histology, the medical analysis at microscopic level of tissue specimen from the human body, is a cornerstone in medical research. Digitization of the microscopy images opens up exciting possibilities for visualization and image analysis.
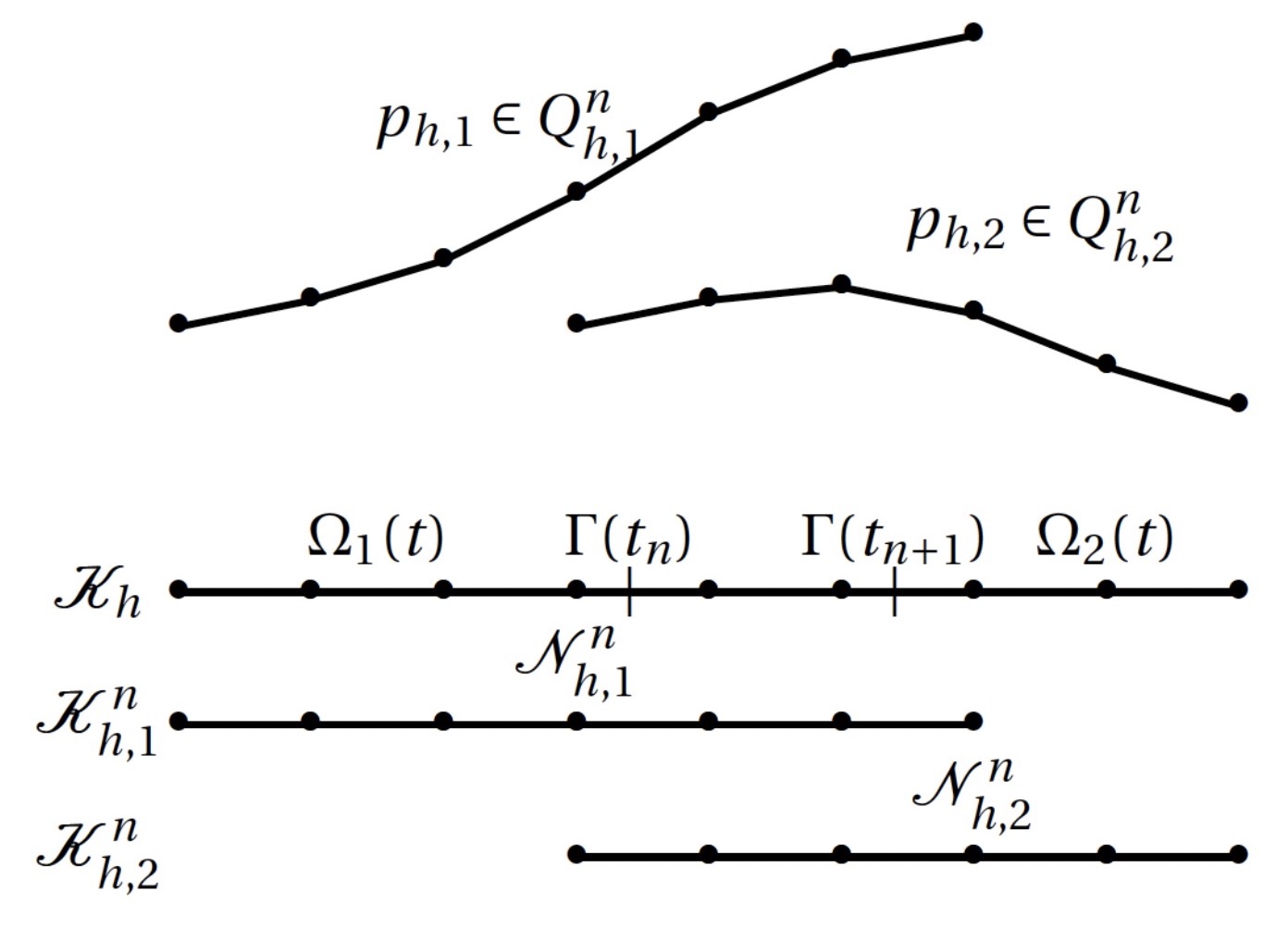
A Cut Finite Element Method for Incompressible Two-Phase Navier-Stokes Flows
A Cut Finite Element Method (CutFEM) for the time-dependent Navier-Stokes equations involving two immiscible incompressible fluids is developed. The numerical method is able to accurately approximate a discontinuous pressure and a weak discontinuous velocity field across evolving interfaces without requiring the mesh to be fitted to the domain or regularizing the discontinuities.
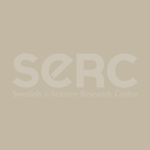
A key role predicted of for fast synaptic plasticity in working memory in the cortex
We tested whether a cortical spiking neural network model with fast Hebbian synaptic plasticity could learn a multi-item WM task (word list learning). The model could indeed reproduce human cognitive phenomena, while being simultaneously compatible with experimental data on structure, connectivity, and neurophysiology of the underlying cortical tissue.
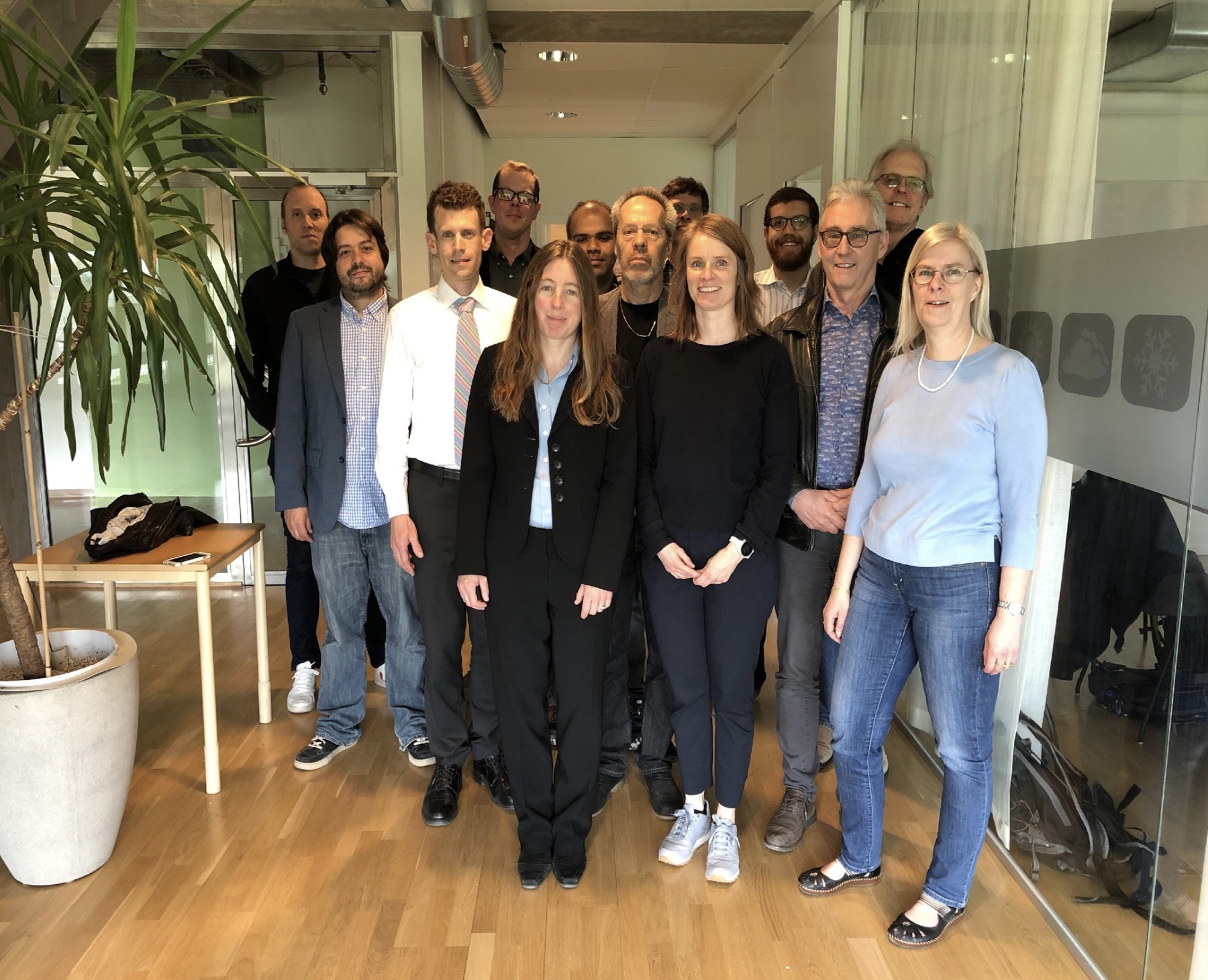
ABL: LES Workshop – May 2019
A series of presentations were given over a span of two days to identify the state-of-the-art of large- eddy simulations (LES)
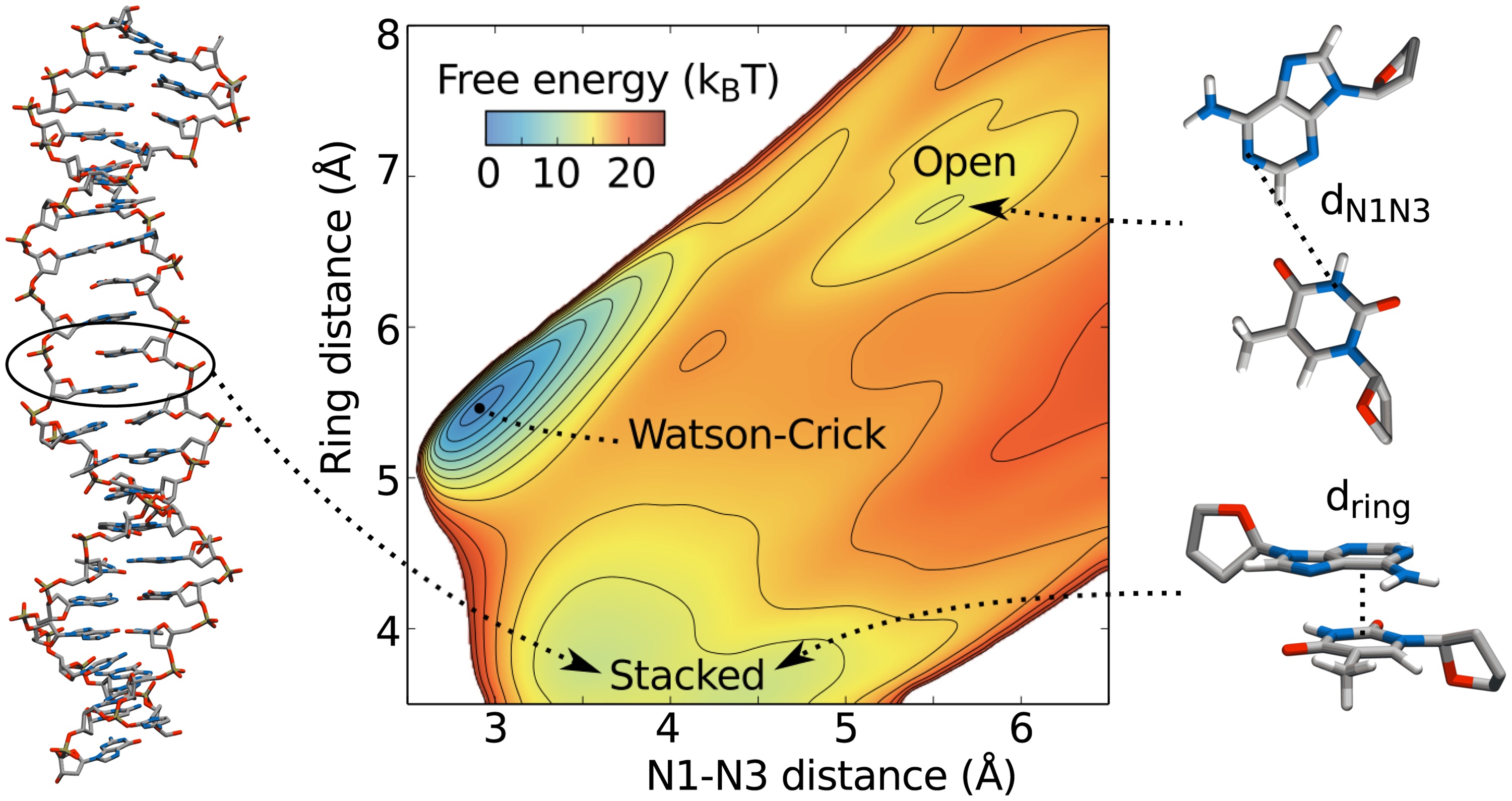
Accelerated sampling of conformational transitions in DNA
Molecular dynamics is a powerful technique to study the behavior of molecules, and in particular bio-molecules such as proteins and DNA. However, the time scales of biologically important events are often much longer than what can be reached with simulations.
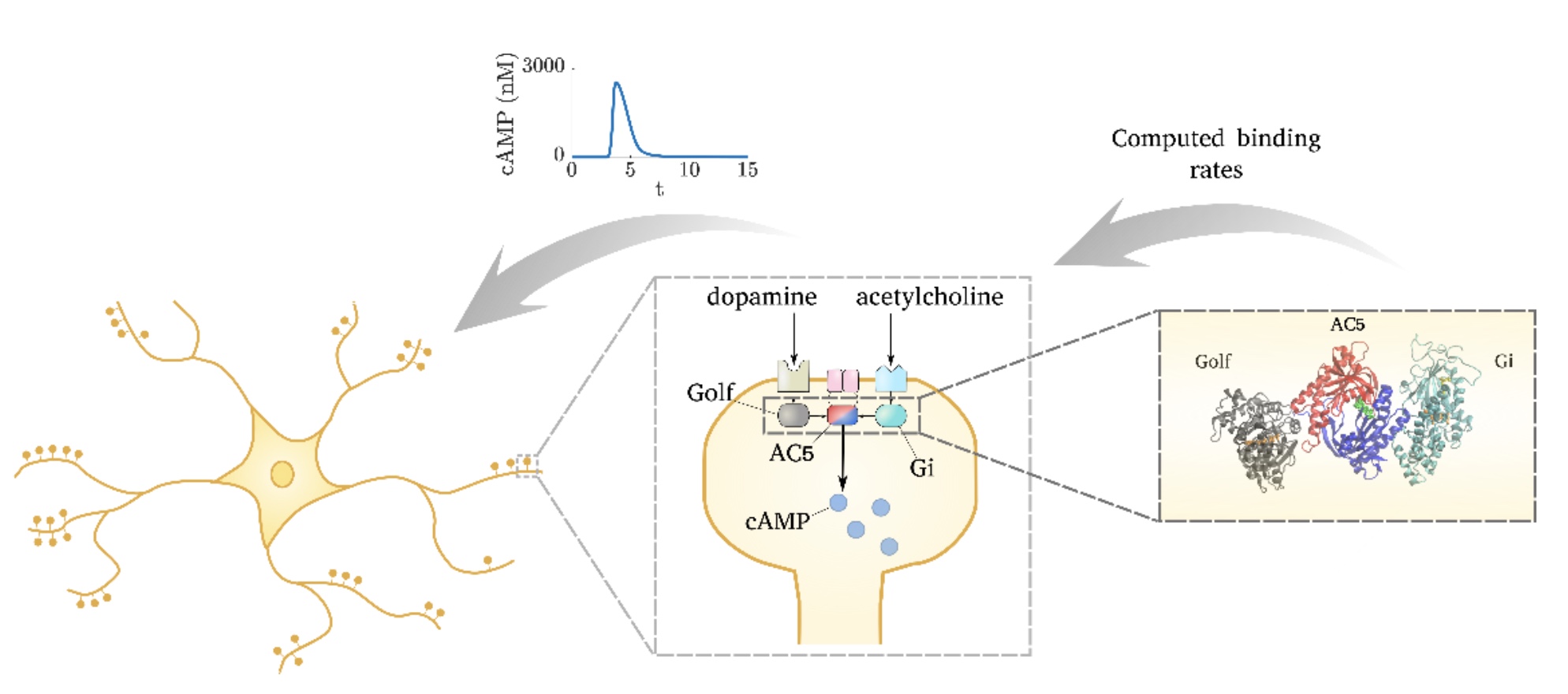
Adenylyl cyclase 5 in striatal neurons confers the ability to detect coincident neuromodulatory signals
We have demonstrated that molecular-level simulations can inform subcellular models of synaptic plasticity. Several computational tools, from molecular dynamics and Brownian dynamics simulations to bioinformatics approaches, were combined to constrain a kinetic model of the adenylyl cyclase type 5 (AC5)-dependent signaling system in the striatum.
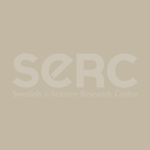
AI-centered visual analytics of histology
This project aims to merge AI techniques with visual exploration.
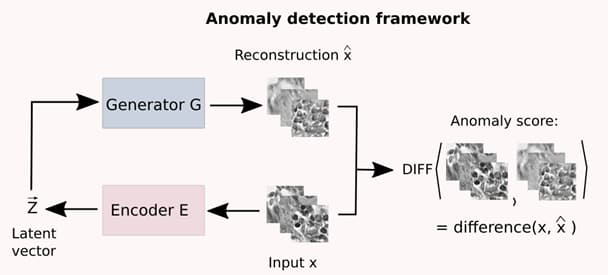
AIVisualization subproject: Using Machine Learning for anomaly detection in medical imagery
Milda Pocevičiūtė, Gabriel Eilertsen, Claes Lundström, “Unsupervised anomaly detection in digital pathology using GANs”, IEEE International Symposium on Biomedical Imaging, 2021.
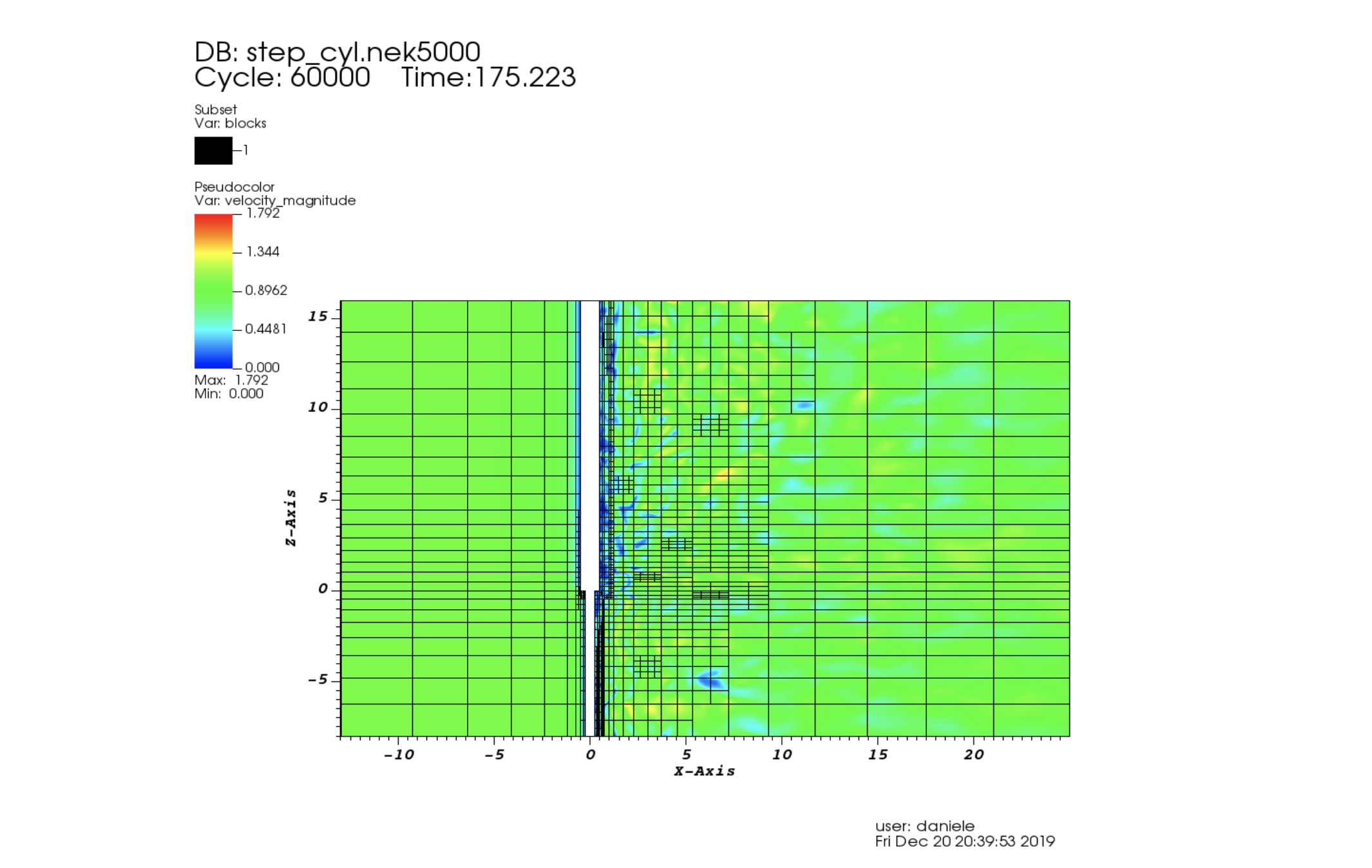
AMR for Nek5000
When we study turbulent chaotic flows in complicated geometries our experience could not be sufficient to suggest where a more refined mesh is required.
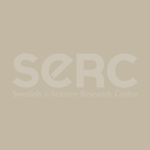
Analytic Imaging Diagnostics Arena (AIDA) funded
AIDA is a cross-disciplinary and cross-sectoral collaboration aiming for large-scale usefulness from Artificial Intelligence (AI) in healthcare.
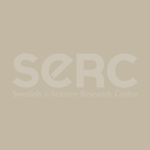
Are NVIDIA Tensor cores good for HPC?
The NVIDIA Volta GPU microarchitecture introduces a specialized unit, called Tensor Core that performs one matrix-multiply-and-accumulate on 4×4 matrices per clock cycle.
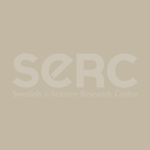
Artificial intelligence for diagnosis and grading of prostate cancer in biopsies
We published an article entitled “Artificial intelligence for diagnosis and grading of prostate cancer in biopsies: a population-based, diagnostic study” in Lancet Oncology (https://doi.org/10.1016/S1470-2045(19)30738-7; impact factor=35).
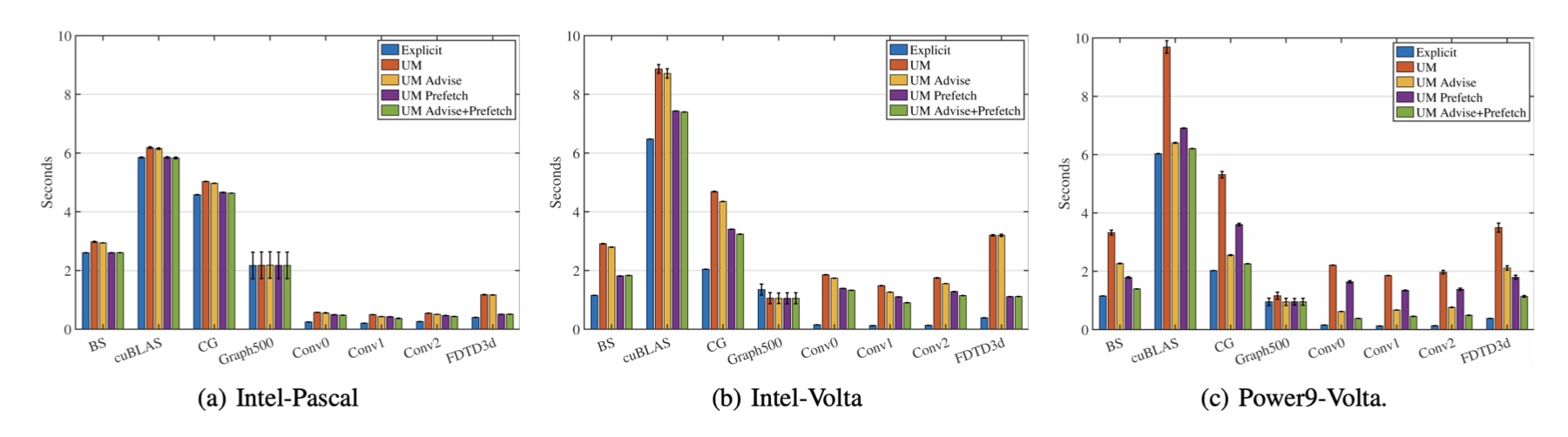
Assessing the Performance Improvements of new Features in CUDA Unified Memory
Recently, leadership supercomputers are becoming increasingly heterogeneous. For instance, the two fastest supercomputers in the world, Summit and Sierra, are both equipped with Nvidia V100 GPUs for accelerating workloads.
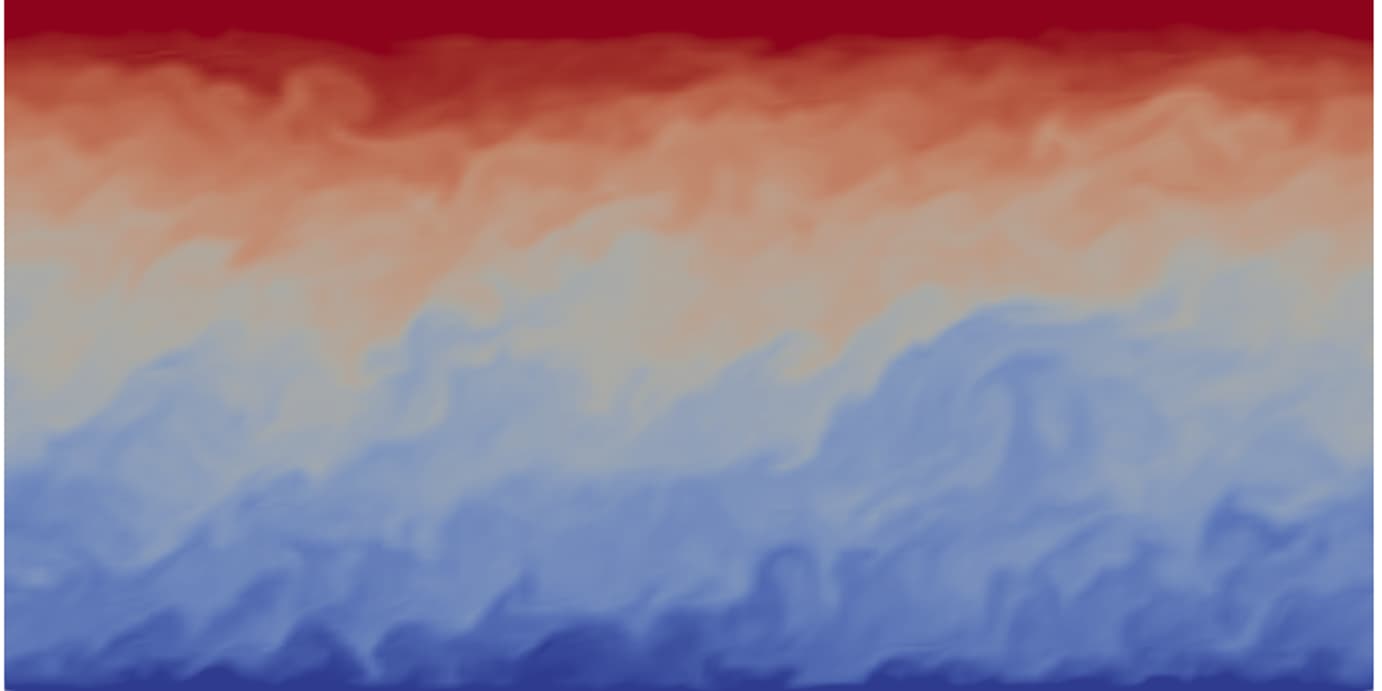
Atmospheric boundary layers
Recent developments in large-eddy simulation of thermally stratified atmospheric boundary layers (ABLs) are achieved in collaboration by Drs. Mohanan, Mukha, Brethouwer, Schlatter, Henningson and Svensson.
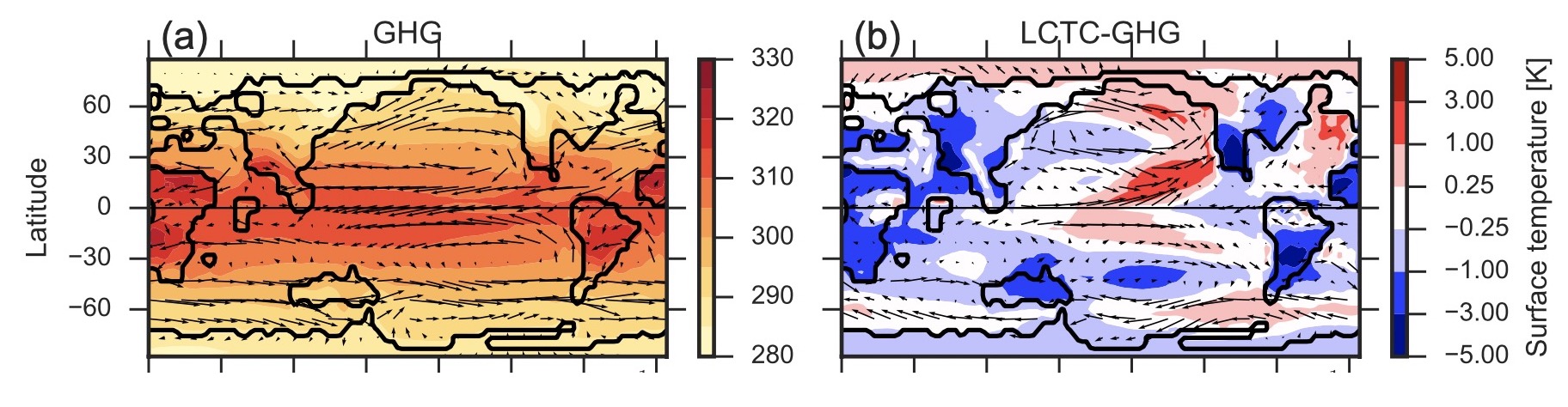
Atmospheric circulation in a much warmer Earth, simulations using alternative warming scenarios for the Eocene
It is possible to study this period with climate models, but to obtain a reasonable global match between model surface temperature and proxy reconstructions extremely high atmospheric CO2concentrations or a reduction in global cloud albedo is needed. In this work, these two methods are examined.
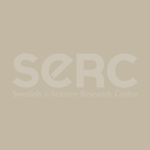
Automatic workflow, data collection, and development of open-data infrastructure
For DCMD activities in data exploration and visualization, we need to address generation, collection, storing, and organizing data via research on automatic workflow, data collection for materials data, and development of an open-data infrastructure. All activities lead to necessary insights and software to do complex simulations within materials physics and molecular chemistry.
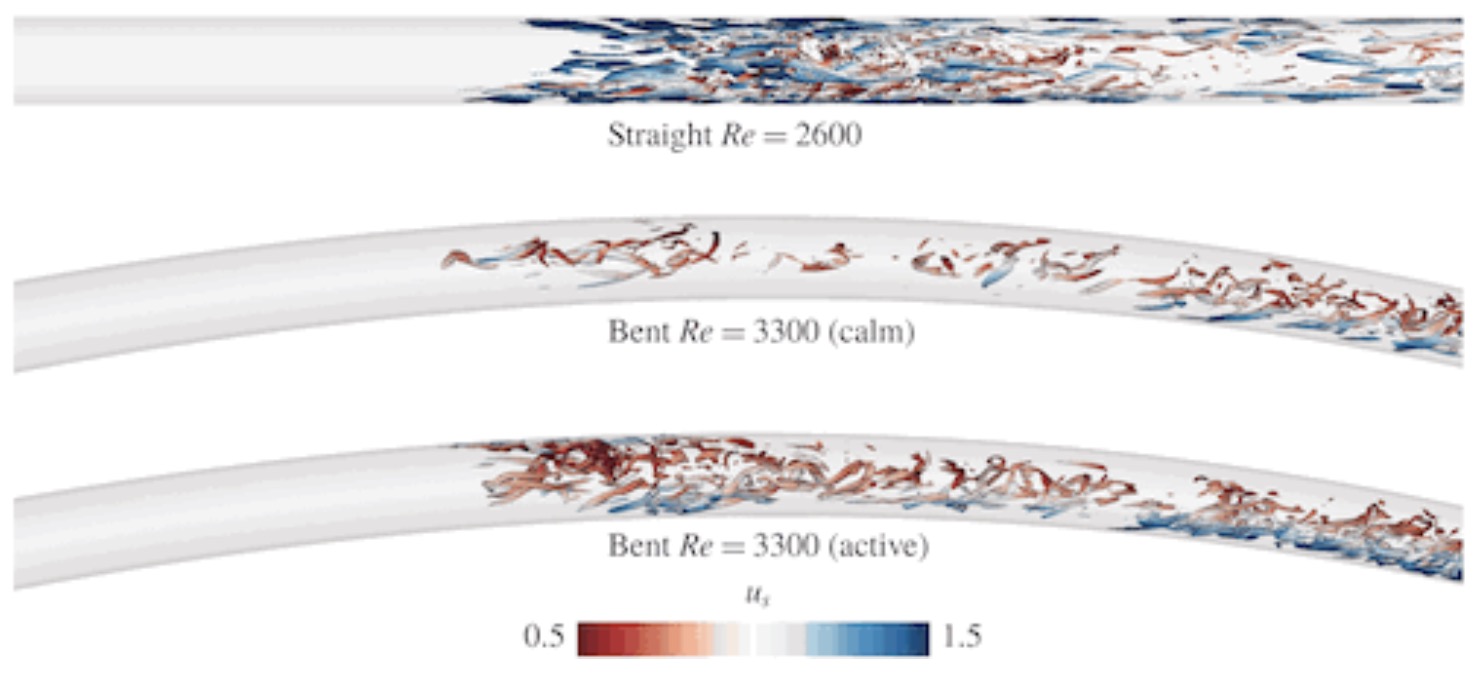
Bent pipe flow
The included simulations include various relevant code features, including a scalable checkpointing and restarting procedure for calculating adjoint sensitivities for optimal initial conditions, plus advanced postprocessing and visualization of the incipient turbulence.
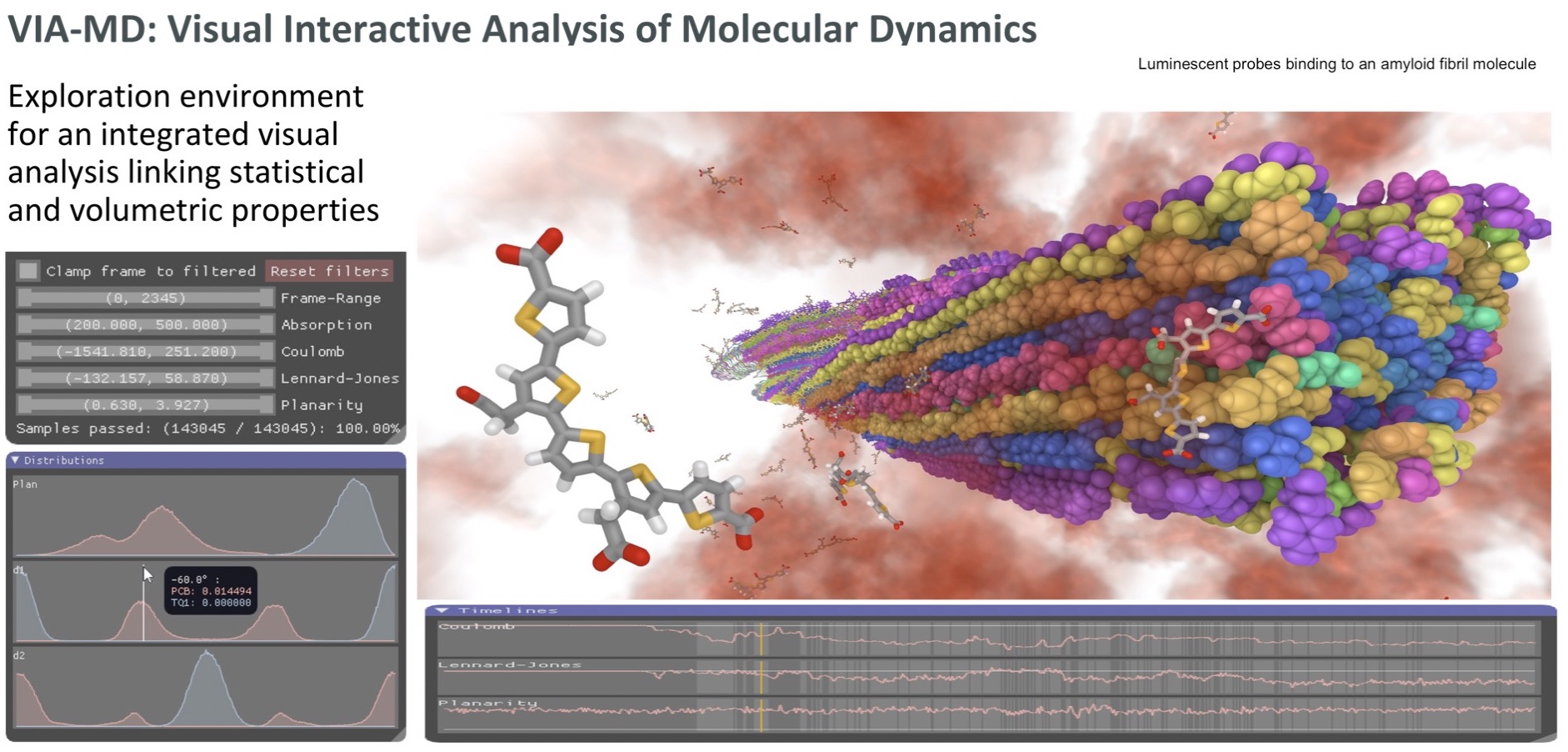
Binding sites for luminescent amyloid biomarkers from non-biased molecular dynamics simulations
A visual analytics environment VIA-MD(visual interactive analysis of molecular dynamics) tailored for large-scale spatio-temporal molecular dynamics simulation data has been developed. A key concept of the environment is to link interactive 3D exploration of geometry and statistical analysis.
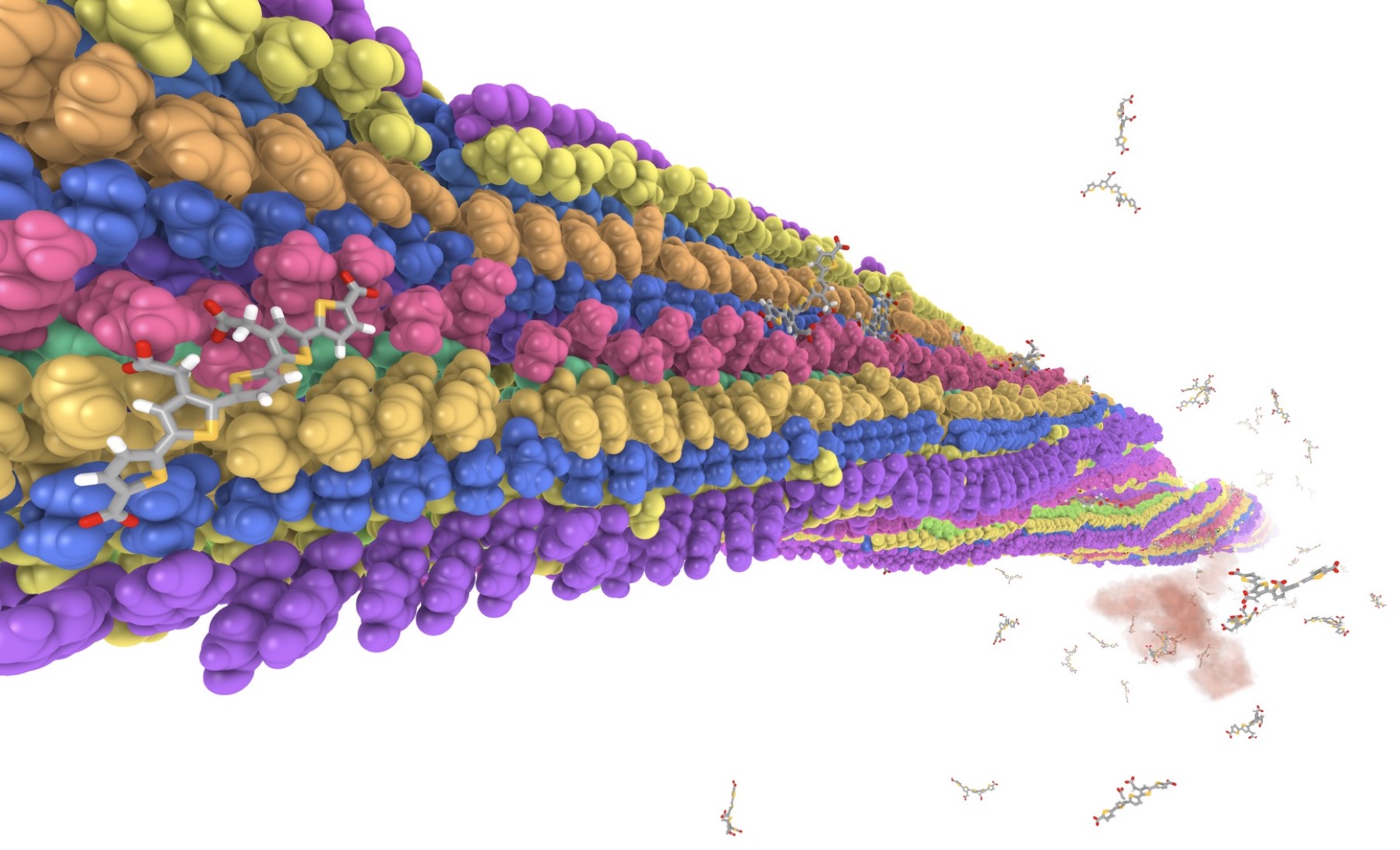
Binding sites for luminescent amyloid biomarkers from non-biased molecular dynamics simulations
A very stable binding site for the interaction between a pentameric oligothiophene and an amyloid- beta(1–42) fibril has been identified by means of non-biased molecular dynamics simulations.
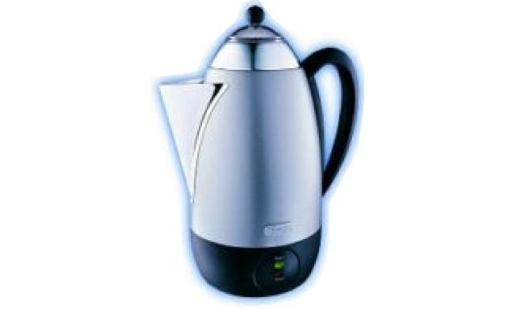
Bioinformatics Highlight: Assessing protein mass spectrometry data using Percolator – how to weed out valuable information from the noise
Mass spectrometry (MS) is currently the most effective way to analyze protein on a large scale, and hence one of the most important tools for answering those questions. There are still however difficult challenges in analysing the wealth of data MS-based experiments produce.
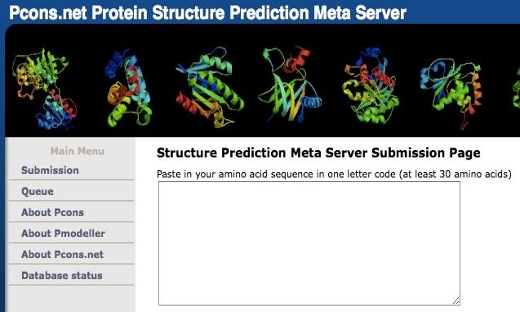
Bioinformatics Highlight: Protein structure prediction — state-of-the-art methods proven in contests
Several scientists in the Bioinformatics community study and develop methods for protein structure prediction.
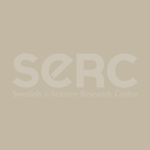
Brain network architecture and dynamics of short- and long-term memory
In this project we intend to study cortical network phenomena accompanying brain plasticity effects relevant to short- and long-term memory processes. The overarching aim is to enhance e-science approaches for studying brain networks developed at KTH and KI, and inject corresponding informatics workflows into the environments at SUBIC. PH plans to advance an existing spiking and non-spiking large-scale neural network models to simulate memory phenomena in close collaboration with AL.
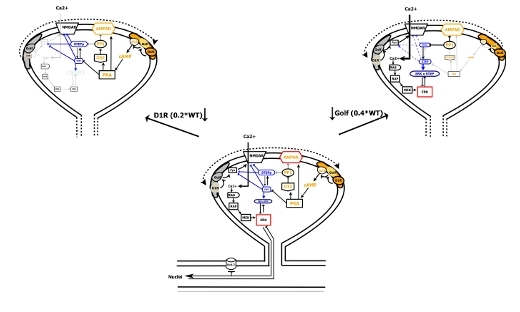
Brain-IT Highlight: D1R-Golf signaling modules segregate into compartments
The development of a large signaling model that takes into consideration the existence of at least two D1R-Golf signaling compartments explains the data pattern. …
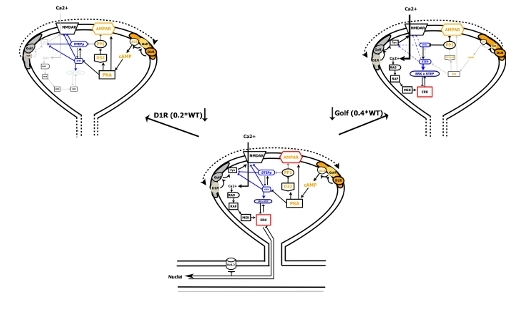
Brain-IT Highlight: D1R-Golf signaling modules segregate into compartments
The development of a large signaling model that takes into consideration the existence of at least two D1R-Golf signaling compartments explains the data pattern. …
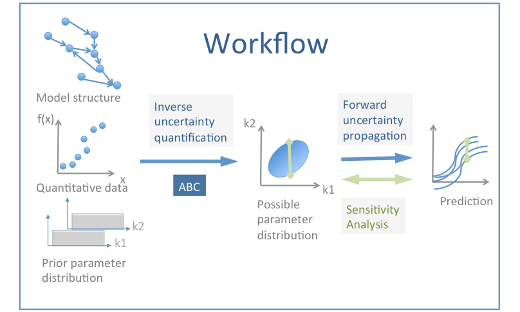
Brain-IT Highlight: Workflows for the estimation of model parameters
When modeling subcellular signaling pathways, experimental data are integrated into a precise and structured framework from which it is possible to make predictions that could be tested experimentally. …
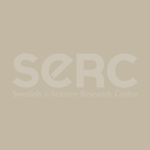
Brain-like approach to Machine Learning
The main aim of this project is to advance the development of hierarchical brain-like network architectures for holistic pattern discovery drawing from the computational insights into neural information processing in the brain in the context of sensory perception, multi-modal sensory fusion, sequence learning and memory association among others
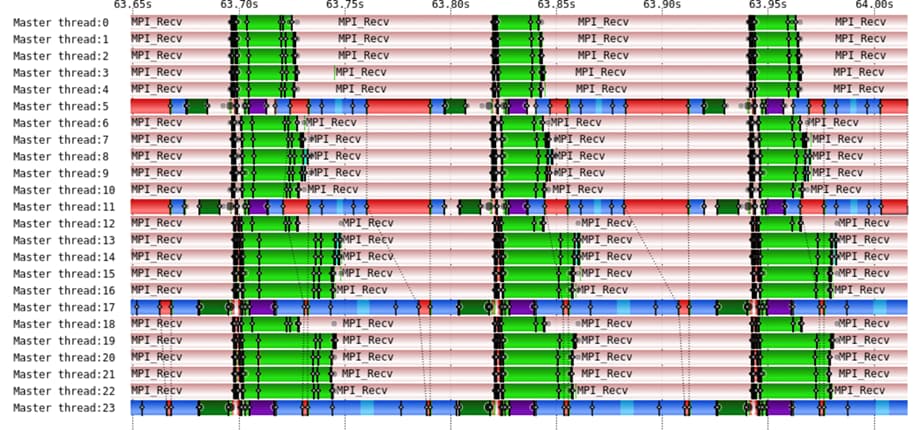
Breaking down GROMACS Parallel Performance
In this work, we quantified GROMACS parallel performance using different configurations, HPC systems, and FFT libraries (FFTW, Intel MKL FFT, and FFT PACK).
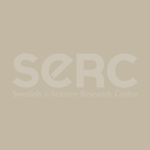
Breakthrough in Big Data: 16X performance gains for Hadoop, delivering over 1.2 million operations per second
At USENIX FAST 2017, researchers from RISE SICS and KTH, in collaboration with Spotify and Oracle, presented a next-generation distribution of Apache Hadoop File System, called HopsFS, that delivers a quantum leap in both the cluster-size and throughput compared to Hadoop clusters.
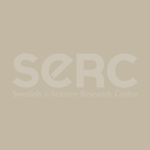
Cancer screening – natural history, prediction and microsimulation
We will continue work on natural history modelling for cervical, breast and prostate cancer. Methods include HPC-intensive calibrations of simulation likelihoods using Bayesian methods and optimisation procedures for expensive or imprecise objective functions (Laure, Jauhiainen at AstraZenenca, Uncertainty Quantification with SeRC-Brain-IT). We will investigate a computational framework for storage and analysis of m icro-simulation experiments for calibration and prediction (Laure, Dowling).
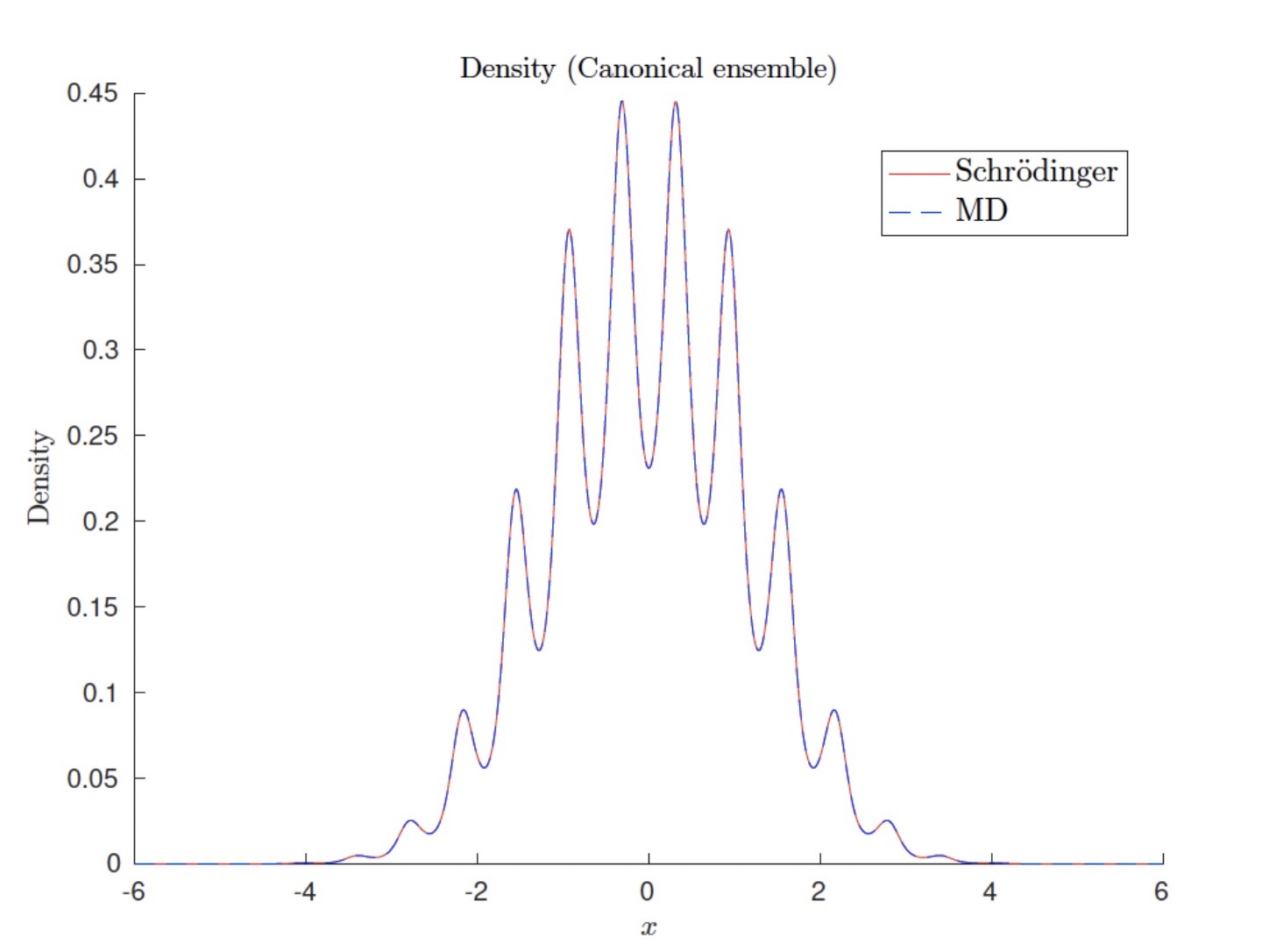
Canonical quantum observables for molecular systems approximated by ab initio molecular dynamics
It is known that ab initio molecular dynamics based on the electron ground state eigenvalue can be used to approximate quantum observables in the canonical ensemble when the temperature is low compared to the first electron eigenvalue gap.
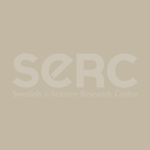
CausalHealthcare
In this project, we aim to develop machine learning tools for causal discovery and causal inference, along with tools for visualizing large causal structures to a human to increase the interpretability of the structure.
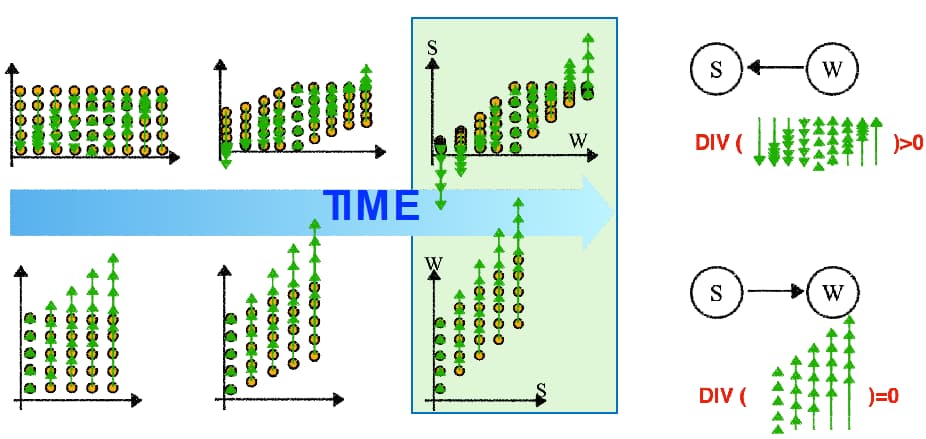
CausalHealthcare subproject: Using principles from Physics to disambiguate the causal direction between variables in passively recorded data
Ruibo Tu, Kun Zhang, Hedvig Kjellström, and Cheng Zhang. Optimal transport for causal discovery.
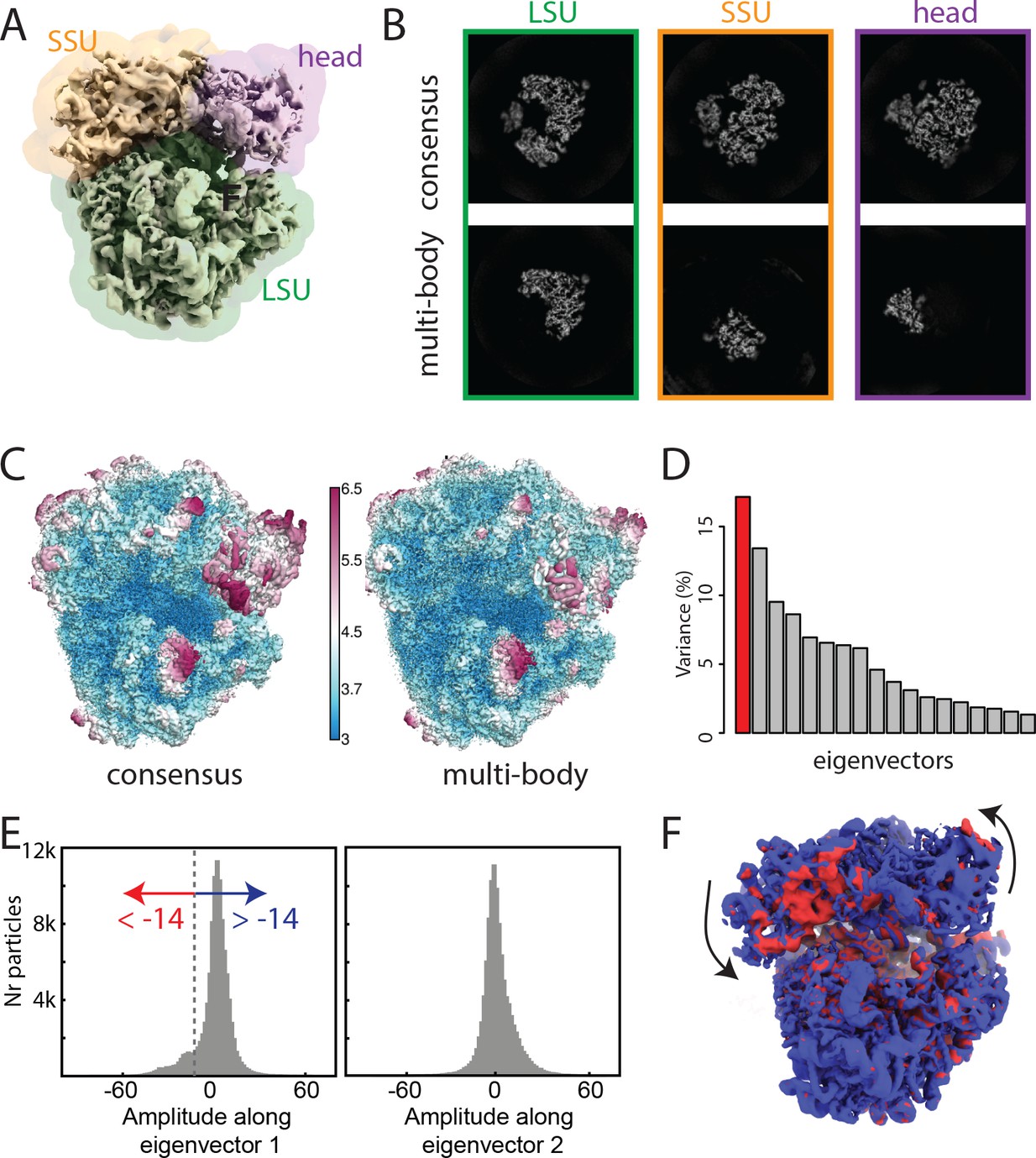
Characterization of molecular motion in Cryo-EM image reconstruction
In collaboration with the Laboratory of Molecular Biology (Cambridge, UK), the SeRC Molecular Simulation community has continued their work on new reconstruction algorithms for cryo-electron microscopy.
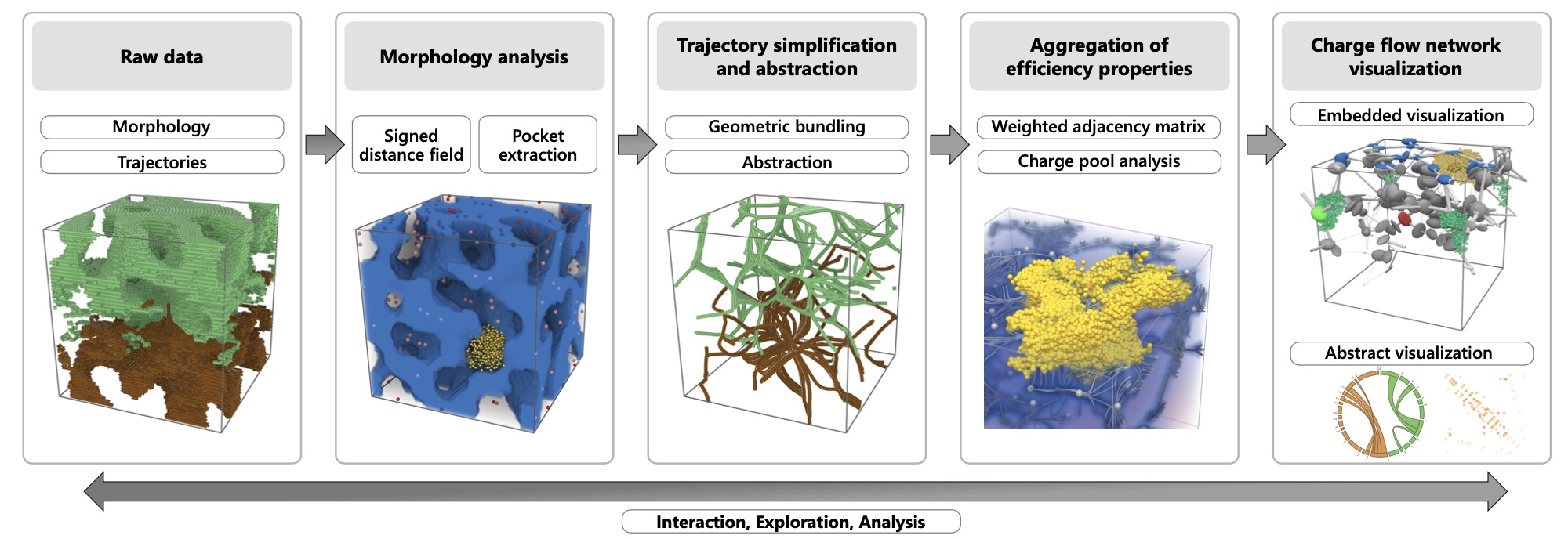
Charge-flow network for interactive exploration of data from organic solar cell simulations
In the field of organic electronics, understanding complex material morphologies and their role in efficient charge transport in solar cells is extremely important.
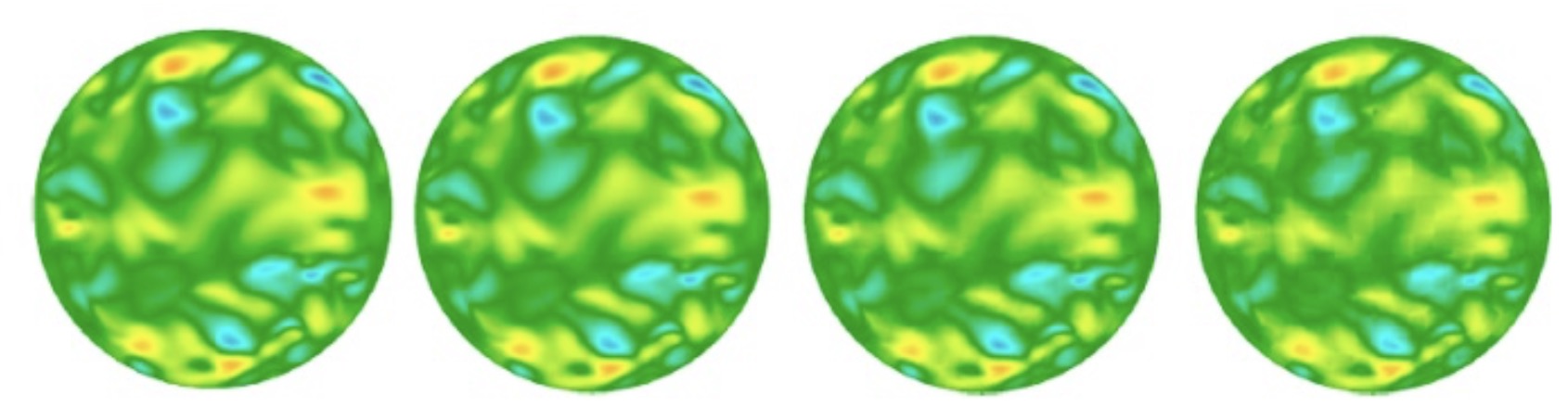
Data compression in Nek5000 (with SESSI)
Instantaneous in-plane horizontal velocity in a turbulent Pipe flow, showing progressively increasing compression ration from 0% (uncompressed), 88.5%, 95.7% and 97.5% using our discrete Legendre Transform (DLT) technique.
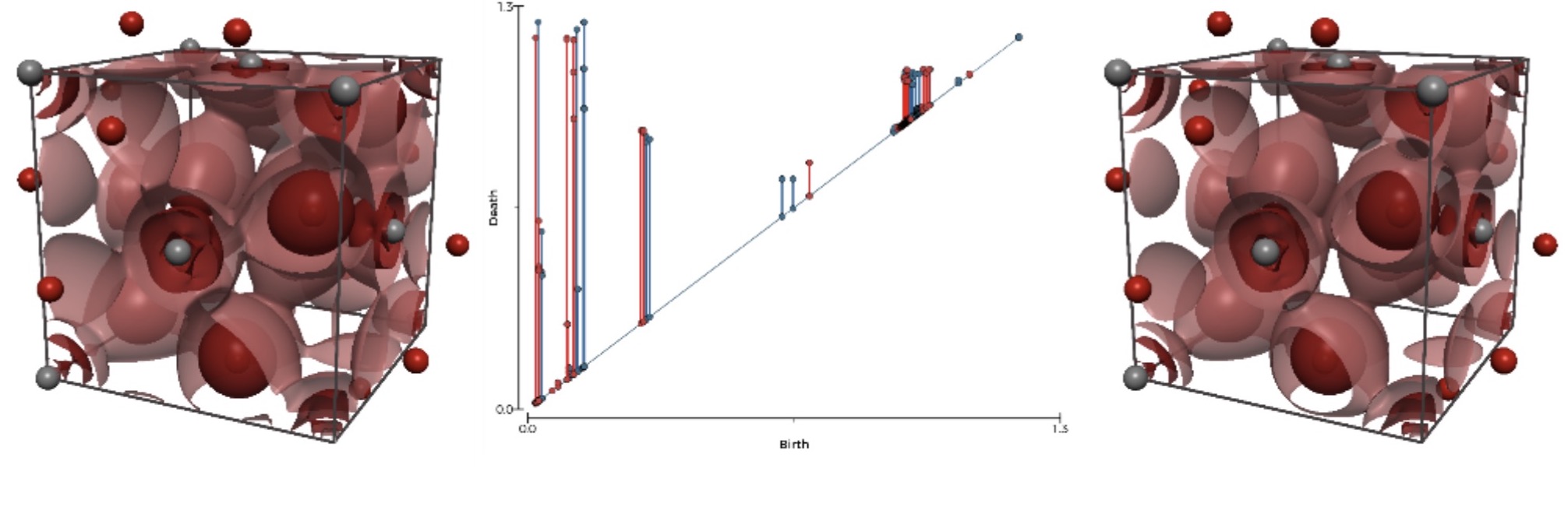
Data exploration and visualization
Method development is needed into data exploration and visualization of the generated materials data. This is a new and exciting field of multidisciplinary research where data science meets computational materials physics. Specific activities in this group include:
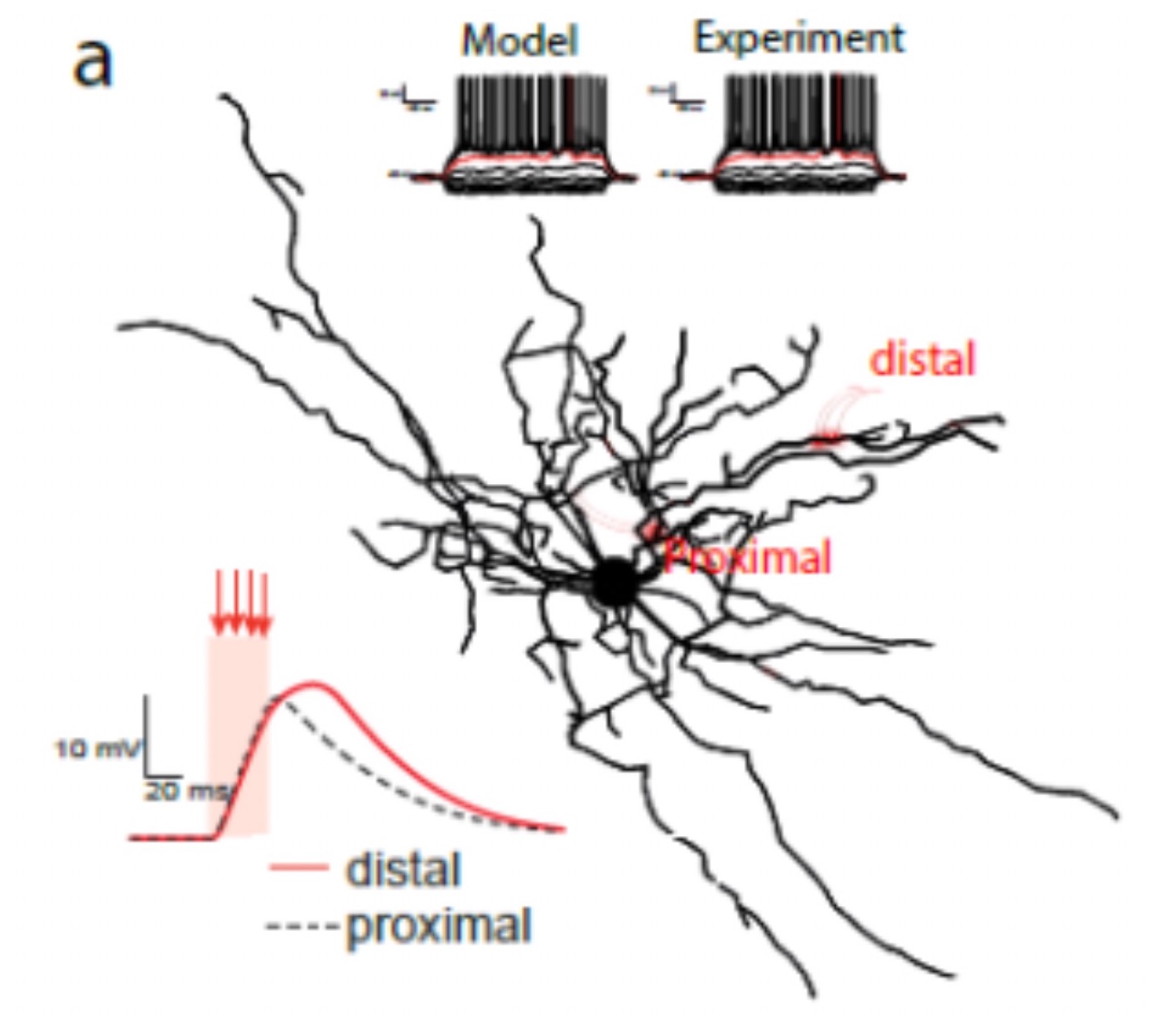
Data-driven brain modeling highlights the importance of the activation patterns of single inhibitory interneurons in the brain
Dendritic plateau potentials generated by the activation of clustered excitatory inputs play a crucial role in neuronal computation and are involved in sensory perception, learning, and memory.
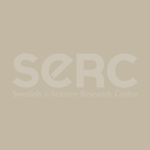
DeepClimate
In climate science, we will use datasets collected from existing external projects as well as public datasets to build prediction models that out-perform existing analytical and simulation models.
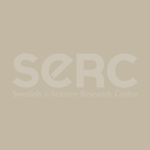
DeepProtein
In this project, we will develop deep learning methods using biological data. In particular, we will address the protein structure prediction problem, which involves predicting the structure from the amino acid sequence, predict interactions with other proteins and peptides, evaluating model qualities and predict amino acid contacts.
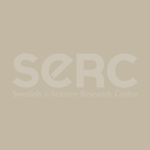
DeepTurbulence
In this project, we propose to develop and use generic Deep Learning techniques that are able to model physical (simulated and/or measured) dynamics.
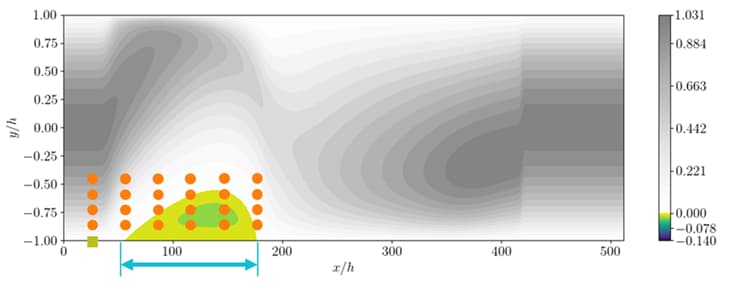
DeepTurbulence subproject: Using Machine Learning for controlling turbulent flow
Guastoni, Ghadirzadeh, Rabault, Schlatter, Azizpour, Vinuesa, “Deep Reinforcement Learning for Active Drag Reduction in Wall Turbulence”, American Physics Society, 2021.
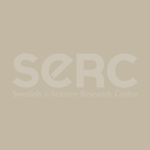
Designing the Next-Generation FFT library
FFT algorithms and software libraries are workforces of scientific computing and data analysis. Most large-scale scientific applications use and rely on FFT libraries, such as FFTW and FFTPACK, which were developed at the end of the Eighties.
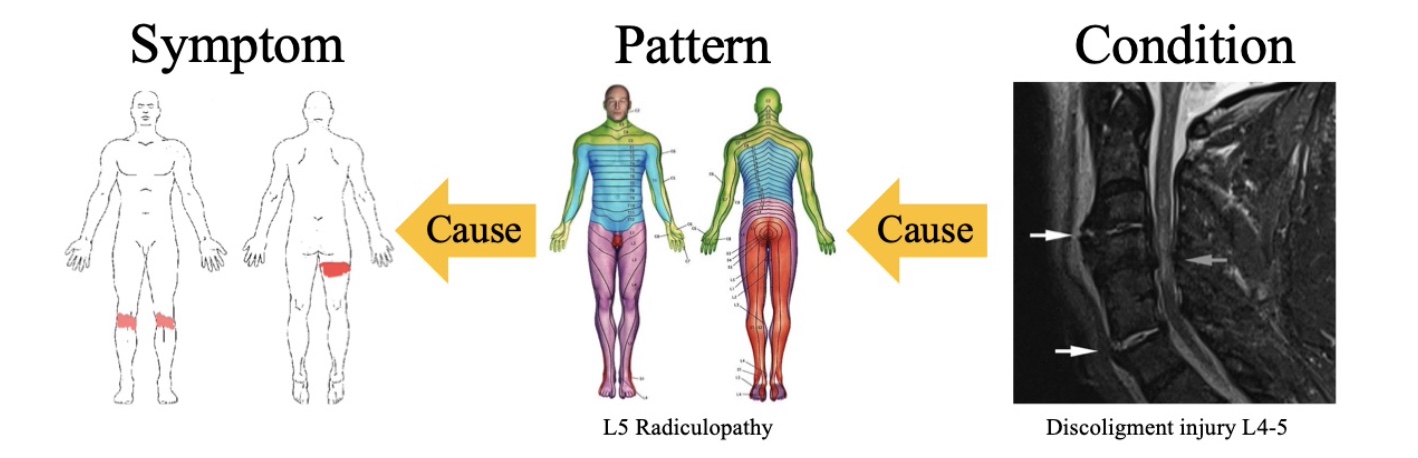
Developed methods for discovering cause and effect from medical data
Reasoning about cause and effect is an important aspect of human intelligence, and would thus constitute a valuable part of a medical diagnostics system.
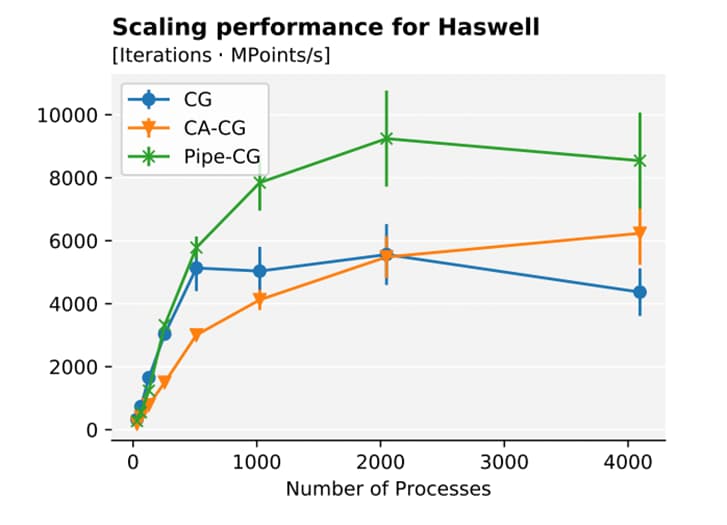
Developing Communication-Avoiding Schemes for Exascale Spectral Element Codes
We investigated a communication-bound solver at the core of many high-performance applications, namely the Conjugate Gradient (CG) method.
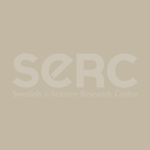
Development of a novel AI-based risk stratification model for intermediate risk breast cancer patients
This study is one of the first AI-based computational pathology studies that focus on precision diagnostics, i.e. extracting novel information that can be used to risk stratify patients beyond current clinical routine.
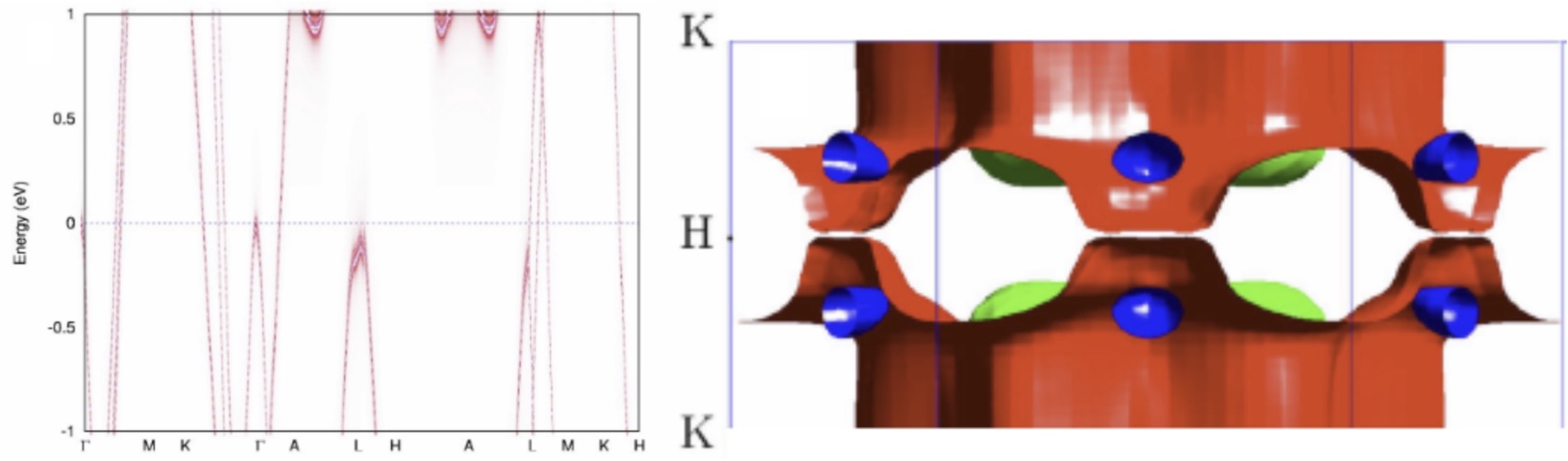
Development of novel modeling techniques
A need for high quality large volume materials data requires basic research into thedevelopment of novel modeling techniques. This work concerns method development with increased accuracy and efficiency, including dynamical mean-field theory (DMFT), spin- dynamics, time-dependent response theory (TDRSP), and molecular dynamics (MD).
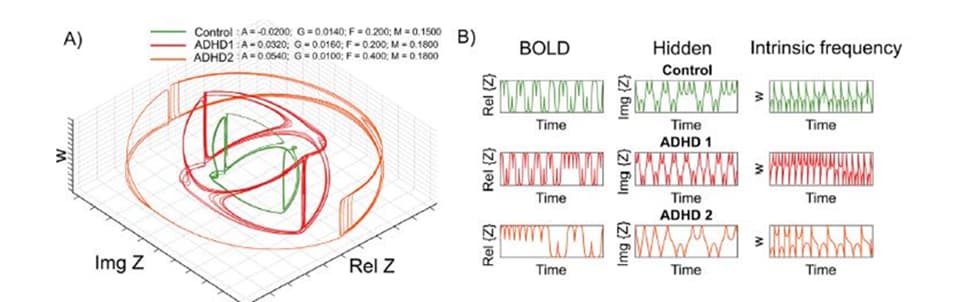
Differentiating ADHD subtypes and simulation of therapeutical interference
Figure legend: The oscillator model dynamics for the Control and ADHD subgroups.
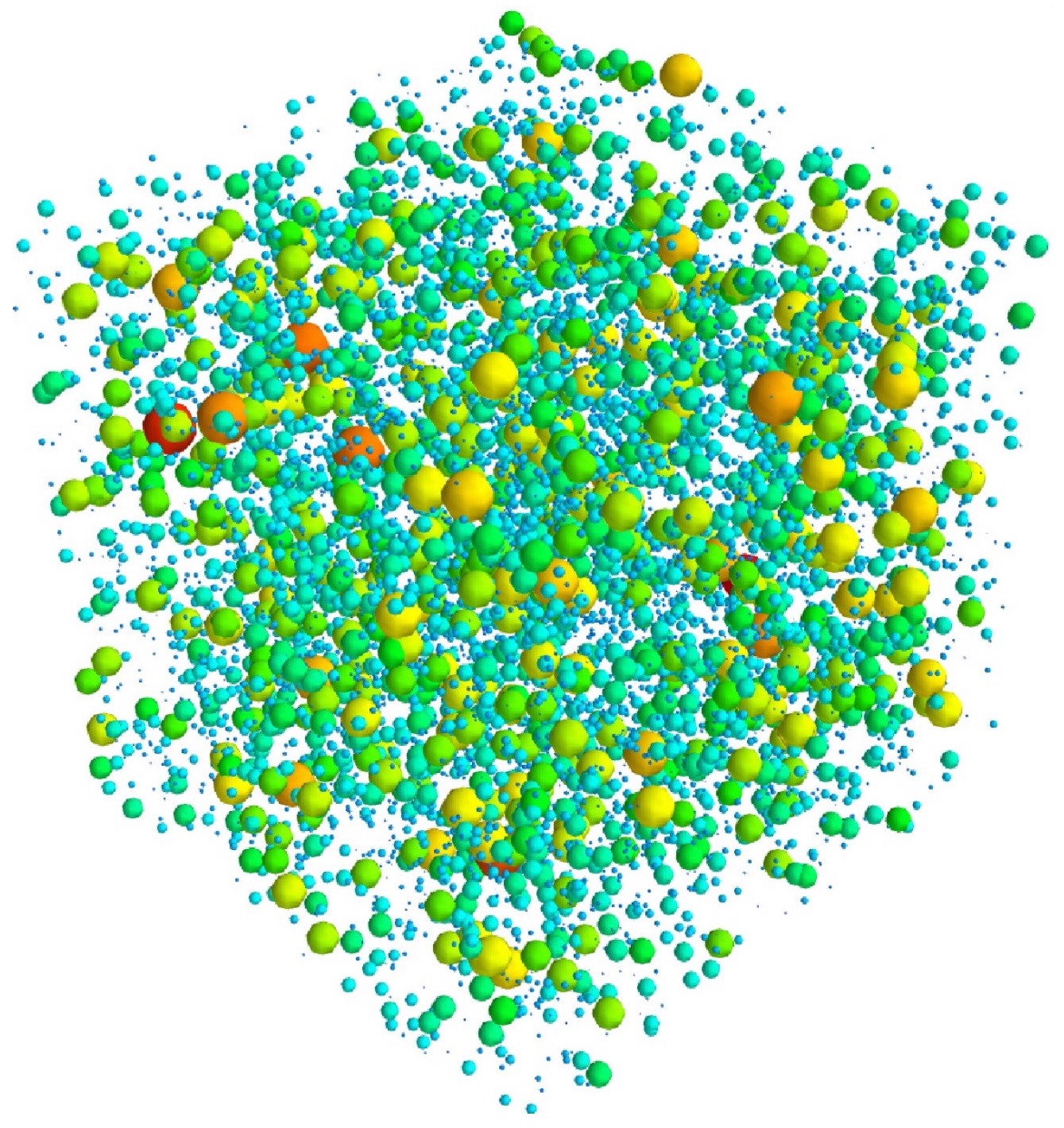
Direct Numerical Simulations of cloud droplet – turbulence interactions
The possible enhancement of droplet growth by turbulence is investigated. The first step in this project is to include droplet microphysics, condensation and collection processes, in a DNS code.
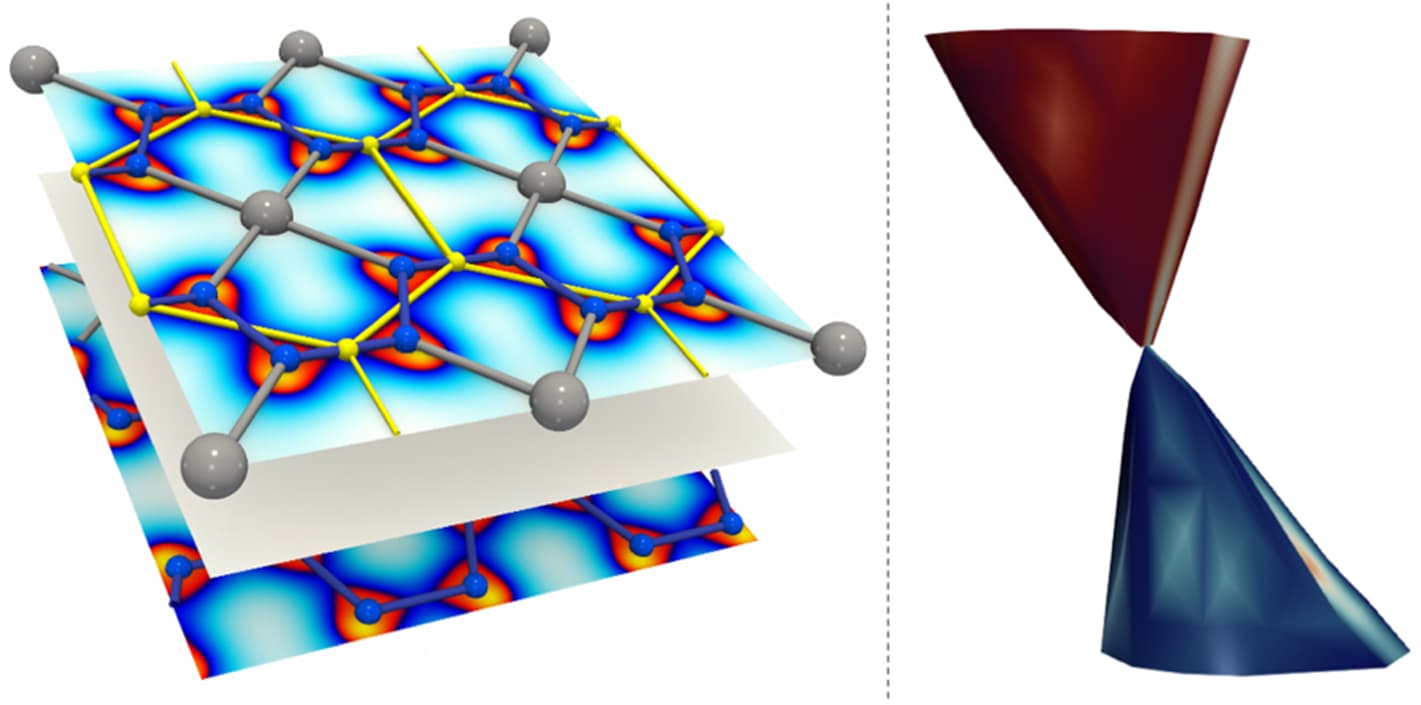
Discovery of a new Dirac material, layered van der Waals bonded BeN4 polymorph
In collaboration between theory, visualization and experiment we predicted and confirmed experimentally a possibility to synthesize new layered compound with atomic-thick BeN4 layers interconnected via weak van der Waals bonds consisting of polyacetylene-like nitrogen chains with conjugated π-systems and Be atoms in square-planar coordination.
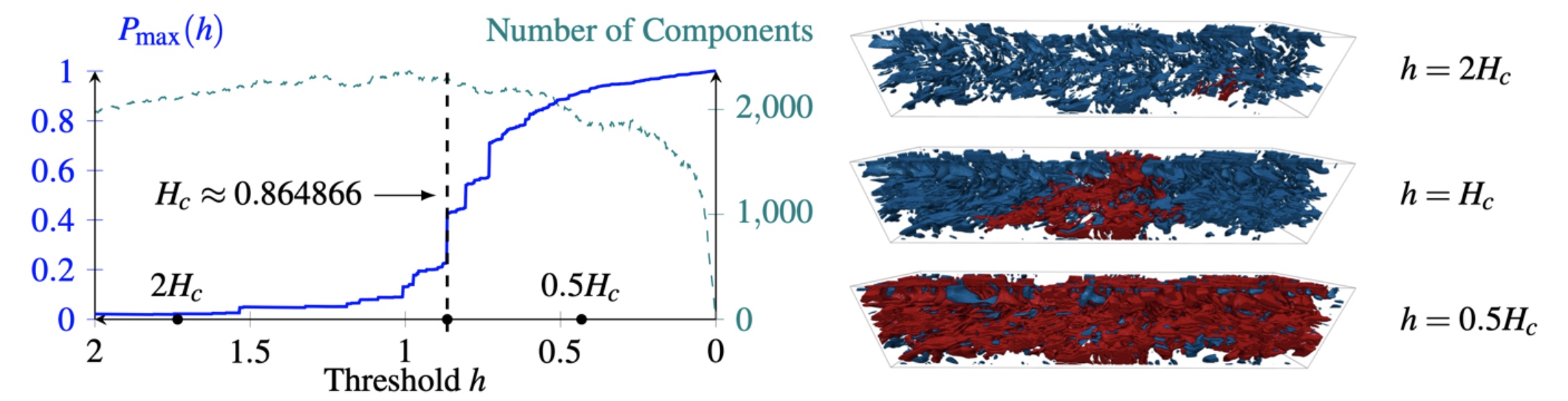
Distributed Percolation Analysis for Turbulent Flows
Turbulent flow analysis plays a crucial role in many domains e.g. design of fuel-efficient cars and is an active research area primarily addressed through direct numerical simulations (DNS) of the Navier-Stokes equations.
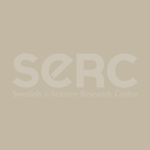
e-Spect: In silica spectroscopy of complex molecular systems
Theoretical simulations are essential for the microscopic understanding of spectroscopic data that enable the design of biomarkers and materials. Modeling these complex systems requires a combination of molecular dynamics (MD) and quantum mechanics/molecular mechanics (QM/MM) approaches.
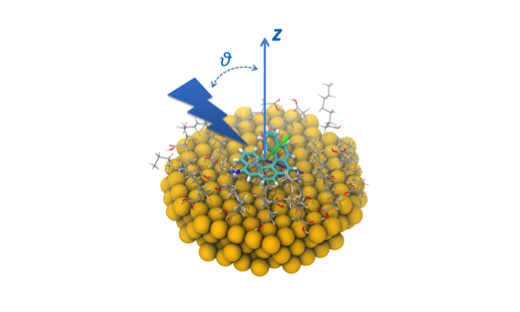
Electronic Highlight: Development of the Dalton program
Two powerful molecular electronic structure programs, Dalton and lsDalton, provide an extensive functionality for the calculation of molecular properties. …
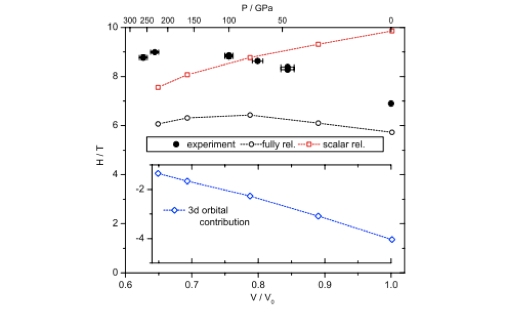
Electronic Highlight: Hyperfine Splitting and Room-Temperature Ferromagnetism of Ni at Multimegabar Pressure
Observed magnetic hyperfine splitting confirms the ferromagnetic state of Ni up to 260 GPa, the highest pressure where magnetism in any material has been observed so far. …
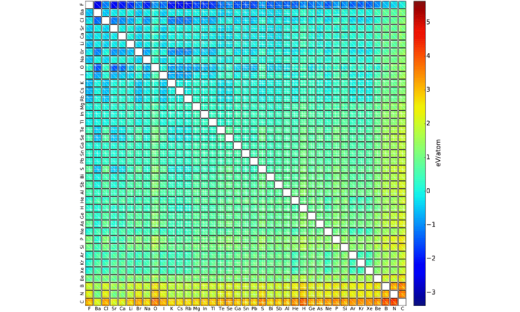
Electronic Highlight: Machine Learning Energies of 2 Million Elpasolite (ABC2D6) Crystals
Elpasolite is a common crystal structure. We have developed and trained a machine learning model to predict formation energies of all 2M pristine ABC2D6 elpasolite crystals that can be made up from main-group elements (up to bismuth). …
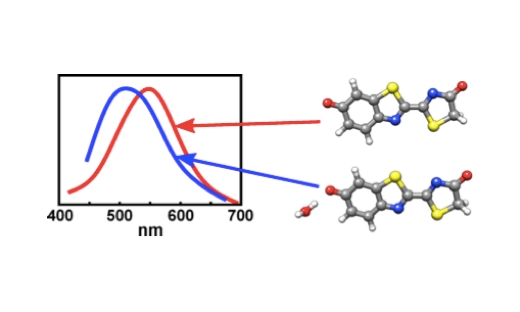
Electronic Highlight: On the Influence of Water on the Electronic Structure of Firefly Oxyluciferin Anions
Combining molecular dynamics simulations and time-dependent density functional theory calculations indicate that the preferred binding site for the water molecule is the phenolate oxygen of the anion. …
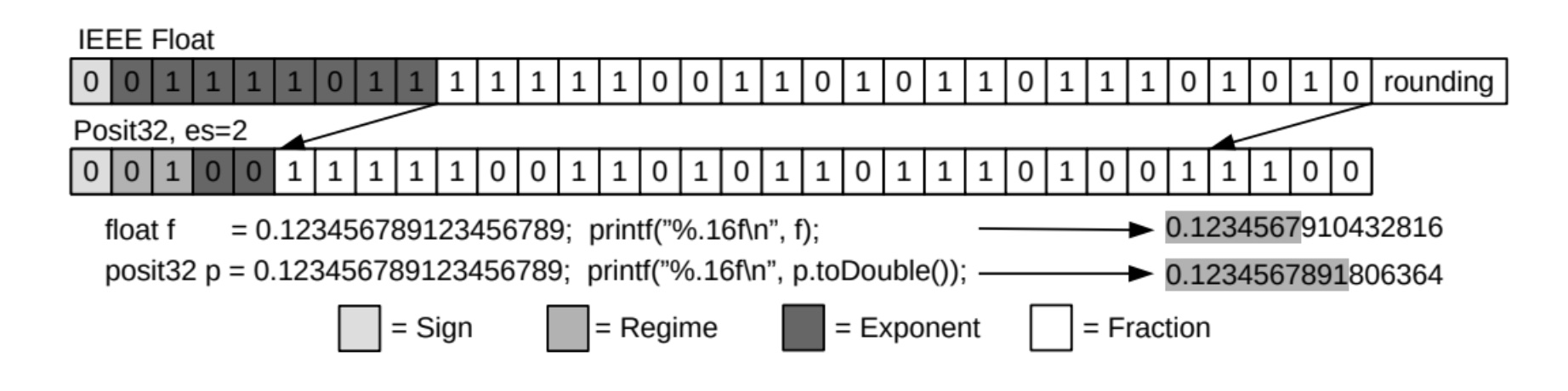
Emerging Floating-Point Formats for HPC
Floating-point operations are indispensable for many scientific applications. Their precision formats can significantly impact the power, energy consumption, memory footprint, performance, and accuracy
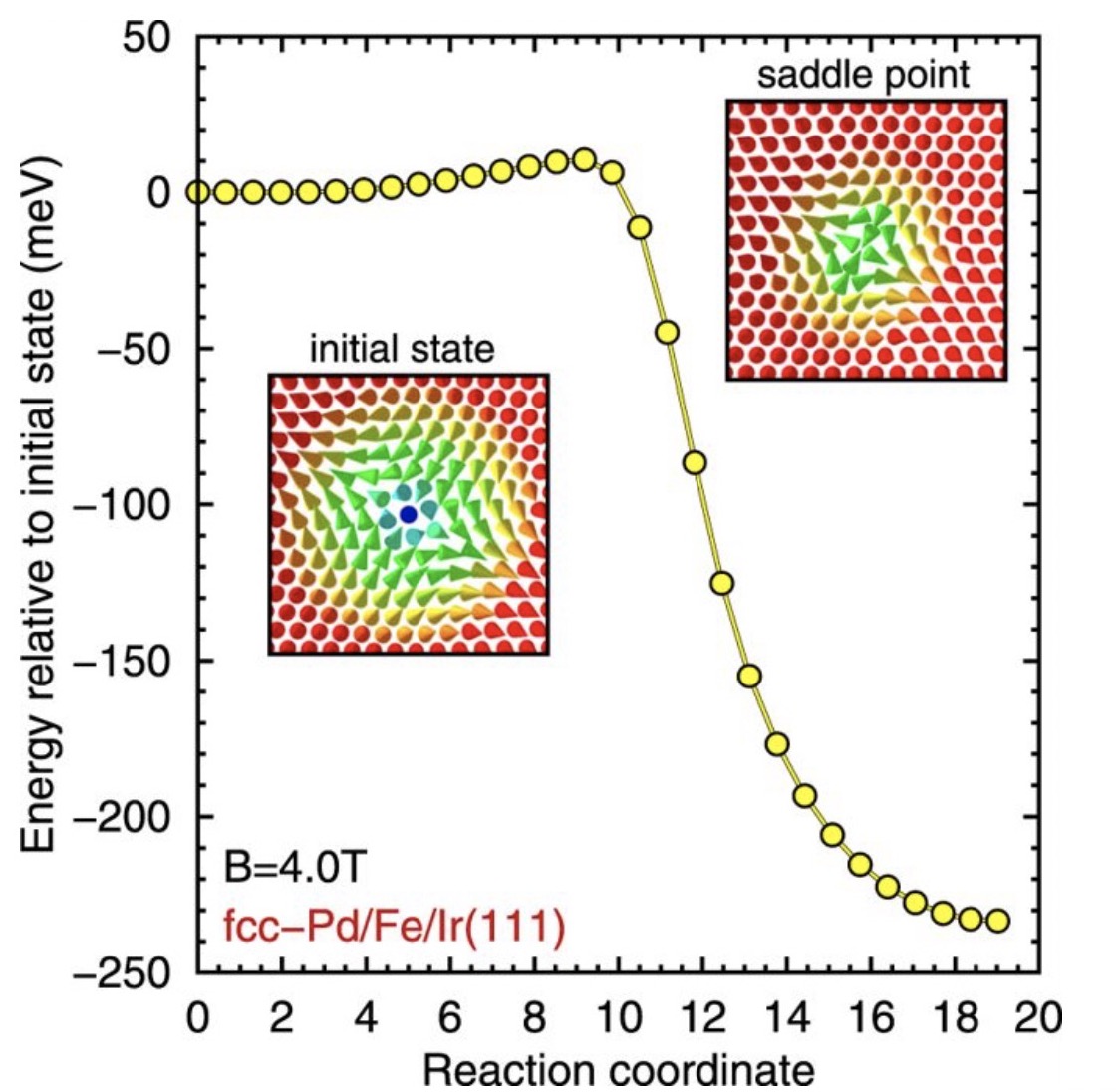
Enhanced skyrmion stability due to exchange frustration
We show that energy barriers and critical fields of skyrmion collapse as well as skyrmion lifetimes are drastically enhanced due to frustrated exchange and that antiskyrmions are metastable.
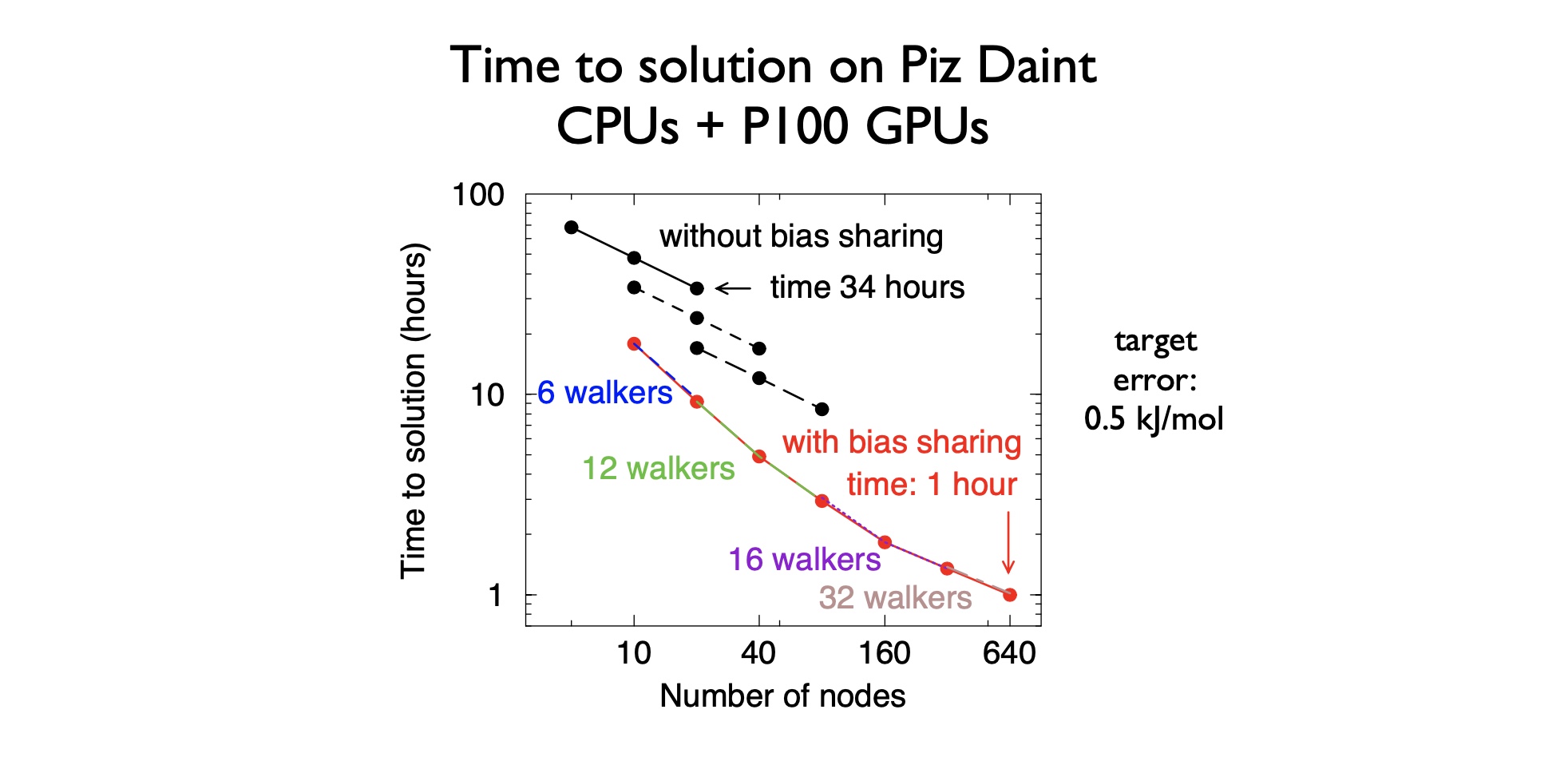
Ensemble parallelism in GROMACS
Scaling individual atomistic simulations of bio-molecules is strong scaling problem, as the molecules have fixed size.
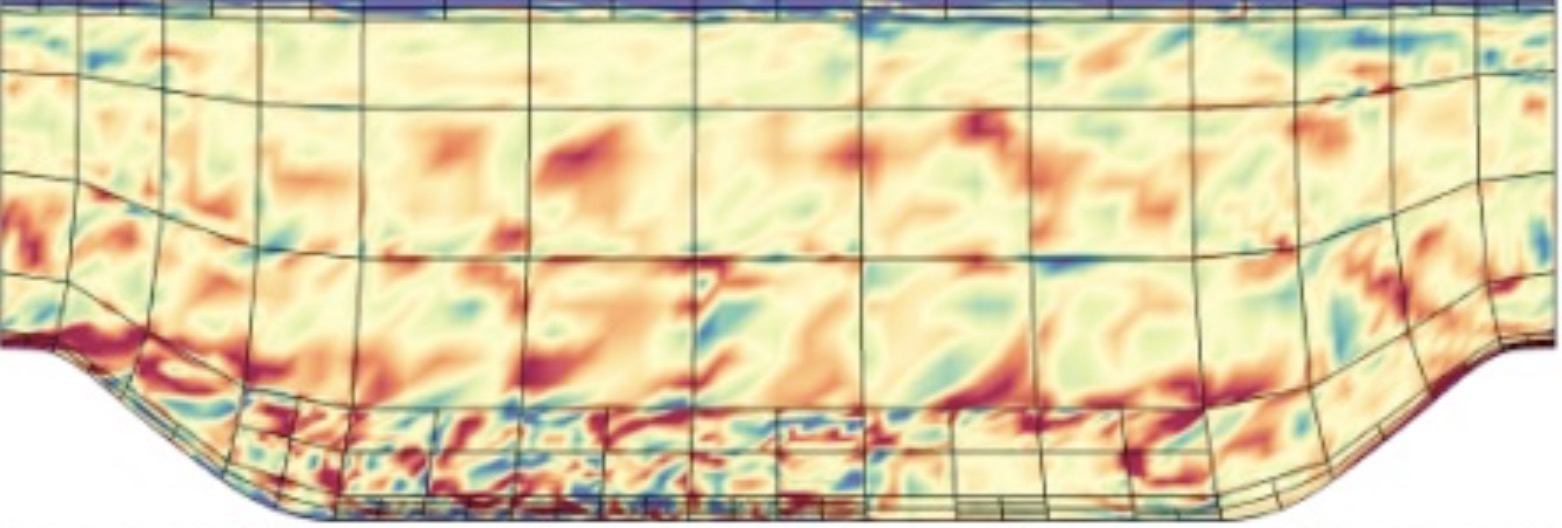
Error estimators and adaptive mesh refinement for Nek5000 (with SESSI)
Our continuing work on extending the open-source code Nek5000, based on the high-order spectral element method, has now reached a level of maturity such that we can – for the first time – perform simulations of turbulence in complex geometries.
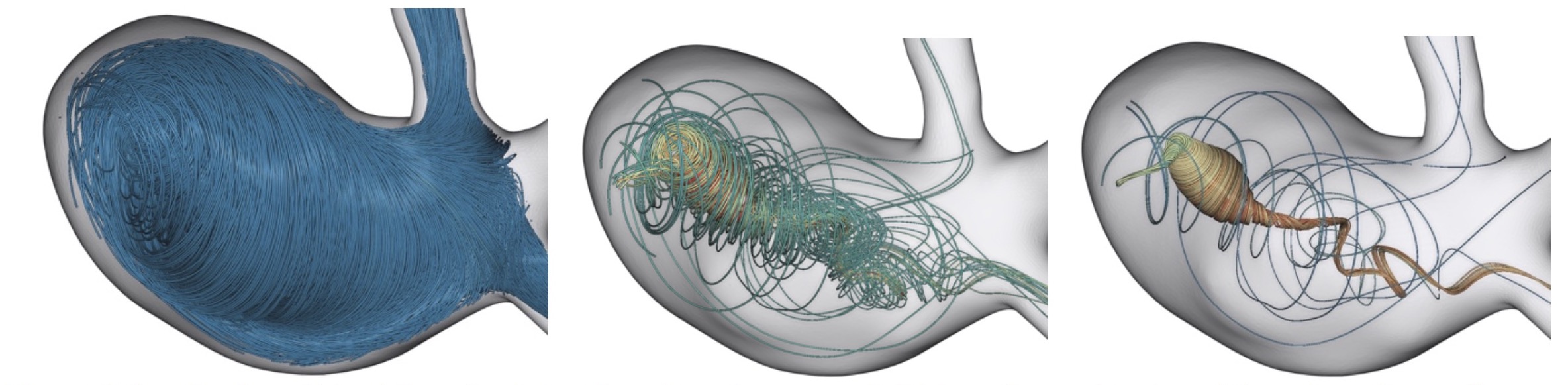
Evolutionary streamlines for automatic flow feature extraction
In this work we explore the potential of evolutionary algorithms to generate expressive visualizations. Evolutionary algorithms find close-to-optimal solutions for tasks by imitating biologically mechanisms like selection, mutation and recombination.
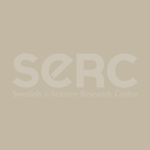
ExABL
A better understanding of the processes in atmospheric boundary layers (ABL) and efficient simulations are required e.g. to improve climate predictions, and simulations of wind parks.
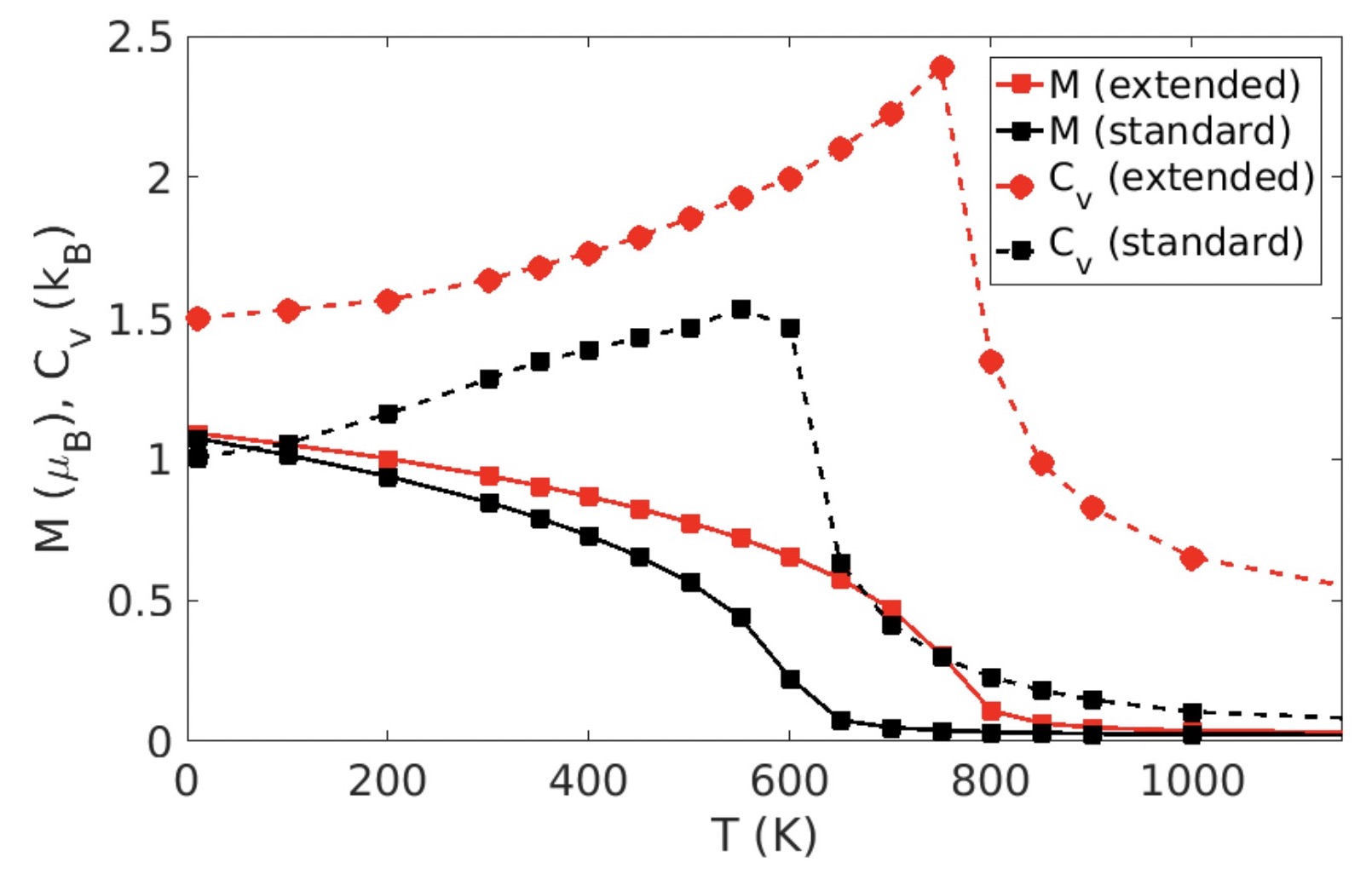
Extended spin model in atomistic simulations of alloys
The proposed model strives to find the right compromise between accuracy and computational feasibility for accurate modeling, even for complex magnetic alloys and compounds.
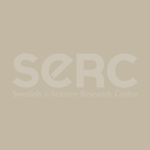
Feature based exploration of large-scale turbulent flow simulations
This project will enable in-situ detection and tracking of flow structures, which will be used as focus regions to build a multi-resolution description of the data. Interactive visualization methods from volume and flow visualization will be adapted to the new multi- resolution scheme.
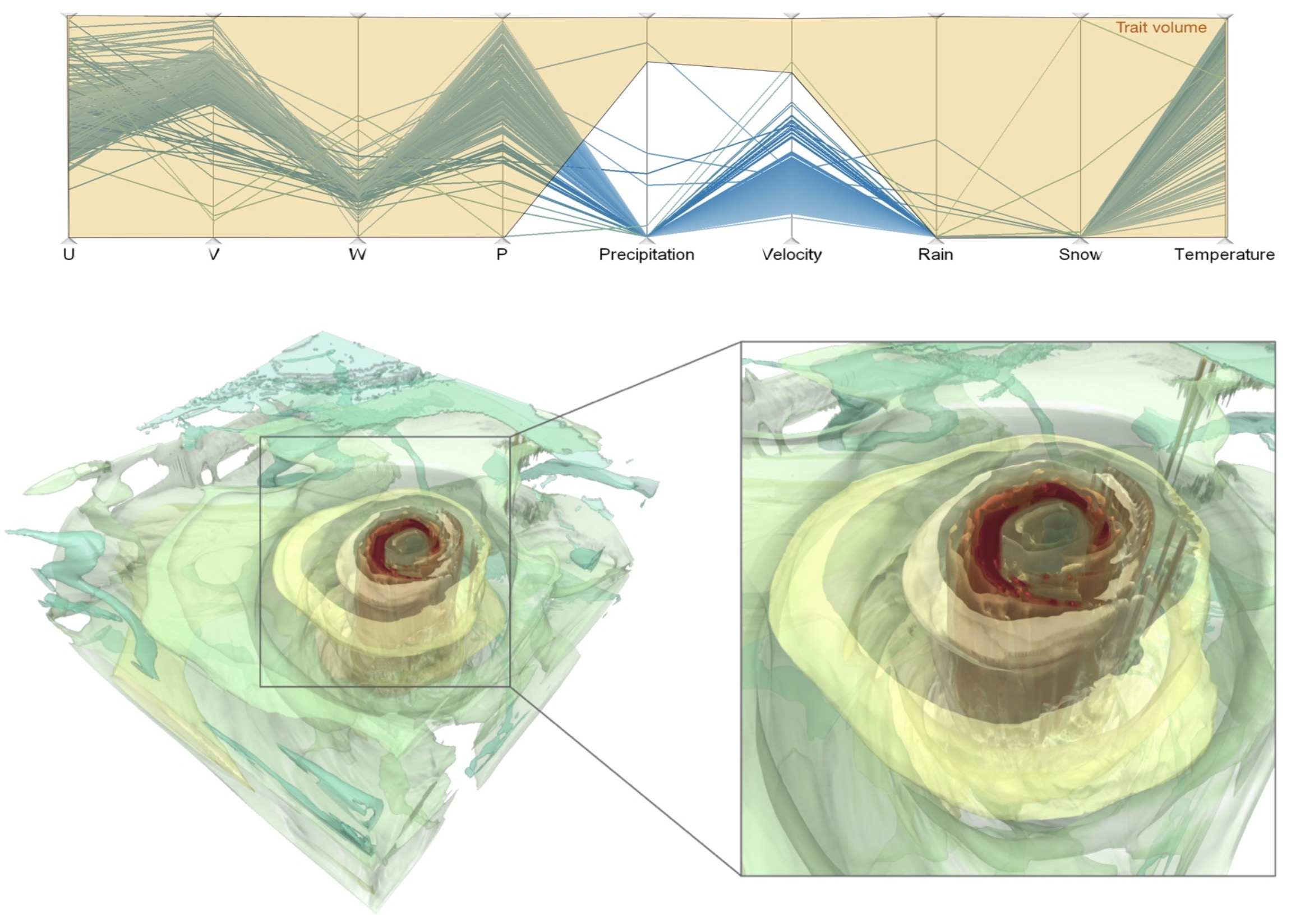
Feature level sets for multi-field visualization
Iso-surfaces or level-sets provide an effective and frequently used means for feature visualization. However, they are restricted to simple features for uni-variate data.
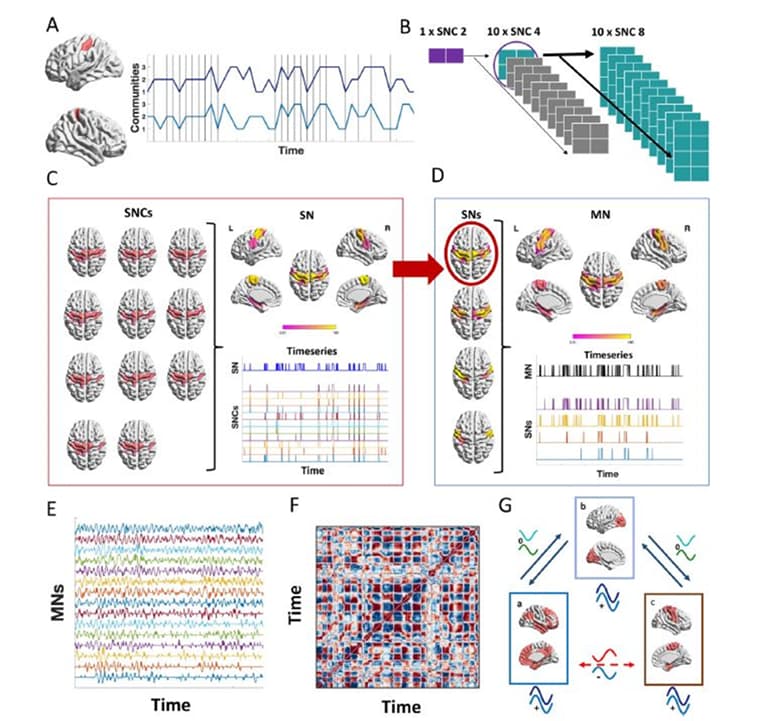
Flexible subnetworks reveal the quasi-cyclic nature of integration and segregation in the human brain
Figure Legend: Schematic figure that summarizes the key steps for the proposed model. A) The calculation of the parameter q int is illustrated for the case of a pair of brain areas in the left and right motor cortex.
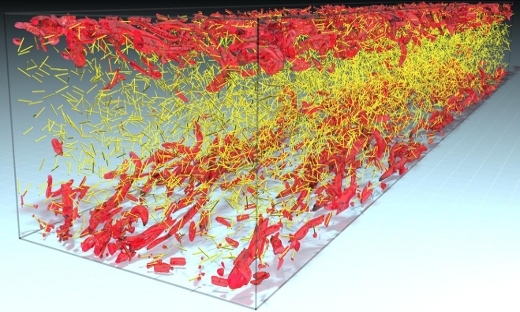
FLOW Highlight: Fully resolved simulations of fibers with a significant size in turbulent flows
The interaction between fibers and carrier fluid is modeled through an external boundary force method. …
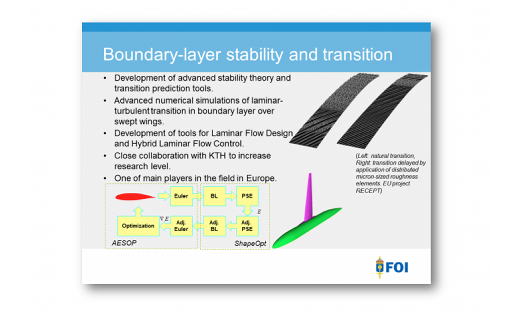
FLOW Highlight: Natural Laminar Flow
Direct numerical simulations of flow over wings for understanding the physics of modern methods of transition control. …
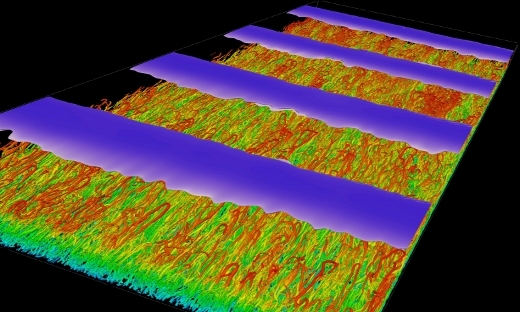
FLOW Highlight: Recurring intense bursts of turbulence in rotating channel flow simulations
Carrying out extensive simulations and observing for the first time intense recurrent bursts of turbulence in rotating channel flow. Rotating channel flow is therefore suggested as a prototype for future studies. …
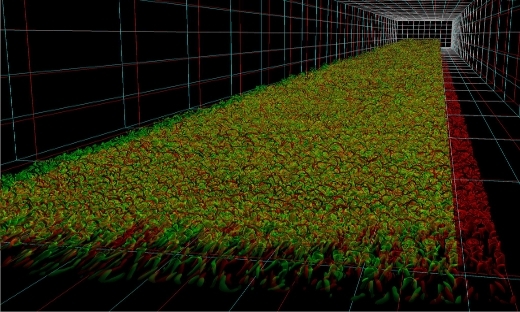
FLOW Highlight: Simulations of turbulent boundary layers at high Reynolds numbers
The study of simplified canonical flows allows for deducing important properties of the physics. Therefore, a number of canonical flow cases have emerged as standard model problems to study wall-bounded turbulence. …
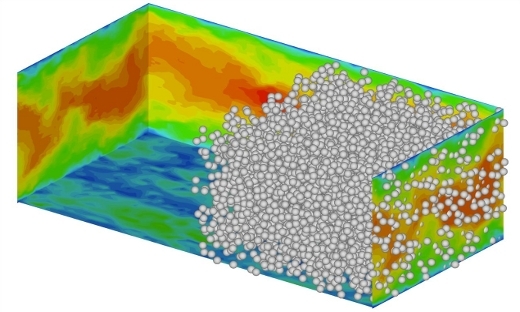
FLOW Highlight: TRITOS: Transitions and Turbulence Of complex Suspensions
Investigating the mechanisms by which the system microstructure determines the macroscopic flow properties provides valuable insight into the nature of flowing suspensions, and also leads to new ways of modelling and controlling it.
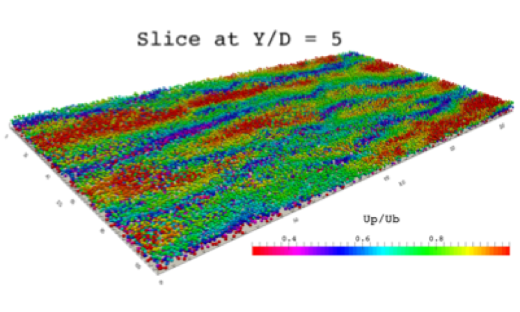
FLOW Highlight: Universal Scaling Laws or Dense Particle Suspensions in Turbulent Wall-Bounded Flows
We examine by means of large-scale interface-resolved numerical simulations the macroscopic behavior of dense suspensions of neutrally-buoyant spheres in turbulent plane channel flow. …
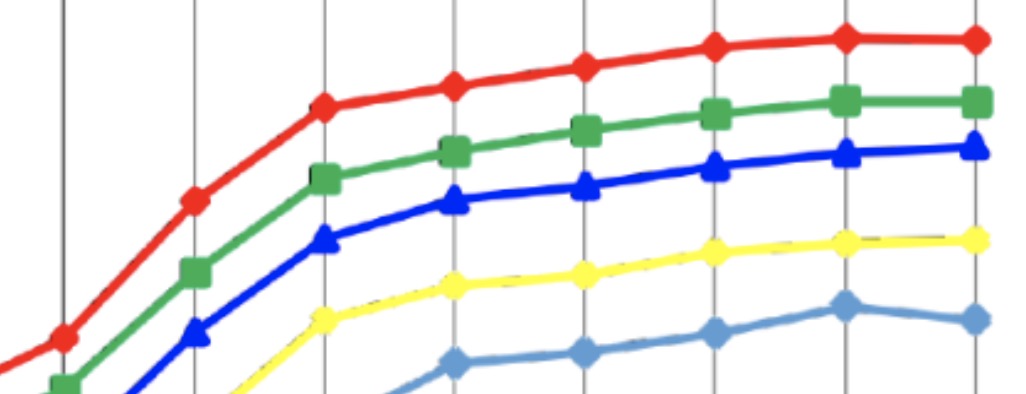
GPU acceleration in Nek5000 (with SESSI)
Due to its high performance and throughput capabilities, GPU-accelerated computing is becoming a popular technology in scientific computing, in particular using programming models such as CUDA and OpenACC.
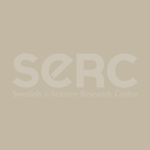
GROMACS
Molecular simulation has evolved into a standard technique employed in virtually all high-impact publications e.g. on new protein structures. The main bottleneck for scaling in GROMACS is the 3D-FFT used in the particle-mesh Ewald electrostatics (PME). Since PME is very fast, and used by MD codes world wide, it is worth investigating if the communication overhead can be lowered. This is done in collaboration with PDSE (see the 3D-FFT sub-project), as well as a co-design effort with Nvidia for parallelizing the 3D-FFT over GPUs. For extreme scaling, we will also investigate the fast multipole method (FMM) since it has better scaling complexity. A problem was always energy conservation, which is now solved in collaboration with the numerical analysis community, and we will integrate the ExaFMM code of Rio Yokota (Tokyo Tech) into GROMACS.
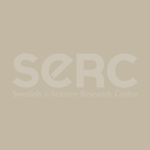
Health economic evaluations of prostate cancer testing in Sweden
We provided important evidence to policy makers on prostate cancer screening and testing.
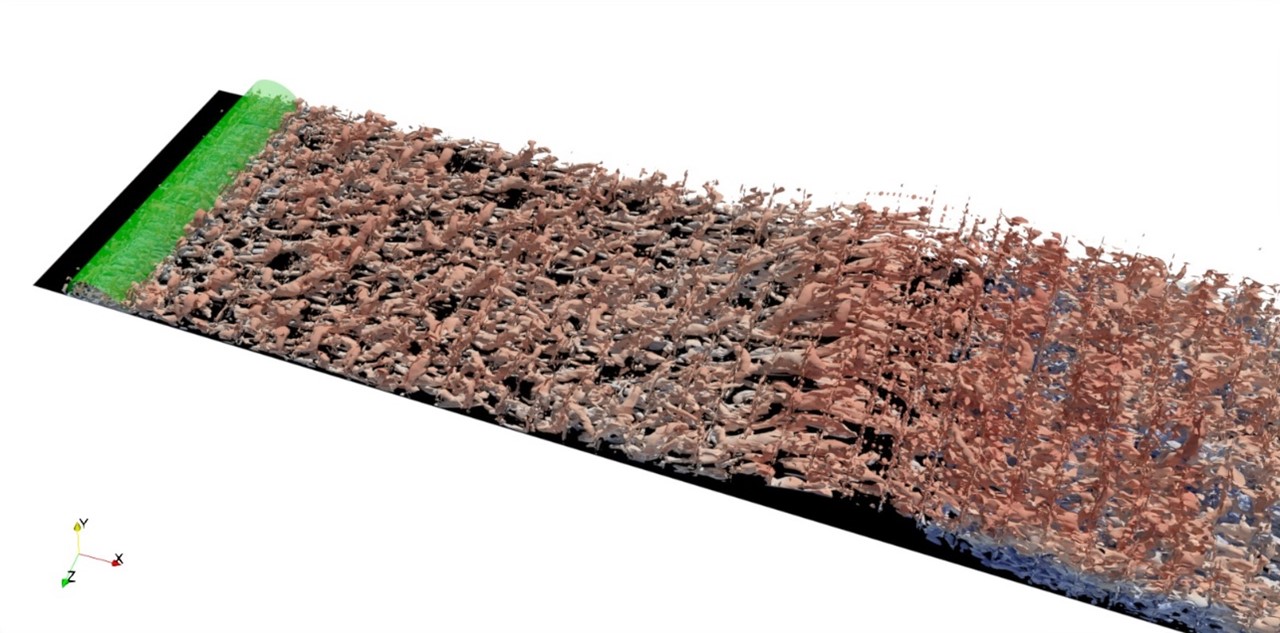
High-fidelity simulations on massively parallel architectures for aeronautical applications
Simulations of flow over a smooth bump are being conducted by R. Stanley under the supervision of Drs. Mukha, Schlatter, and Markidis. Currently, numerical tripping is used to trigger transition of the incoming boundary layer to turbulence.
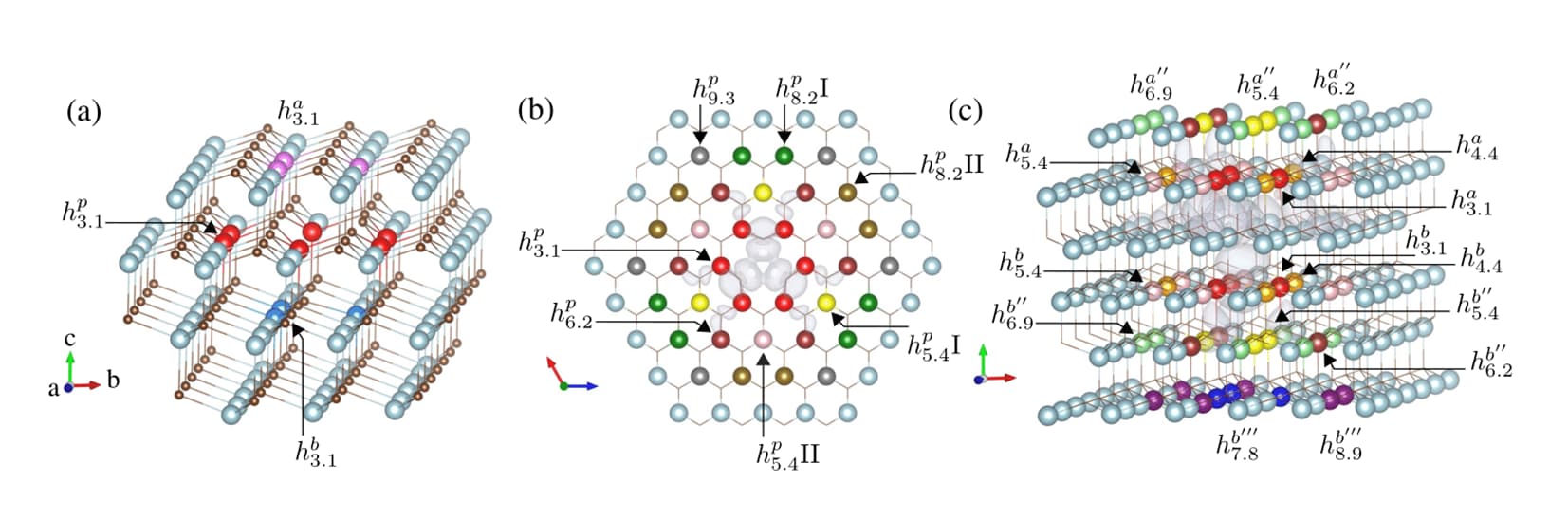
High-throughput search of point defects in SiC
Point defects in semiconductors is an active field in quantum applications. These defects are used as sensors and qubits.
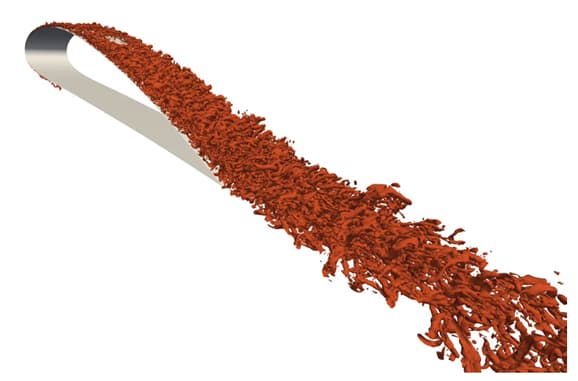
In situ visualization of large‐scale turbulence simulations in Nek5000
In situ visualization on high-performance computing systems allows us to analyze simulation results that would otherwise be impossible, given the size of the simulation data sets and offline post-processing execution time.
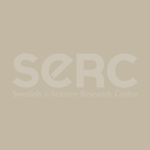
Integrated analysis of cardiac function
Recent developments within image-based simulation and imaging of cardiac function can potentially advance diagnosis and treatment optimization of a range of cardiac diseases.
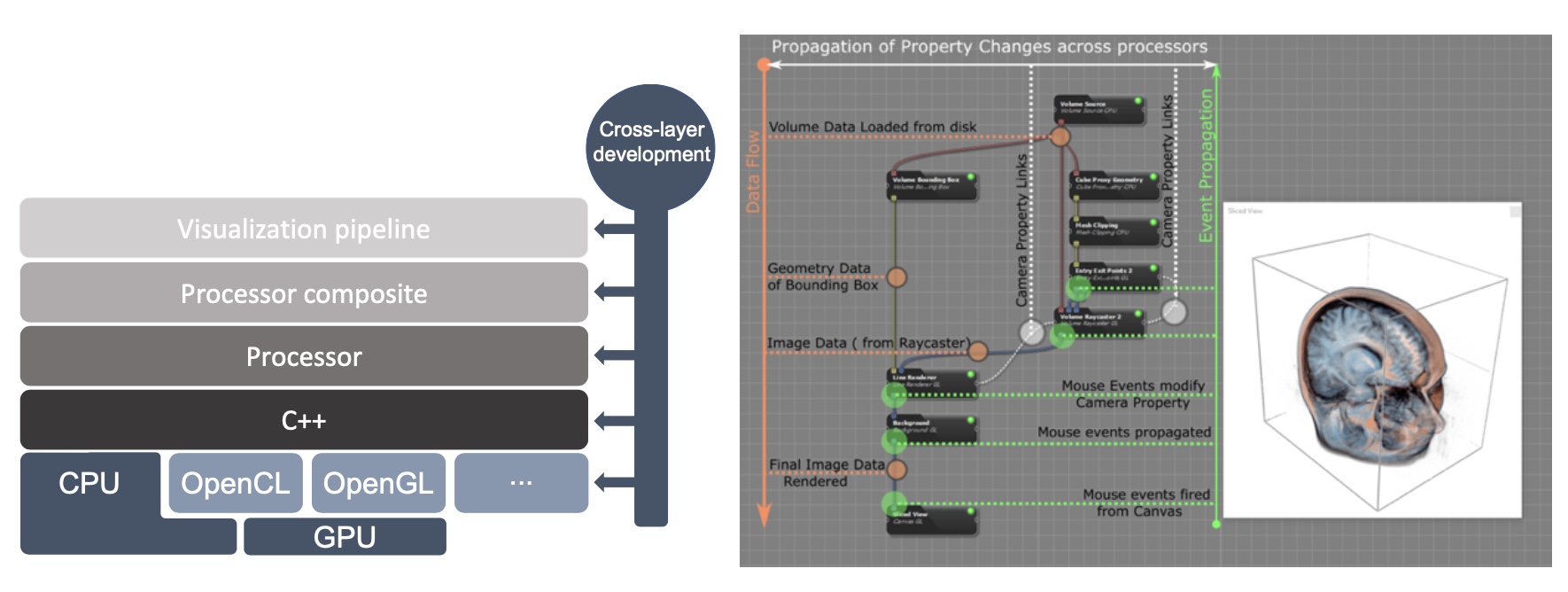
Inviwo – An open-source Visualization System with Usage Abstraction Levels
Inviwo is a software framework for rapid prototyping visualizations. It builds the basis for the development of novel visualization research and teaching
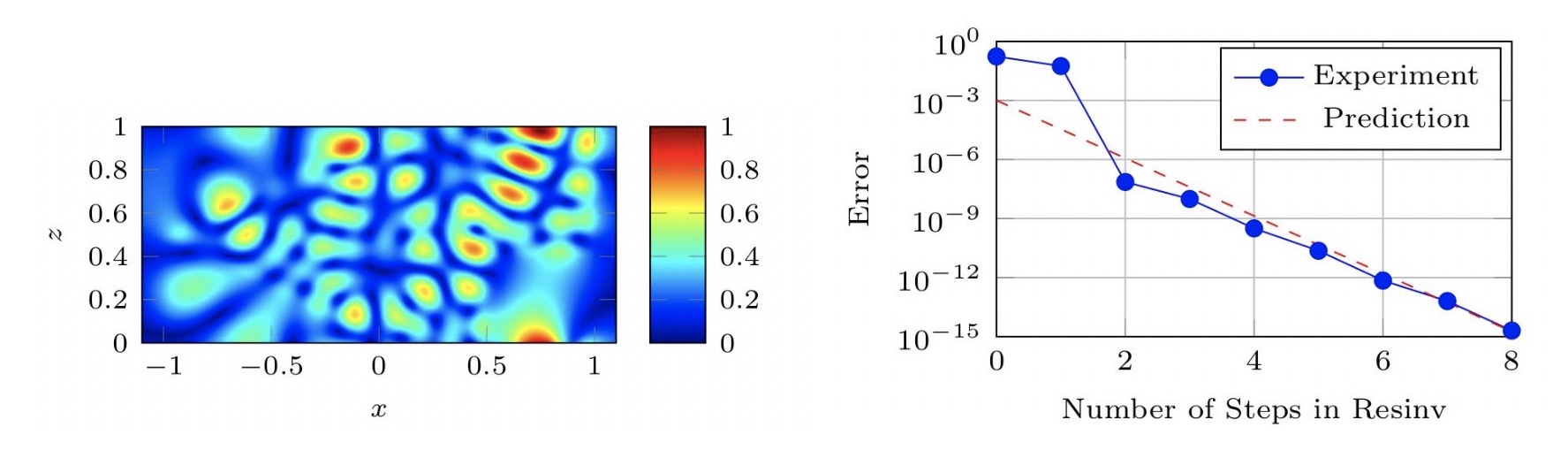
Iterative eigenvalue algorithms in unbounded domains
In this project, we take an approach where the domain is treated as an infinite domain, leading to a nonlinear eigenvalue problems. New approaches are developed from a numerical linear algebra perspective, e.g. using Arnoldi’s method and modern iterative methods for linear systems, as well as a perspective of numerical methods for partial differential equations.
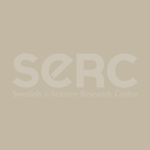
KARISMA trial results: chemoprevention for breast cancer
We completed the KARISMA trial (PI Per Hall) and show for the first time in a 6-armed controlled randomized trial (n=1440) that low-dose Tamoxifen induces noninferior magnitude of breast density decrease at a 2.5 mg dose, but fewer side effects compared with the standard dose of 20 mg [4].
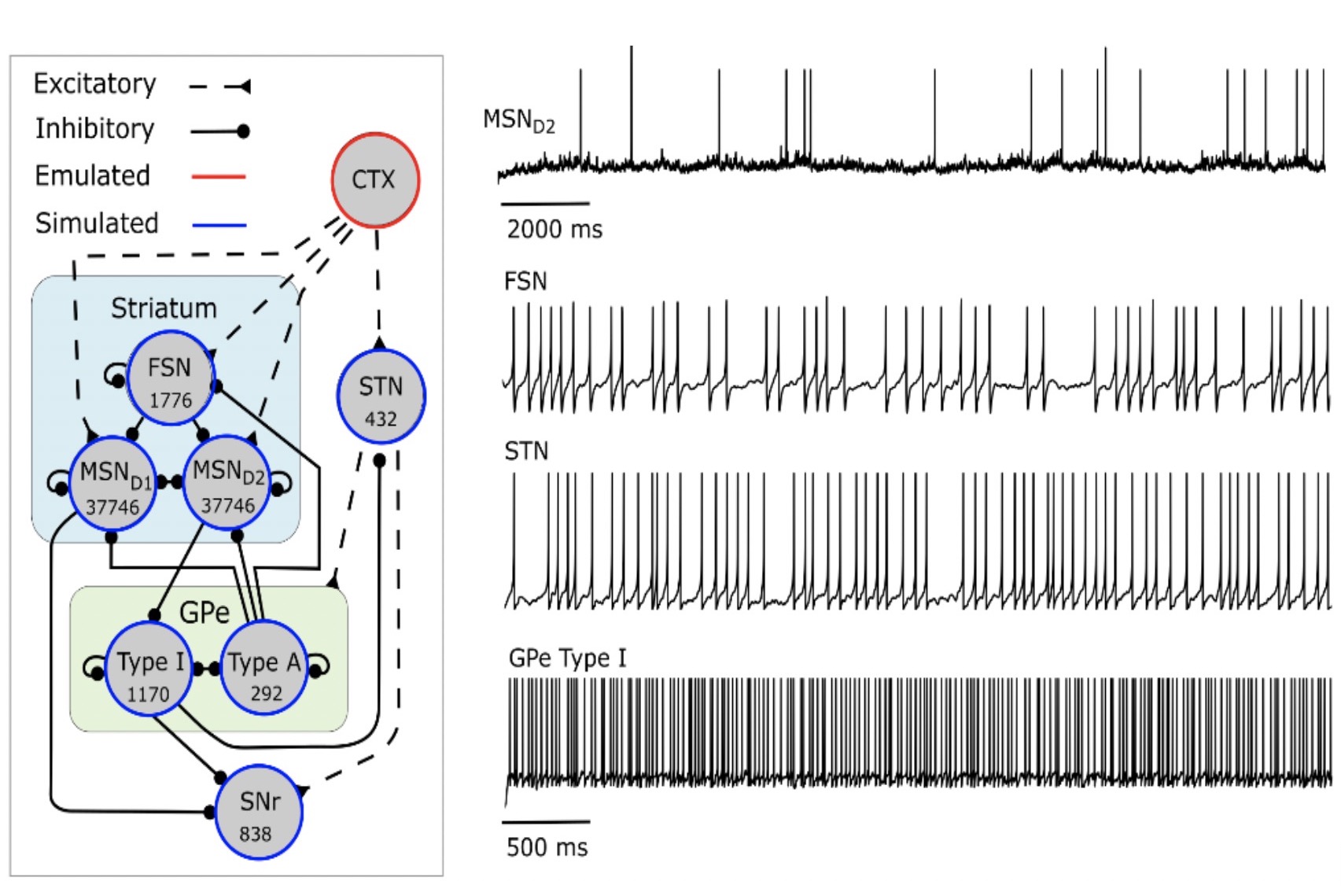
Large scale neural network models of the basal ganglia – an in silico tool for understanding the healthy and diseased system
We built a BG network model consisting of 80 000 spiking neurons (Lindahl and Hellgren Kotaleski, 2017) and used it to better understand how network parameters contribute to function as well as network dynamics, and how functionality can be recovered in the disease state.
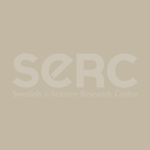
Large-scale ice sheet modeling
We focus on improving the efficiency of ice sheet models coupled to the ocean. Constructing accurate models of the ice sheets on Greenland and Antarctica is crucial in order to reliably estimate sea level rise. Due to low efficiency of todays models it is currently not possible to run accurate enough simulations for long enough time spans.
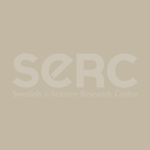
LearningSystems
Within the scope of the DataScience MCP, we aim to work on a radical software platform architecture able to close the gap between recording data and acting on it.
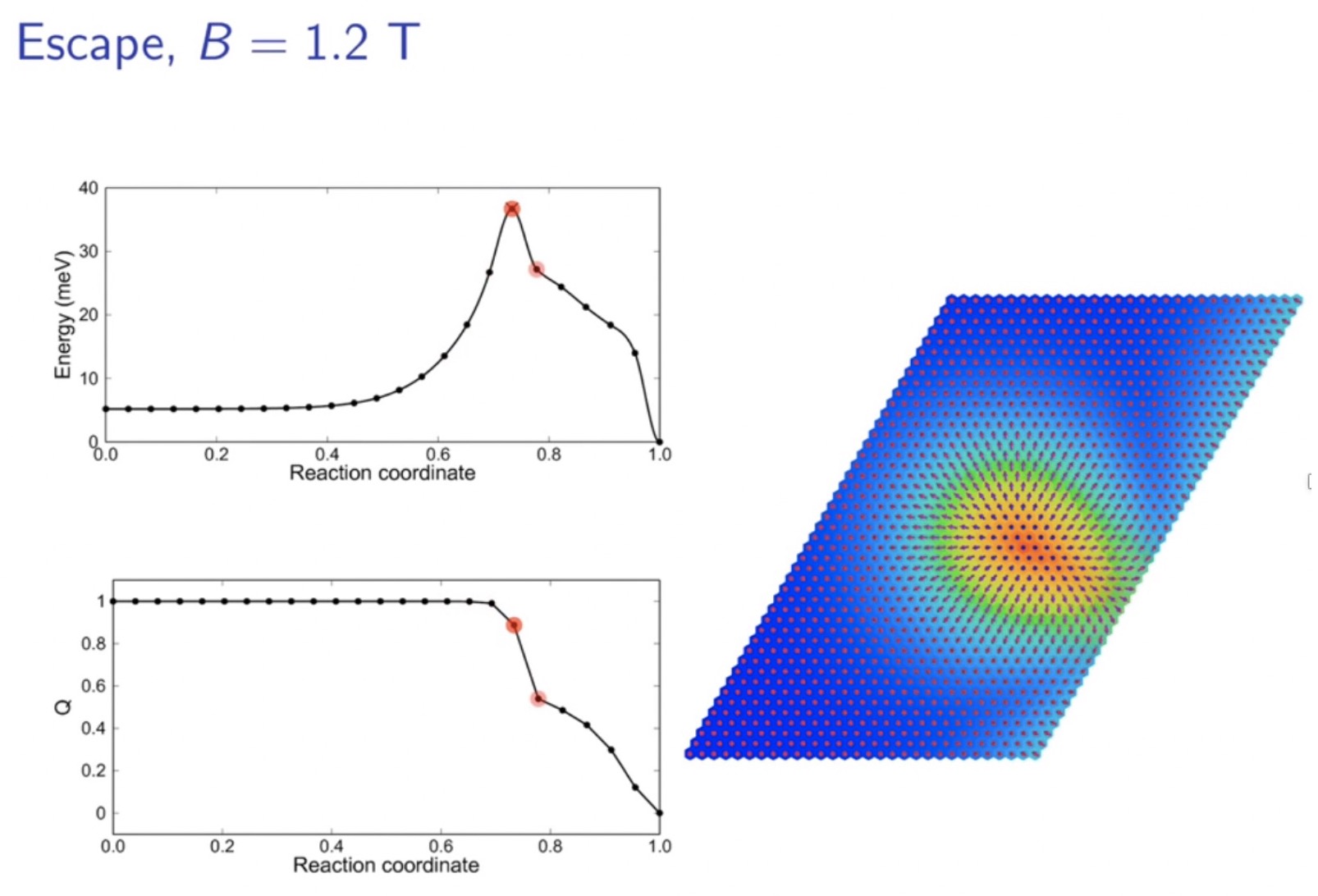
Lifetime of racetrack skyrmions
The skyrmion racetrack is a promising concept for future information technology. There, binary bits are carried by nanoscale spin swirls-skyrmions-driven along magnetic strips. Stability of the skyrmions is a critical issue for realising this technology. Here we demonstrate that the racetrack skyrmion lifetime can be calculated from first principles as a function of temperature, magnetic field and track width.
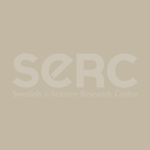
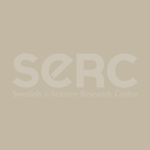
Medical image analysis and deep learning, with applications to prostate biopsies and mammograms
The ability to digitise large quantities of medical images together with recent progress in the area of deep learning and stochastic modelling of highly structured systems offers an opportunity to change and improve diagnostic procedures for screening.
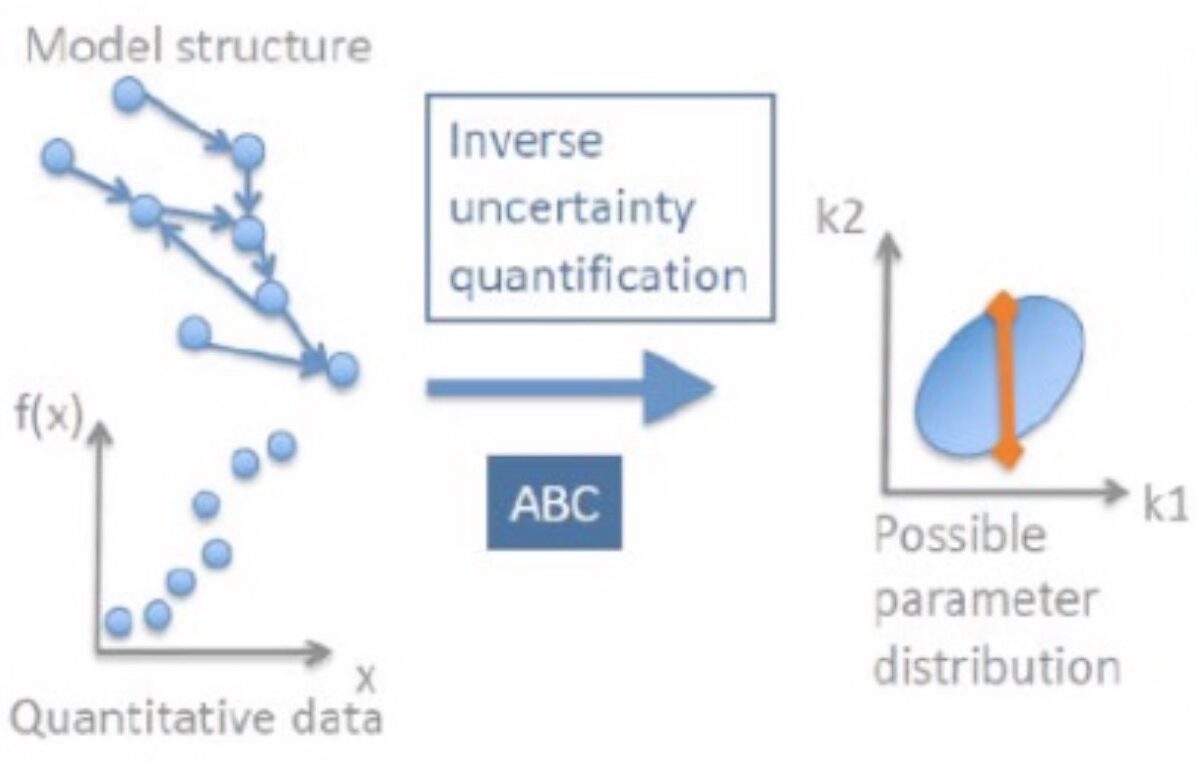
Model building workflow for subcellular models
A model building workflow supporting the estimation of subcellular model parameters using experimental data traces has been published.
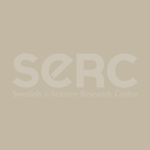
Modern approaches to prostate cancer screening: trial results from the STHLM3-MRI and STHLM3-AS studies
We finished the STHLM3-MRI trial and wrote the first two publications from the trial, published in the New England Journal of Medicine (impact factor 91) and the Lancet Oncology (impact factor 54) (Eklund et al 2021, Nordström et al 2021).
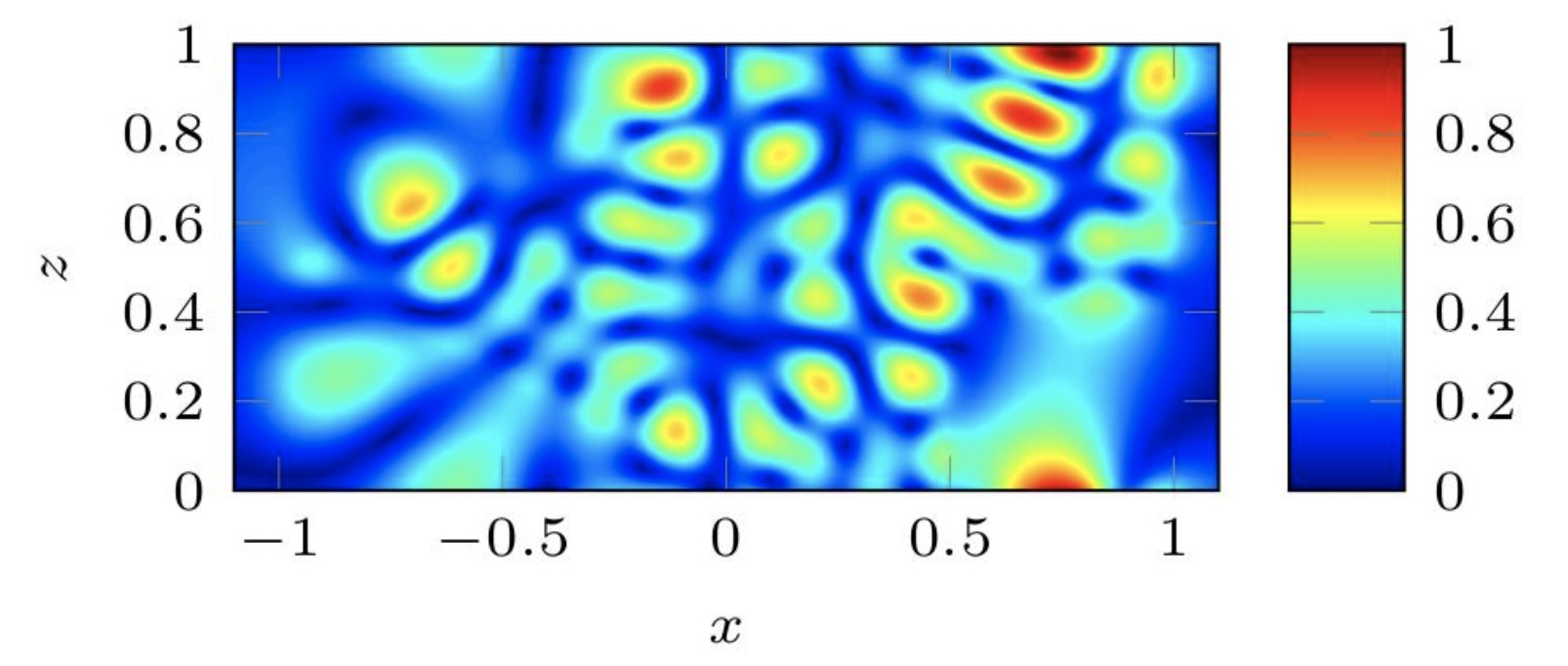
Modern iterative algorithms for waveguides
Waveguides are used in a number of fields, e.g., electromagnetics and fluid mechanics. These require the study of physical domains are typically very large leading to a high computational cost for many direct discretization methods.
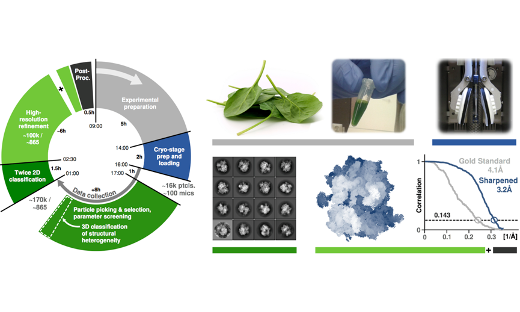
Molecular Highlight: Accelerating cryo electron microscopy refinement
Over the past years cryo electron microscopy has become a dominant technique for determining the structure of large biomolecules. …
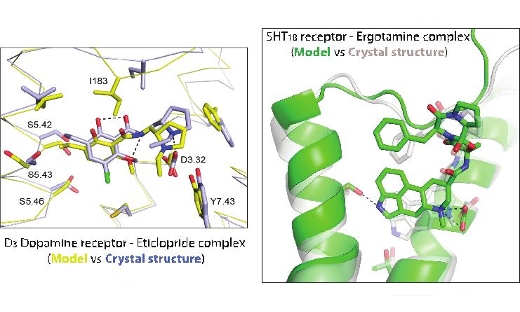
Molecular Highlight: Accurate predictions of GPCR-drug complexes
SeRC researchers have developed methods that enable accurate prediction of receptor-drug interactions at the atomic level. …
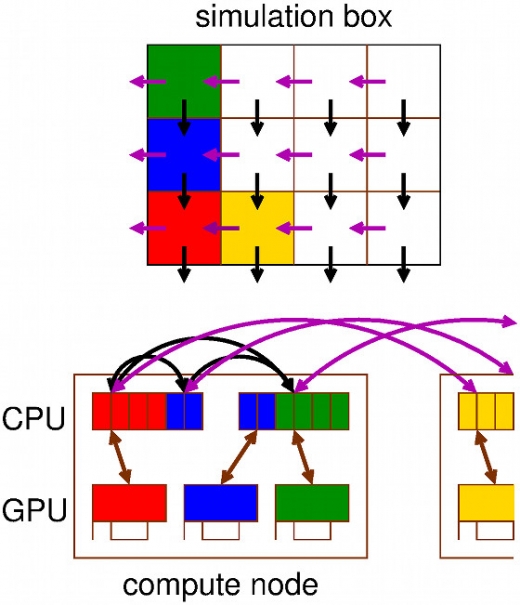
Molecular Highlight: The world’s fastest software for Molecular Dynamics on CPUs & GPUs
The increase in computing power allows part of (bio) molecular experimental work to be replaced by simulations. However, designing software that leverages the full power of modern (super) computing is becoming more and more challenging due to the fact that hardware is continuously becoming more parallel and heterogeneous. …
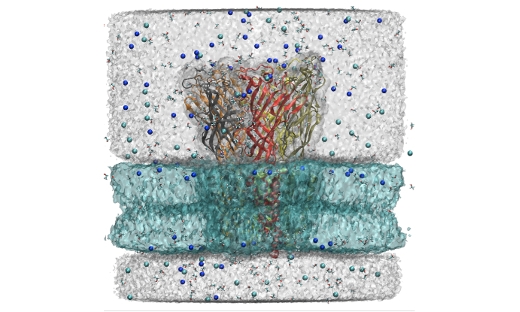
Molecular Highlight: Unraveling the strokes of ion transporters in computers
As highlighted by the 2013 Nobel Prize for chemistry, life science and biomolecular modeling are some of the most important applications for molecular simulation. By simulating the motions of membrane protein transporters in close connection with experiments, we have been able to explain fundamental mechanisms of nerve signal propagation both inside and between cells. …
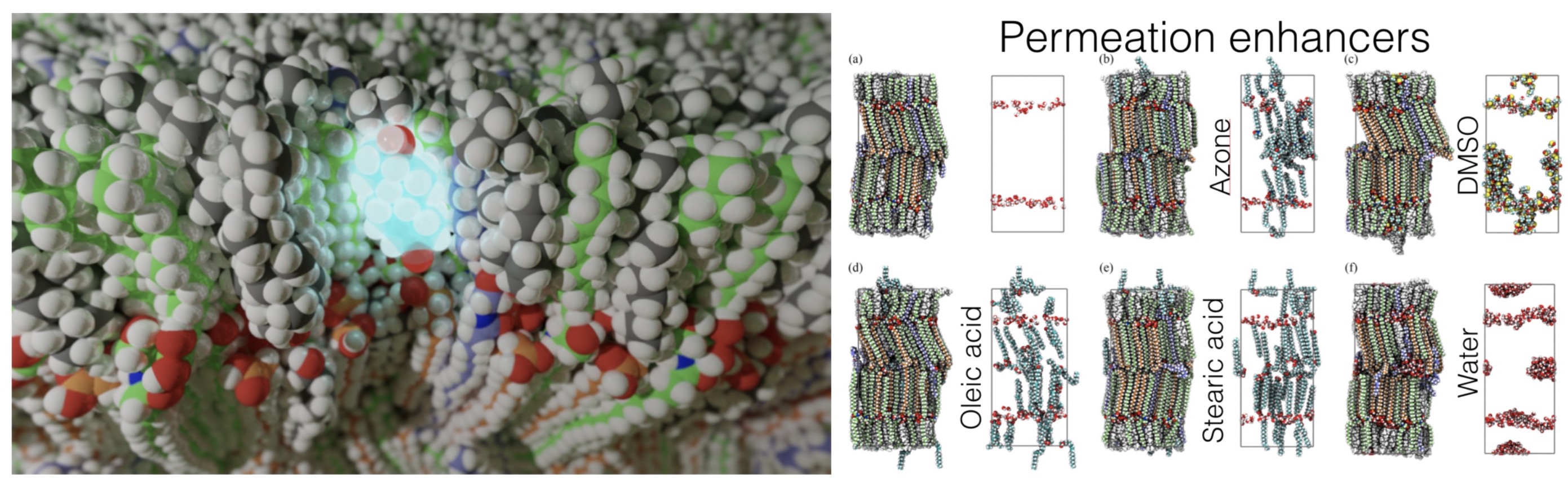
Molecular models of the skin from cemovis data and simulations of permeation
In a collaboration between SeRC researchers at SU, KTH, and KI as well as ERCO Pharma AB, we have developed new computational methods to fit molecular data to cryo-EM image data of vitreous sections (CEMOVIS) of skin.
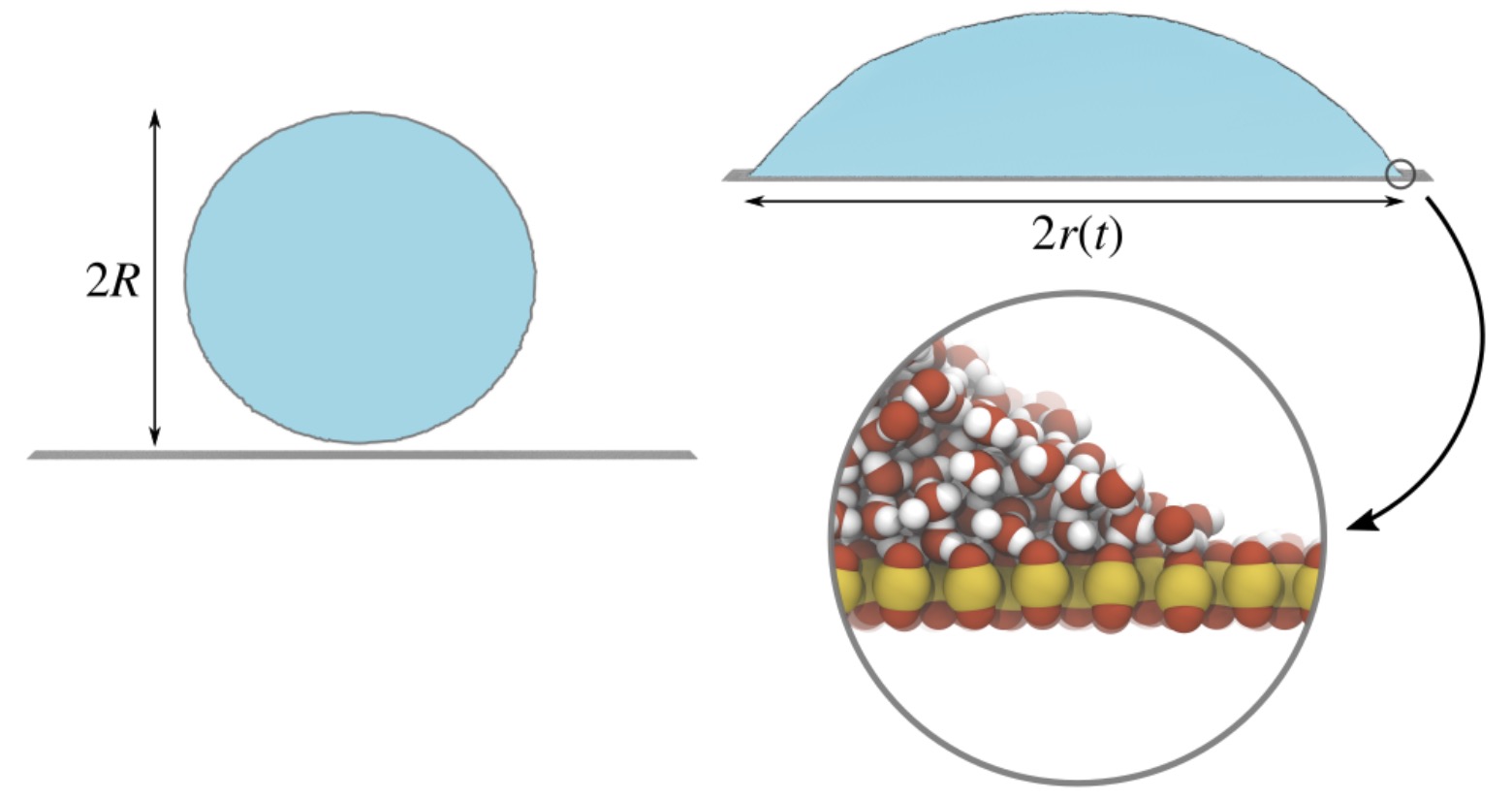
Molecular origin of contact line friction in dynamic wetting
The dynamics of a droplet spreading on a substrate is often strongly affected by friction at the three-phase contact line, where vapour, liquid and substrate meet. Since water does not slip on a hydrophilic substrate, the contact line can only move by water molecules “jumping” ahead of the current contact line.
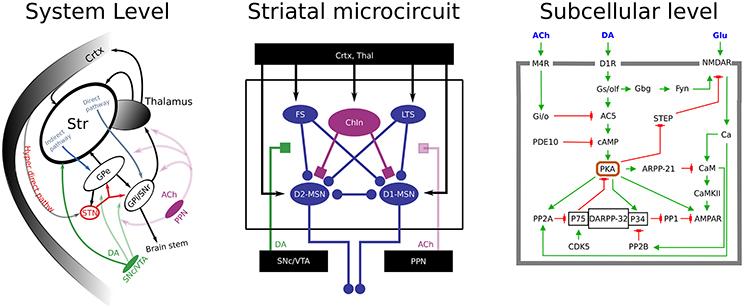
Multiscale simulations
We have integrated models of receptor induced subcellular signaling into a quantitatively detailed neuron model of a striatal projection neuron to be able to investigate the underlying mechanisms of fast dopaminergic neuromodulation.
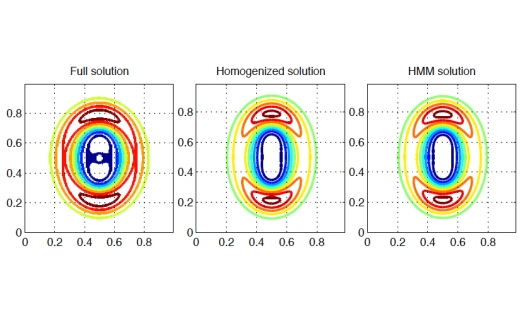
NA Highlight: Multi-scale methods for wave propagation
A new type of multi-scale numerical method for simulation of wave problems has been developed and analyzed. …
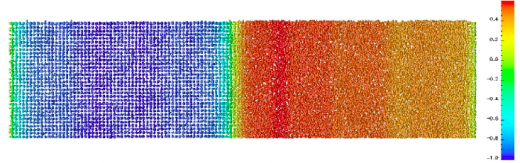
NA Highlight: Numerical methods for molecular dynamics
Langevin dynamics has been derived from the more fundamental Ehrenfest dynamics. …
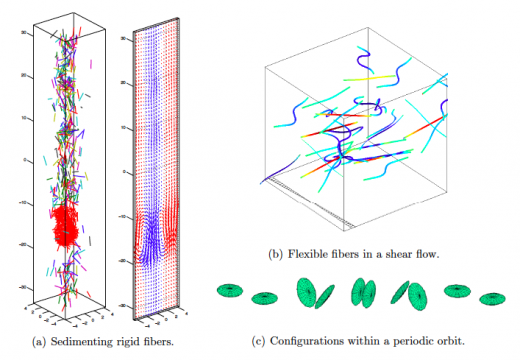
NA Highlight: Spectrally accurate fast Ewald summation
A new highly accurate method for the evaluation of forces resulting from electrostatic interactions has been developed. …
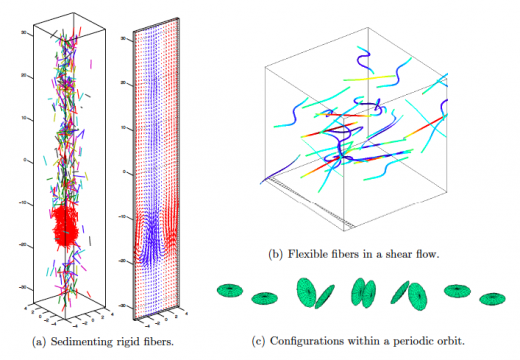
NA Highlight: Spectrally accurate fast Ewald summation
A new highly accurate method for the evaluation of forces resulting from electrostatic interactions has been developed.
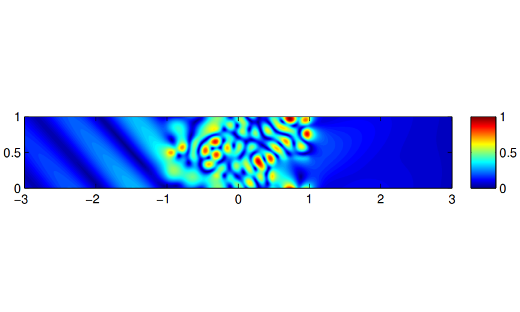
NA Highlight: Structured iterative methods for waveguide eigenvalue problems
In the field of electromagnetics, certain types of wave propagation can be modeled with waveguides, and can be analyzed by discretizing an associated partial differential equation. …
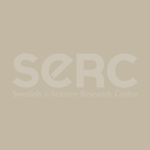
Nek5000
We will focus on two aspects in further developing the Nek5000 code within SESSI. First, we will porting Nek5000 to accelerators using OpenACC and CUDA. We will continue the effort of programming Nek5000 for using accelerators to perform batched small matrix matrix multiplication that this the main computational kernel affecting Nek5000 performance. This work will also include optimization with the possibility of using CUDA in combination with OpenACC and improve the efficiency of data movement between host and GPU memories in the GS operator code. This work will be done in collaboration with the EC-funded exascale EPiGRAM-HS project that is led by PDC, and researchers at the Argonne National Laboratory. Second, we will consider new formulations of the compute and communication intensive kernels of Nek5000, including the main communication library gslib. We continue our work on one-sided communication primitives into this kernel via UPC, a PGAS programming system taking advantage of modern network hardware support for efficient one-sided communication. This includes the expertise of Niclas Jansson who developed an initial proof-of-concept of such new software. Features of new languages will also be used to overlap computation and communications by re-organising the flow of the communication.
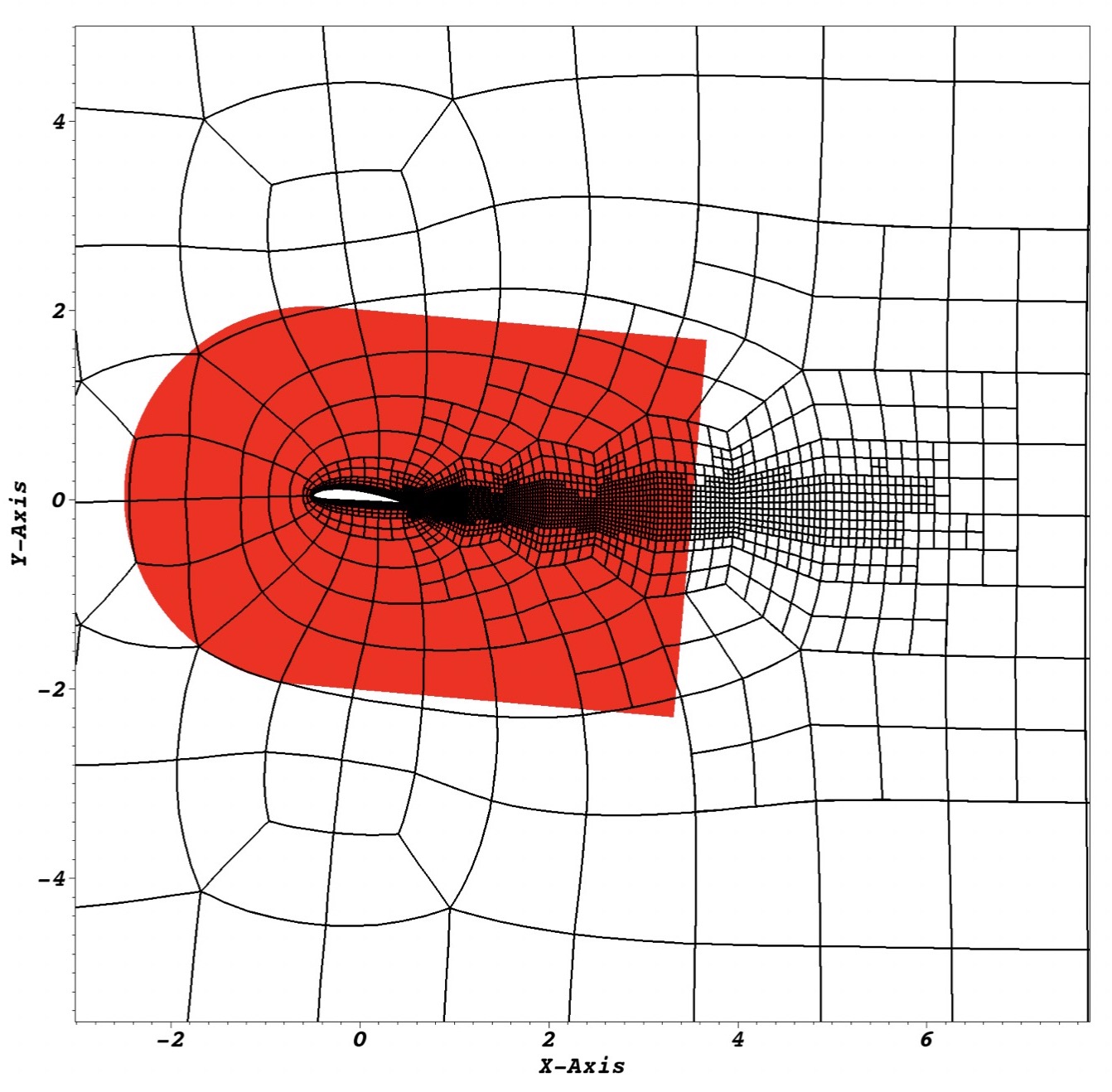
Non-conformal simulations in the high-order code Nek5000
Our continuing work on extending the open-source code Nek5000, based on the high-order spectral element method, has now reached a level of maturity such that we can – for the first time – perform simulations of turbulence in complex geometries.
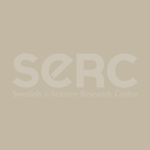
NumericalAlgebra
We aim to develop new better numerical algorithms for certain problems stemming from data science and machine learning, by using state-of-the-art numerical linear algebra techniques and software and solve the corresponding computationally demanding core problems.
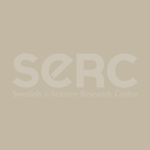
Ontologies for the materials science domain
Ontologies standardize terminology in a domain and are a basis for semantically enriching data, integration of data from different databases, and reasoning over the data. They deal with the big data issues of Variety, Variability, and Veracity.
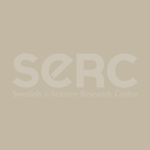
Open Space: A tool for space research and communication
OpenSpace is an international open source software development project, with its seat in Norrköping. It is designed to visualize data in astronomy-related research and development.
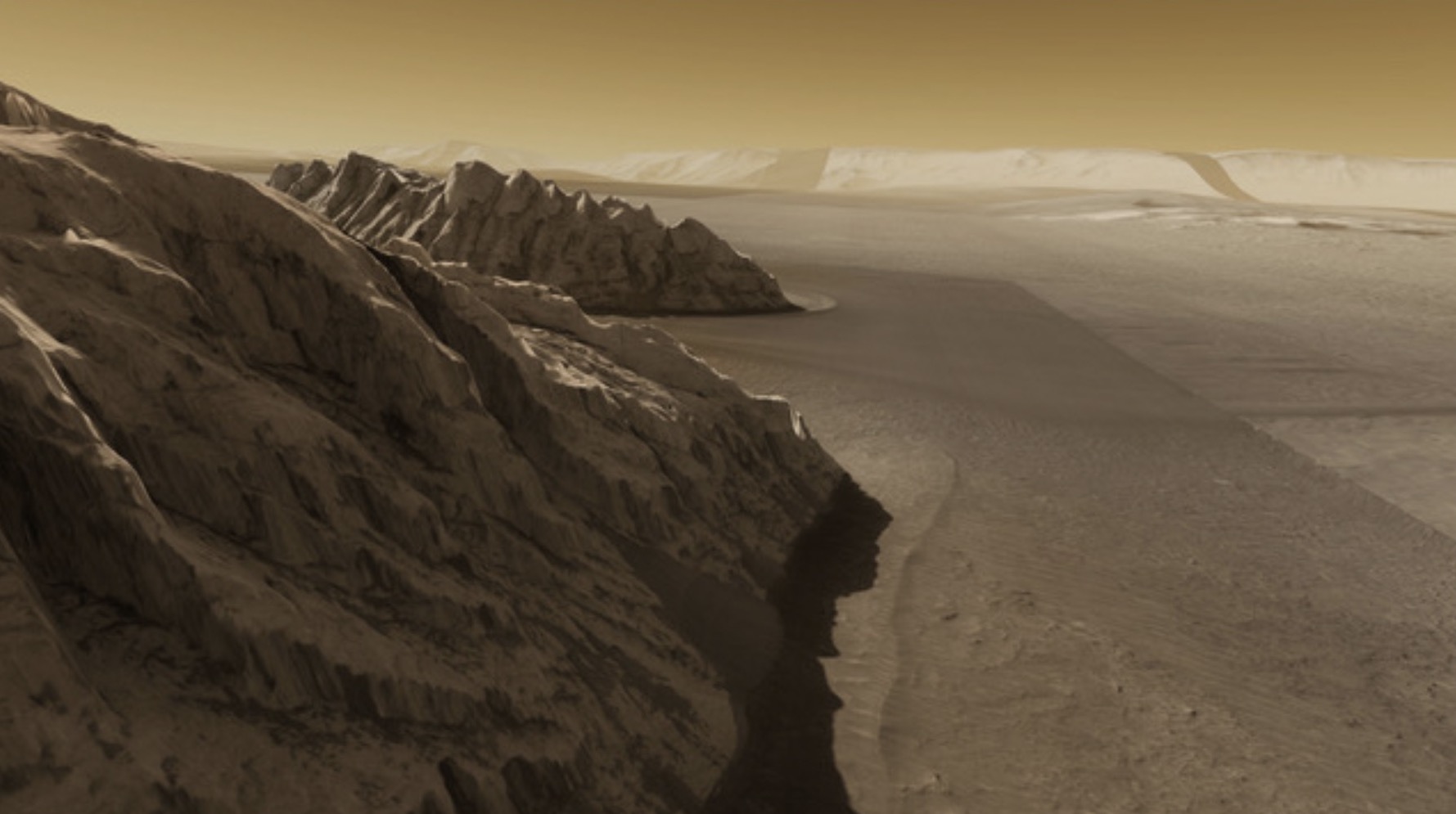
OpenSpace research results in best paper award at IEEE vis conference 2017: Globe browsing: Contextualized spatio-temporal planetary surface visualization
Results of planetary mapping are often shared openly for use in scientific research and mission planning.In its raw format, however, the data is not accessible to non-experts due to the difficulty in grasping the context and the intricate acquisition process. The OpenSpace software enables interactive contextualization of geospatial surface data of celestial bodies for use in science communication.
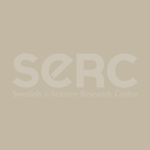
Organic materials for the future
Atomistic MD simulations of polymer- and cellulose-based materials are performed to investigate the impact of ionic liquid on the morphology of systems. We will focus on the dynamics of the capacitive charging, which will provide us with the information of the mechanism of doping/dedopinng on the atomistic scale of the intrinsic capacitance in the presence of ionic liquid.
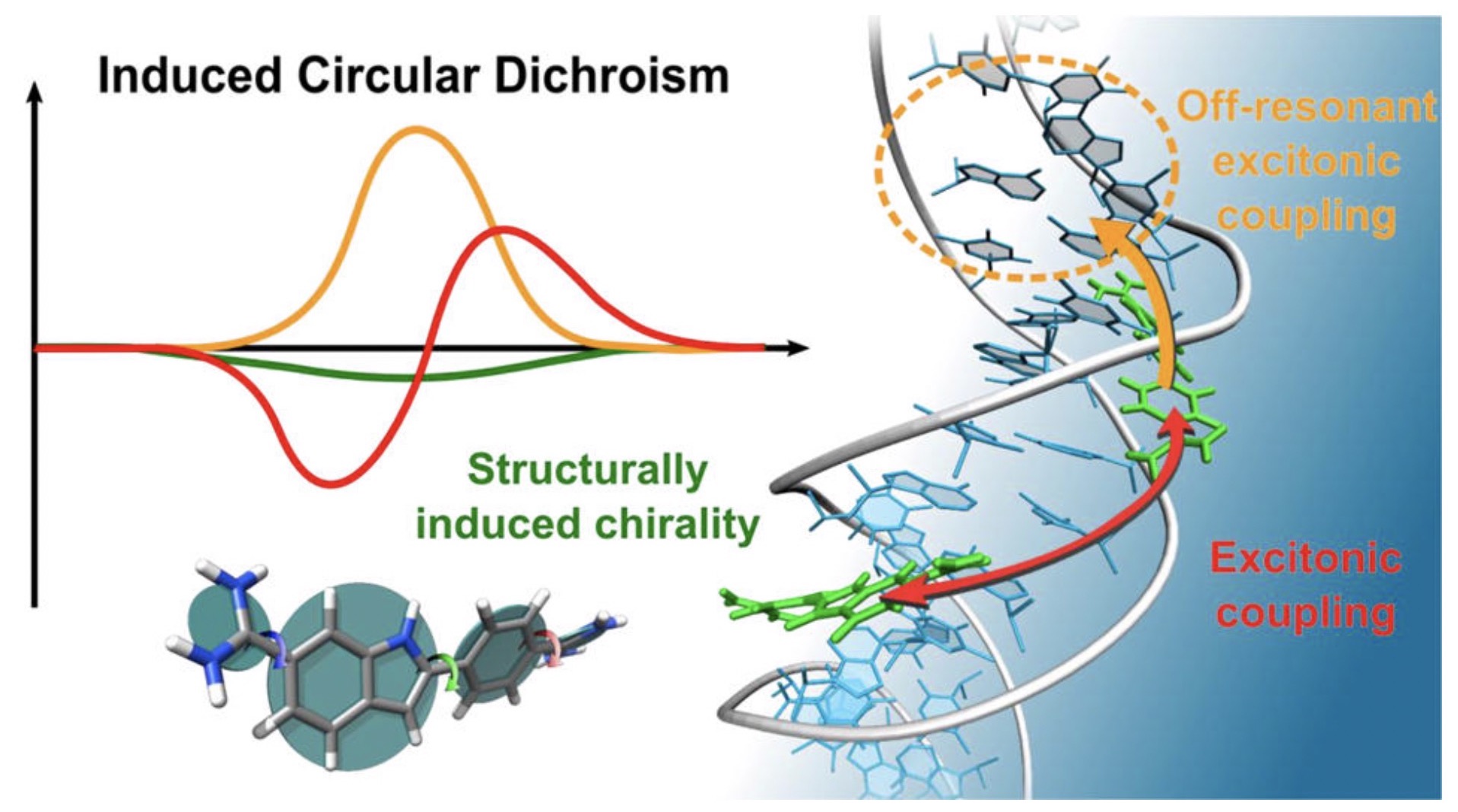
Origin of DNA-Induced Circular Dichroism in a Minor-Groove Binder
With a combination of molecular dynamics simulation, CD response calculations, and experiments on an AT-sequence, we show that the ICD originates from an intricate interplay between the chiral imprint of DNA, off-resonant excitonic coupling to nucleobases, charge-transfer, and resonant excitonic coupling between DAPIs.
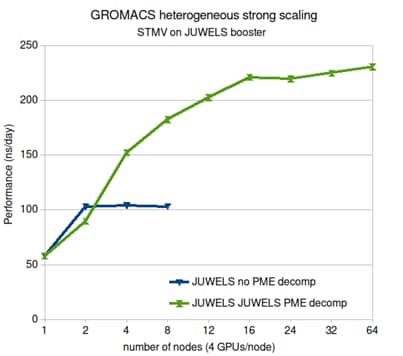
Parallelization of electrostatic over multiple GPUs in GROMACS
In most molecular dynamics simulations the 3D fast Fourier transform used for calculating the long-range electrostatic interactions is what limits parallelization.
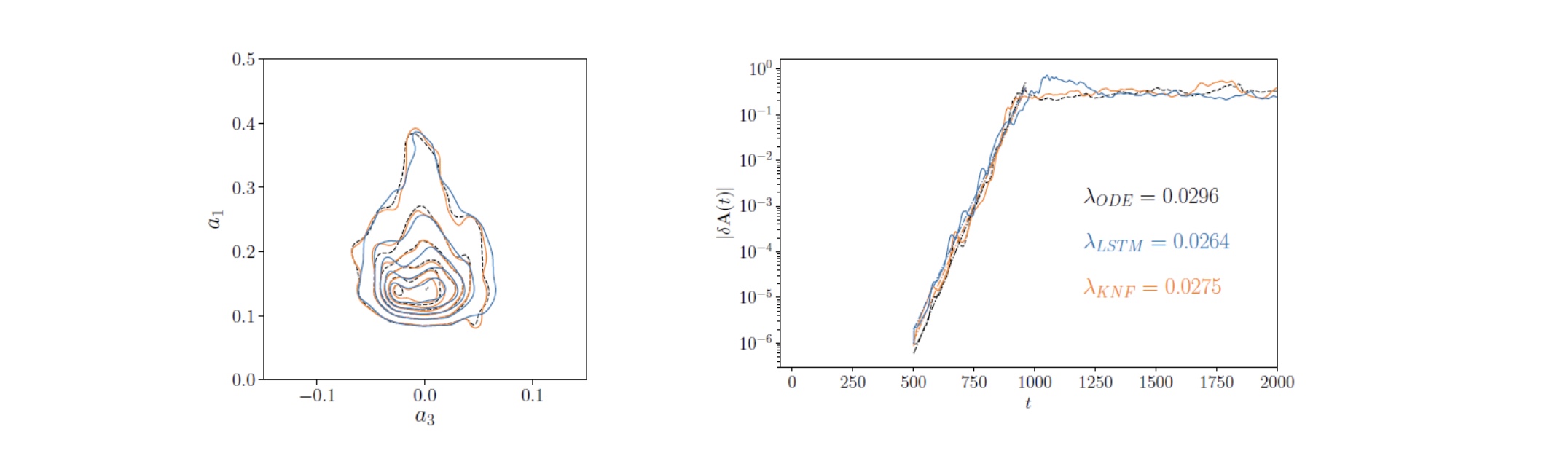
Pioneered the use of recurrent neural networks for temporal predictions in turbulent flows
SeRC researchers have assessed the prediction capabilities of long-short-term memory (LSTM) neural networks
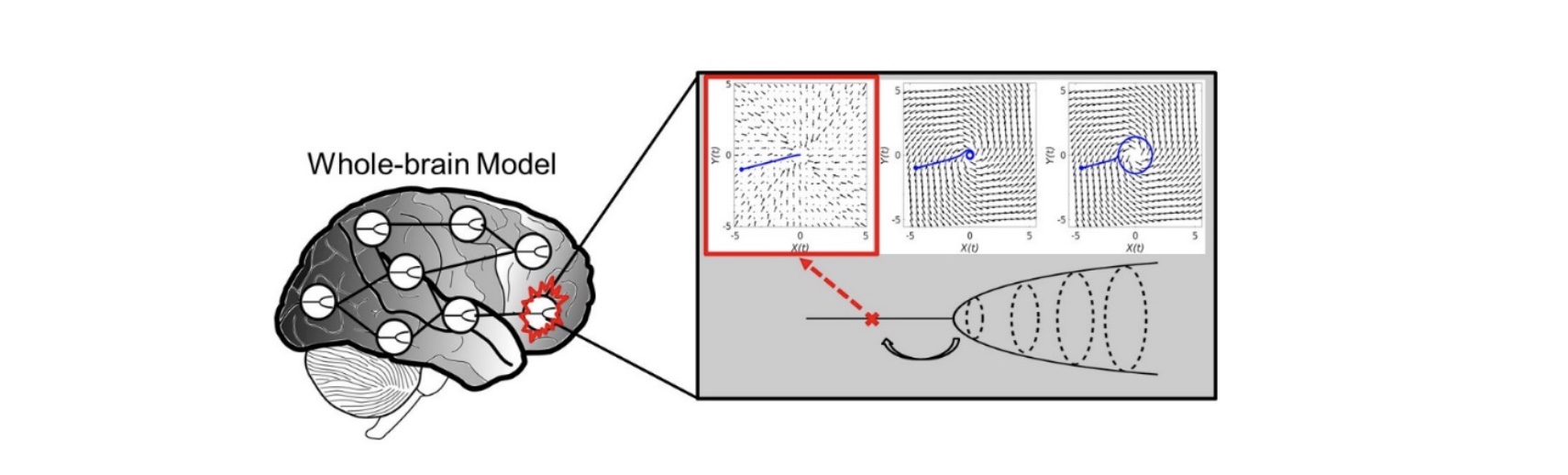
Predicting regional vulnerability in the brain using dynamic modeling of resting-state fMRI data
A new macroscopic computational model of brain oscillations in resting-state fMRI data was developed and evaluated. Results show that the effect of local lesions can be captured and studied by making regional changes in oscillation dynamics.
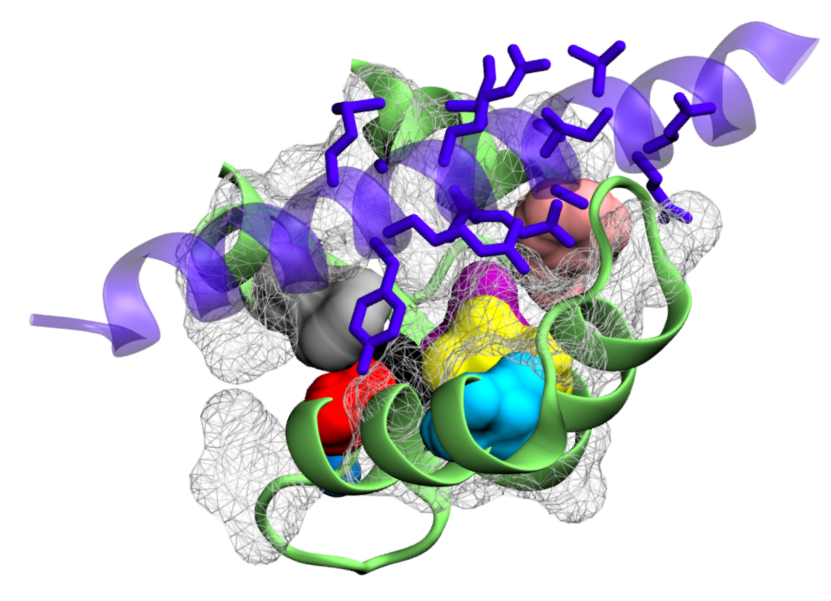
Promiscuous and selective calmodulin
Calmodulin is a ubiquitous calcium sensor that confers calcium sensitivity to many cellular partners. How calmodulin can bind a large number of targets while retaining some selectivity, a phenomenon called promiscuous selectivity, is a fascinating question that we address with molecular dynamics simulations conducted with GROMACS.
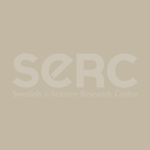
Protein modeling quality
Protein modeling quality is an important part of protein structure prediction. The new quality predictor ProQ3D was developed using deep learning techniques and could improve prediction accuracy relative to previous methods (Uziela et al 2018). ProQ3D was shown to perform at top in the CASP13 evaluation.
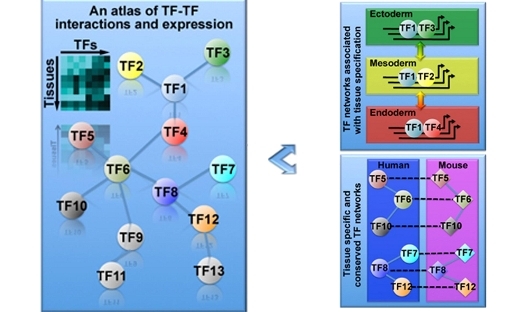
PSDE Highlight: An atlas of combinatorial transcriptional regulation in mice and man
Availability of large TF combinatorial networks in both humans and mice will provide many opportunities to study gene regulation, tissue differentiation, and mammalian evolution. …
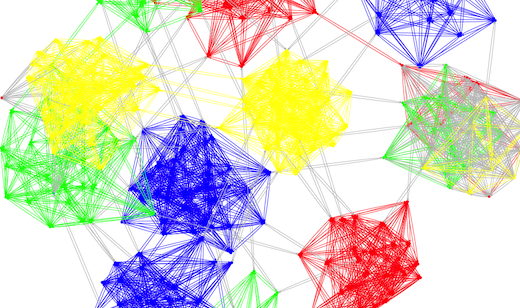
PSDE Highlight: Decentralized Graph Partitioning for Social Networks and Classification Systems
SeRC researchers have developed a novel decentralized method for partitioning of graphs, with applications in areas such as social networks and classification systems, that was awarded with best paper in the IEEE International Conference on Self-Adaptive and Self-Organizing Systems, 2013.
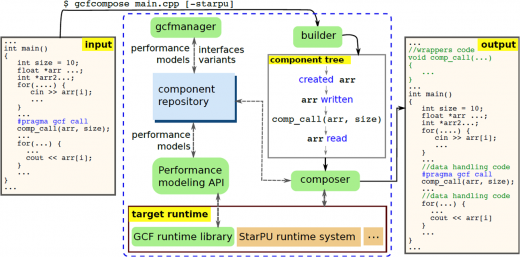
PSDE Highlight: Enabling efficient and future-proof HPC applications: High-level component-based programming frameworks for heterogeneous parallel systems
Recent disruptive changes in computer hardware (in particular, the transition to multi-/manycore and heterogeneous architectures) have led to a crisis on the application software side: efficient programming for modern parallel and heterogeneous systems has become more tedious, error-prone and hardware specific than ever. In particular, this holds for GPU-based systems that are increasingly popular in high performance computing, with GPU architecture evolving quickly – but which application writer has the time to rewrite and/or re-optimize his/her code for each new hardware generation? …
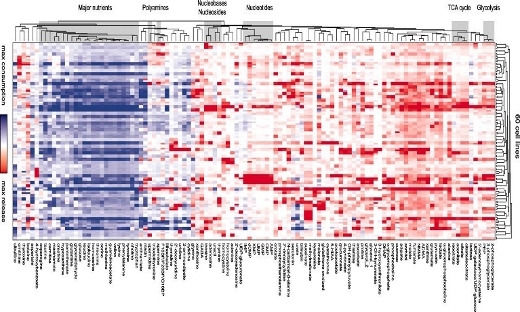
PSDE Highlight: Metabolite profiling identifies a key role for glycine in rapid cancer cell proliferation
Glycine consumption and expression of the mitochondrial glycine biosynthetic pathway seem to be strongly correlated with rates of growth across cancer cells. …
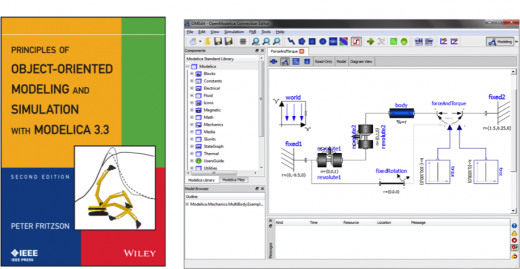
PSDE Highlight: Object-Oriented Modeling and Simulation with Modelica
The modeling language Modelica is bringing about a revolution in the area of simulating complex cyber-physical systems for e.g. robotics, aircrafts, satellites or power plants. …
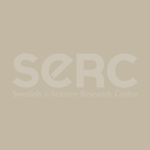
PSDE Highlight: Pediatric systems medicine: evaluating needs and opportunities using congenital heart block as a case study
Executing a systems medicine programme in pediatrics creates potential for collaboration between clinicians and families who are keen to prevent and predict diseases and nurture wellness in the families’ children. …
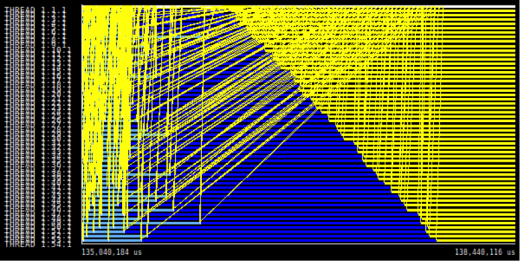
PSDE Highlight: Scalable Performance Monitoring
Understanding how parallel applications behave is crucial for using HPC resources efficiently. Particularly, exascale systems will be composed by heterogeneous architectures with multiple levels of concurrency and energy constraints. In such complex scenarios, performance monitoring and runtime systems will play a major role to obtain good application performance and scalability. SeRC researchers have developed techniques for online access to performance data and efficient data formats for performance data.
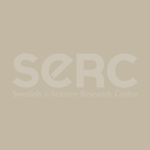
PSDE Highlight: World Record Hadoop Performance
Led by Dr Jim Dowling, in 2016, SeRC researchers from the PSDE community announced world-record performance for the Hadoop platform, with their next-generation distribution of Apache Hadoop File System, HopsFS. …
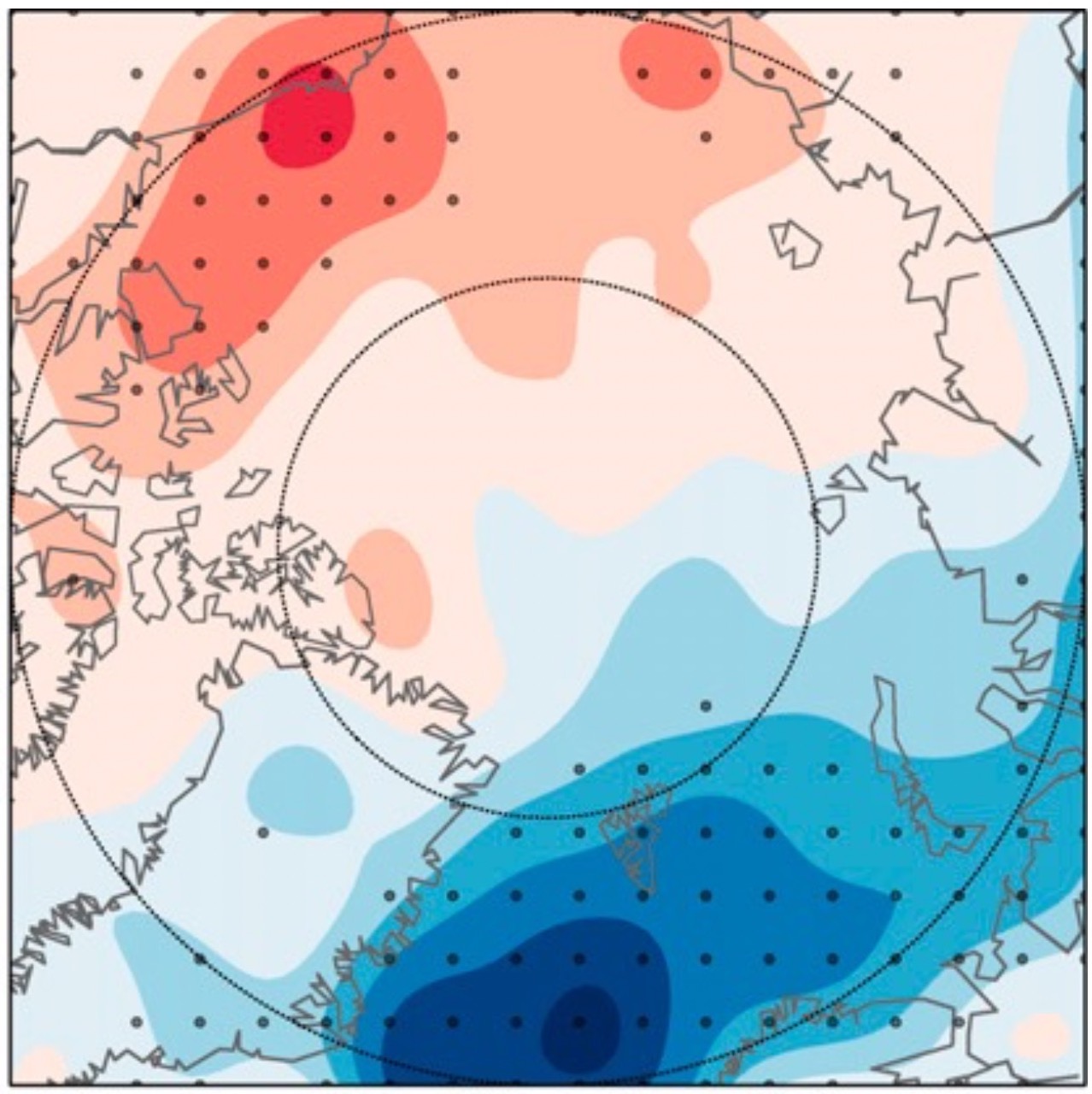
Representation of Arctic moist intrusions in global coupled climate models
Events with warm and moist air entering the Arctic have been shown to have a substantial effect on the surface temperature climate in winter. Here, the coupled global climate models participating in the Coupled Model Intercomparison project Phase 5 (CMIP5) are evaluated with respect to these events.
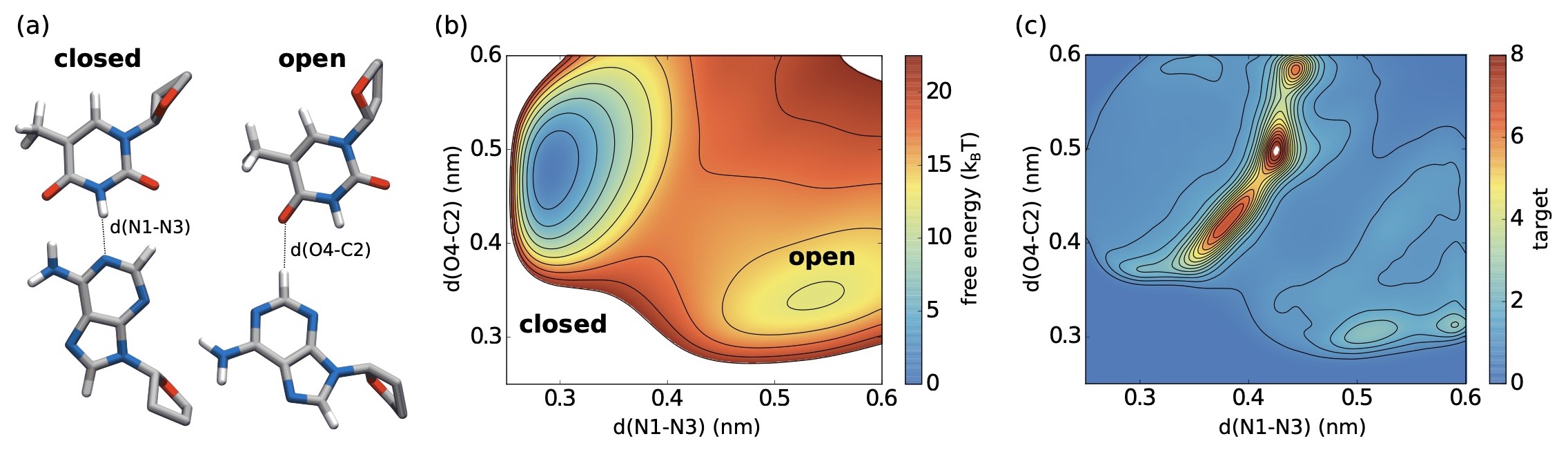
Riemann metric approach to optimal sampling of multidimensional free-energy landscapes
Exploring the free-energy landscape along reaction coordinates or system parameters λ is central to many studies of high-dimensional model systems in physics. In simulations this usually requires sampling conformational transitions or phase transitions, but efficient sampling is often difficult to attain due to the roughness of the energy landscape.
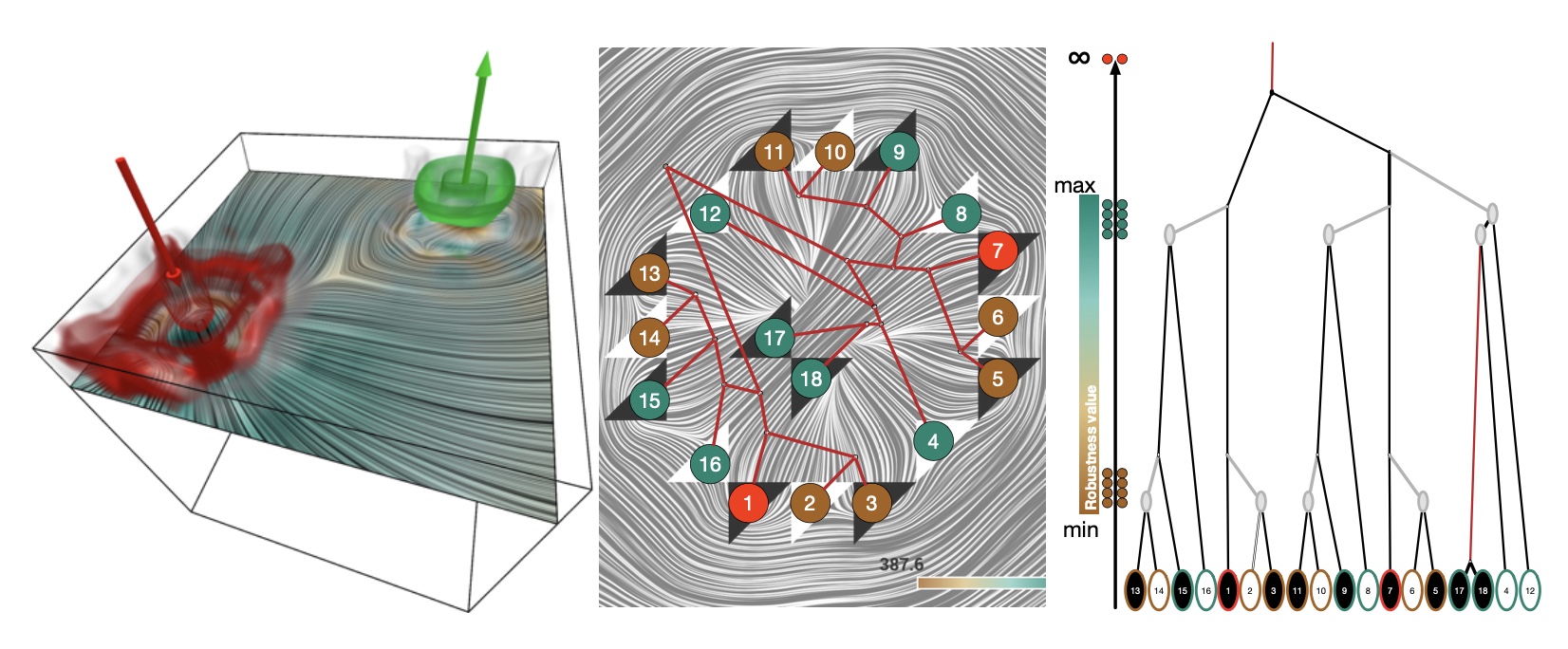
Robust Extraction and Simplification of 2D Tensor Field Topology
Research focus area – Topological methods for dynamical data
Application – Materials for the future
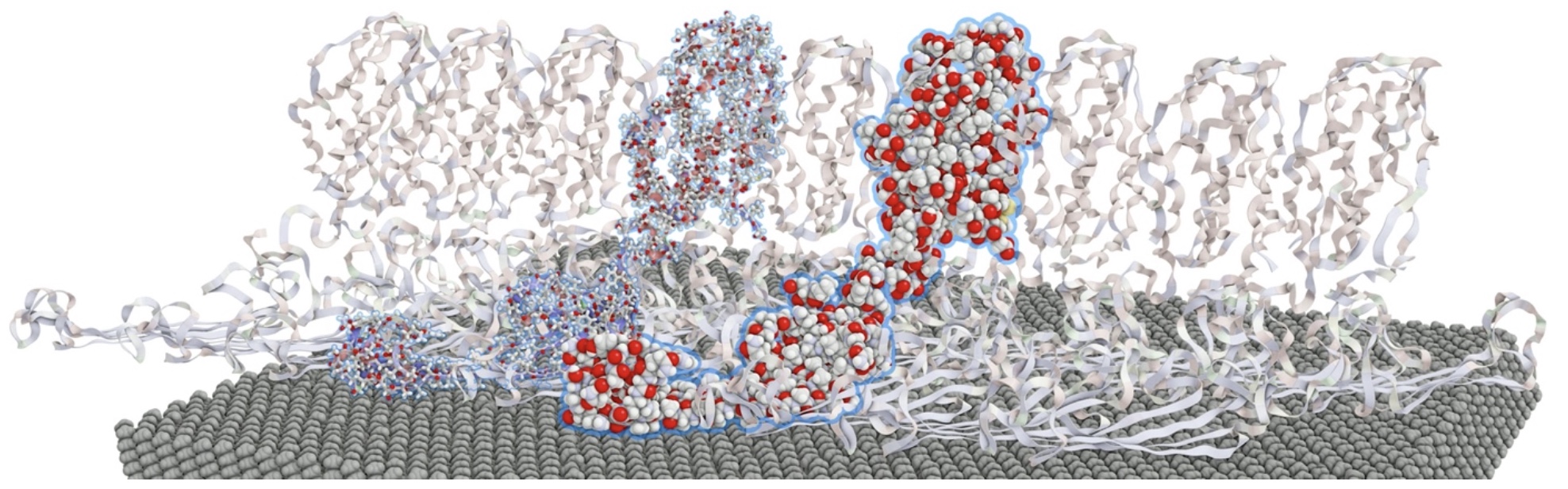
Selection Concepts for Complex Molecular Structures
Research focus area – Multi-scale visualization
Application – Molecular dynamics simulations for conformation analysis
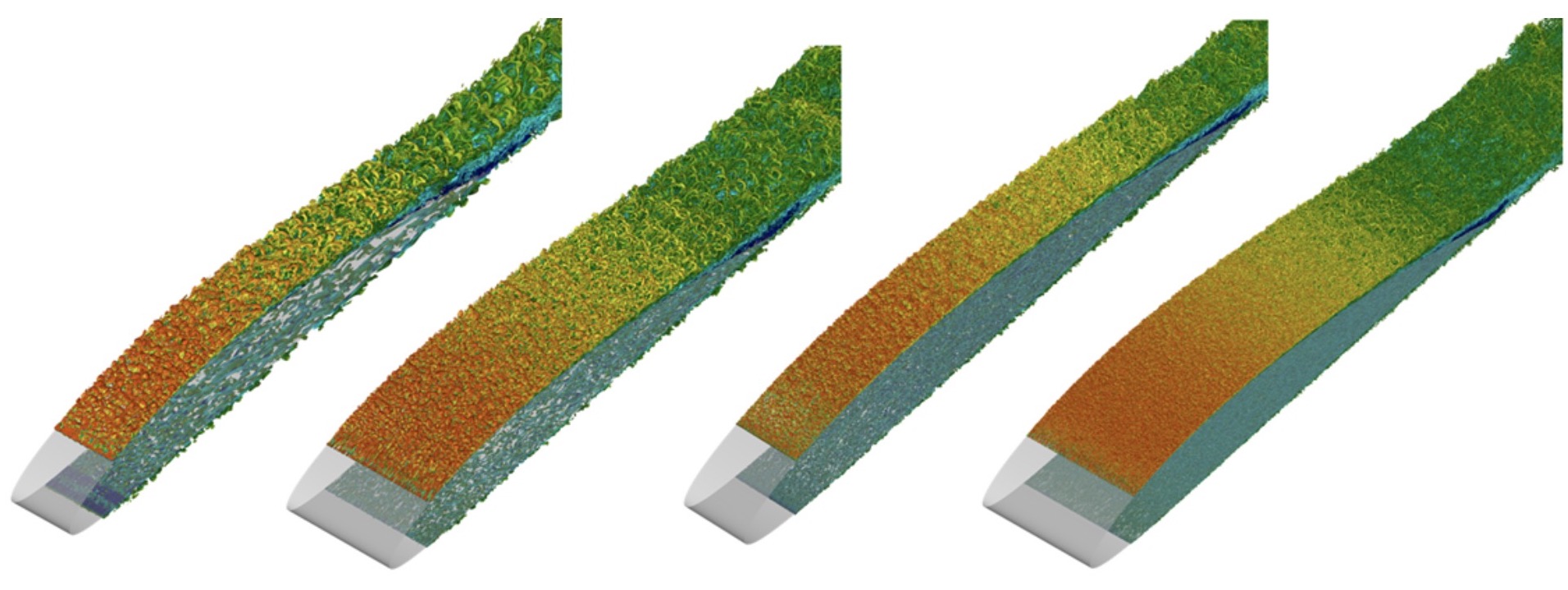
Simulation of turbulent wings at various Reynolds numbers.
Continuing with our efforts on understanding the effect of pressure gradient and curvature on turbulent wings, we have now constructed a database consisting of time and spatially resolved turbulence around wings at 4 Reynolds numbers, starting at the low Re=100k up to the (computationally high) 1M.
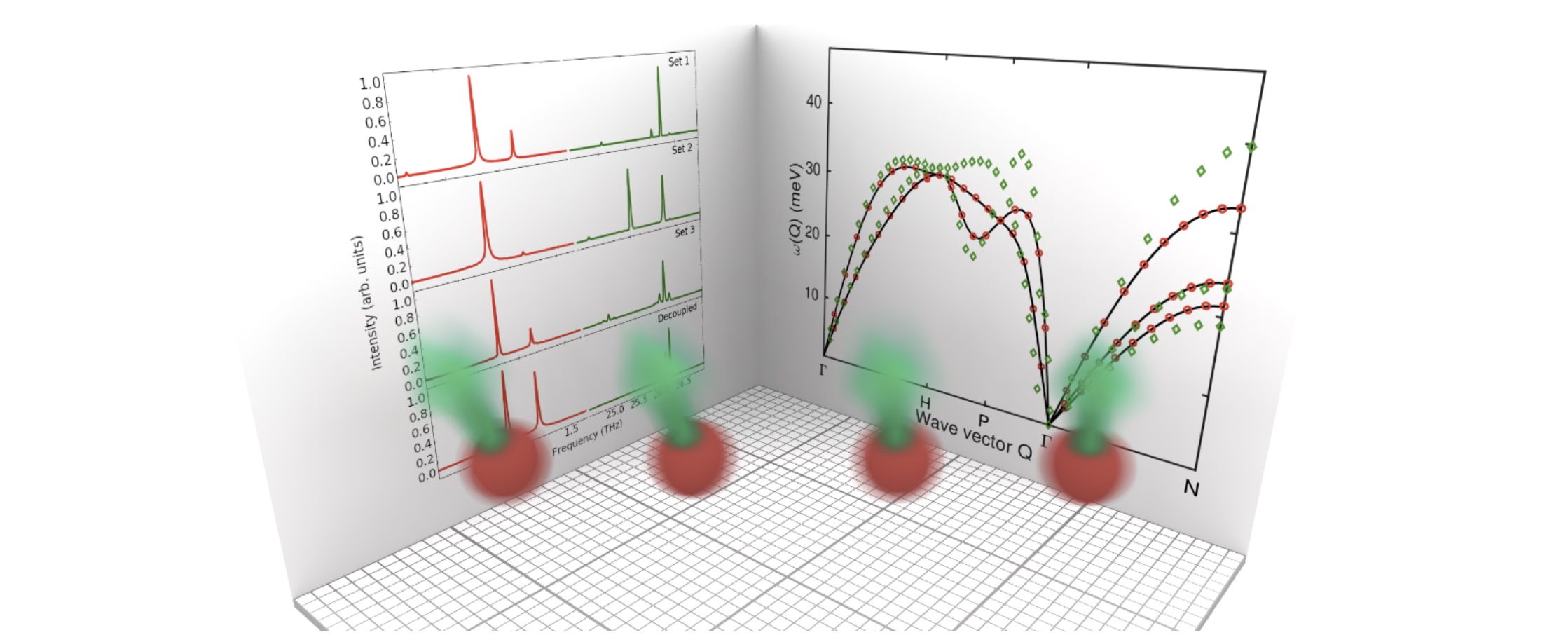
Simultaneous simulations of magnon and phonon dynamics
The atomistic spin-lattice code is up and running and our first paper describing the method has been published.
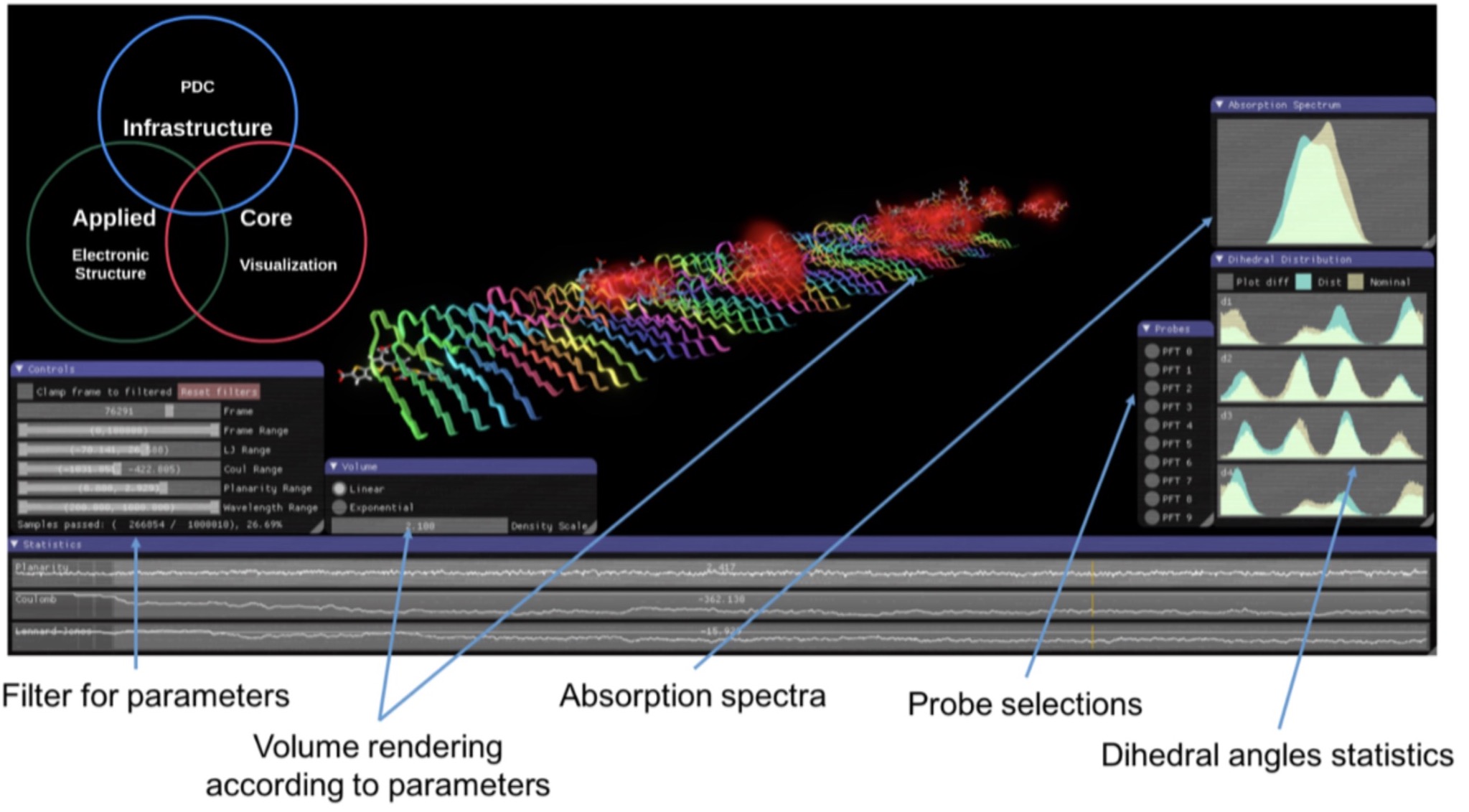
Software development for exploration and design of complex molecular systems
The dominating software for quantum molecular simulations is an American commercial product (Gaussian). In an undertaking together with PDC, we will develop a full-fledged DFT program with all the standard capabilities as well as non-standard functionalities developed in the Scandinavian Dalton program community and which provides state-of-the-art scaling on contemporary and future HPC hardware platforms based on Intel, ARM, and Power CPUs as well as NVIDIA GPUs.
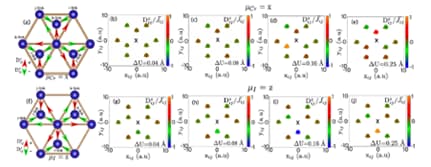
Spin-lattice couplings in two-dimensional from first-principles computations
Since thermal fluctuations become more important as dimensions shrink, it is expected that low-dimensional magnets are more sensitive to atomic displacement and phonons than bulk systems are.
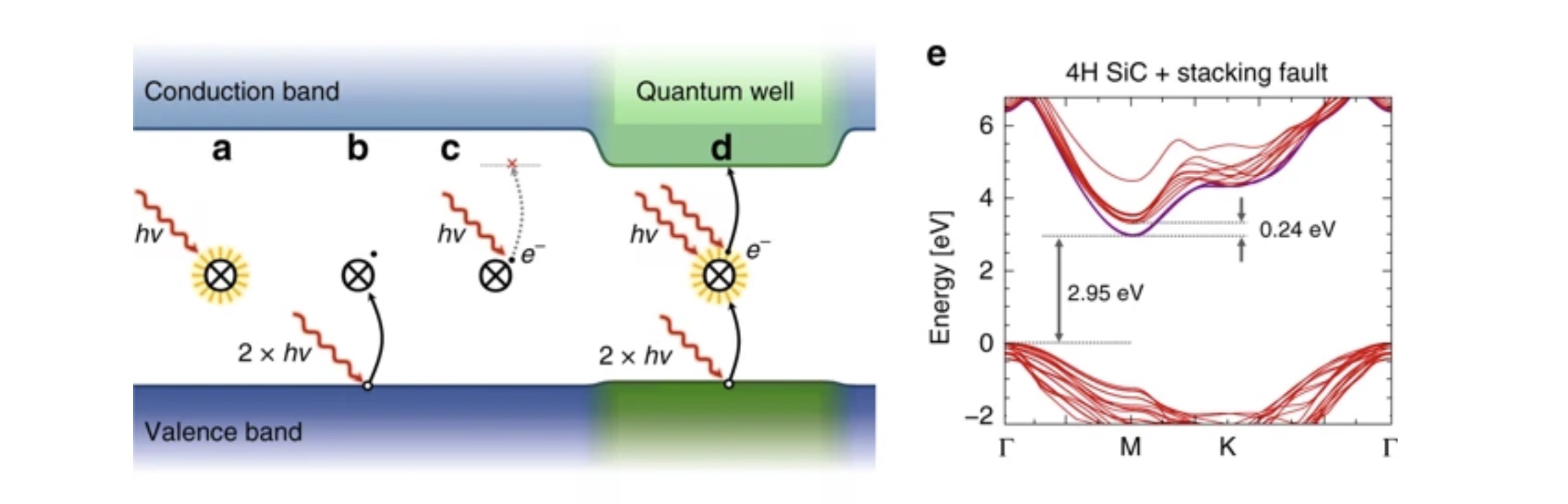
Stabilization of point-defect spin qubits by quantum wells
Defect-based quantum systems in wide bandgap semiconductors are strong candidates for scalable quantum-information technologies.
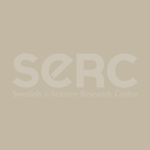
StochasticSimulation
The aim of this project is to develop stochastic simulation methods in Machine Learning along with a corresponding rigorous efficiency analysis.
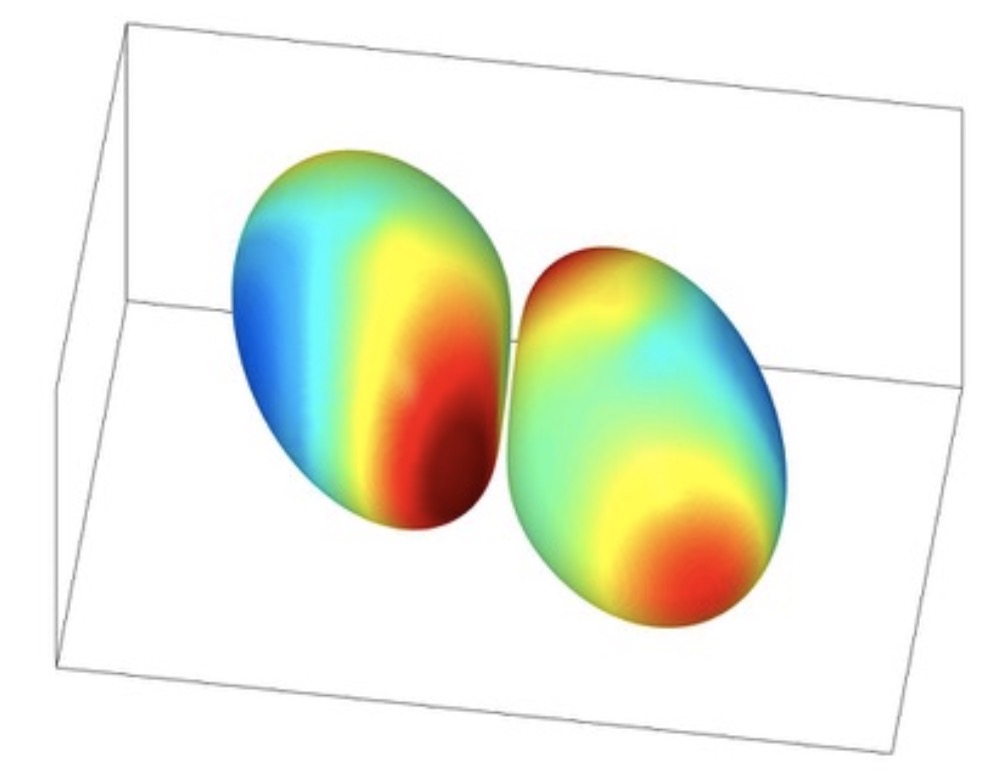
Stokes flow for particles and drops
Research related to integral equation based numerical methods mainly for Stokes flow with particles and drops have been investigated in the community.
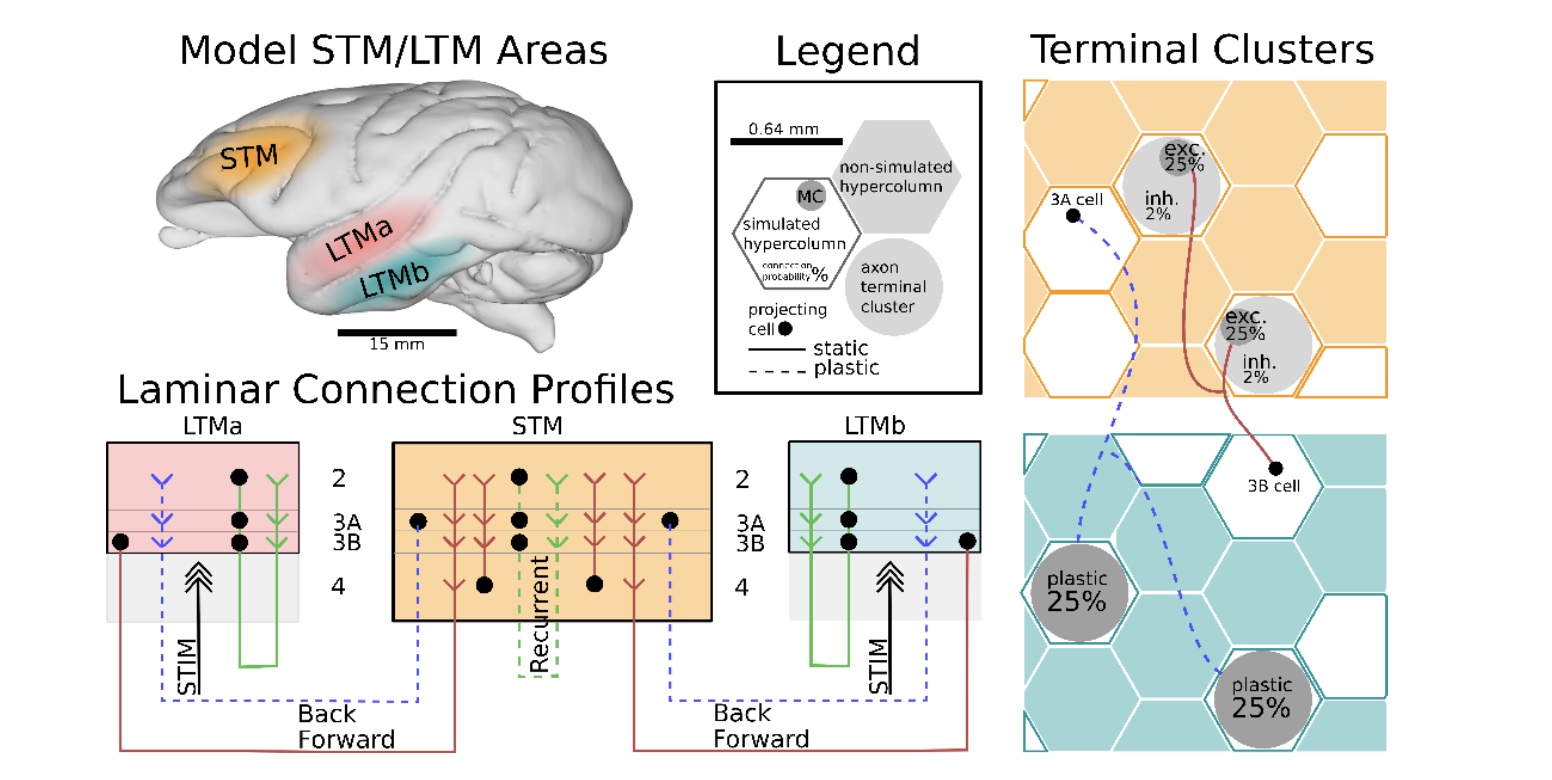
Synaptic theory of working memory explains most recent experimental findings about neural correlates of working memory
Our spiking neural network attractor memory model accounting for a synaptic theory of working memory has been validated using experimental data to explain the most recent observations about bursty nature of neuronal spiking as well as gamma- and beta-band oscillations of field potentials.
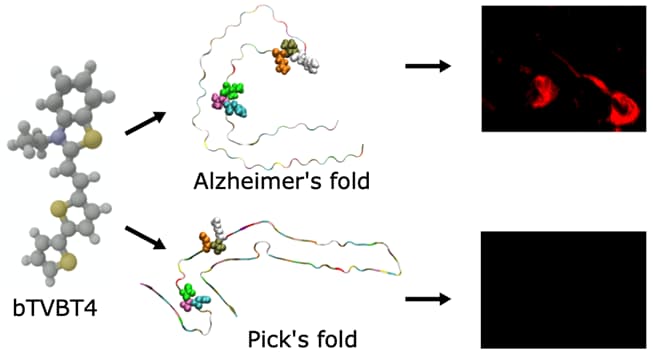
Tau Protein Binding Modes in Alzheimer’s Disease for Cationic Luminescent Ligands
The bi-thiophene-vinylene-benzothiazole (bTVBT4) ligand developed for Alzheimer’s disease (AD) specific detection of amyloid tau has been studied by a combination of several theoretical methods and experimental spectroscopies.
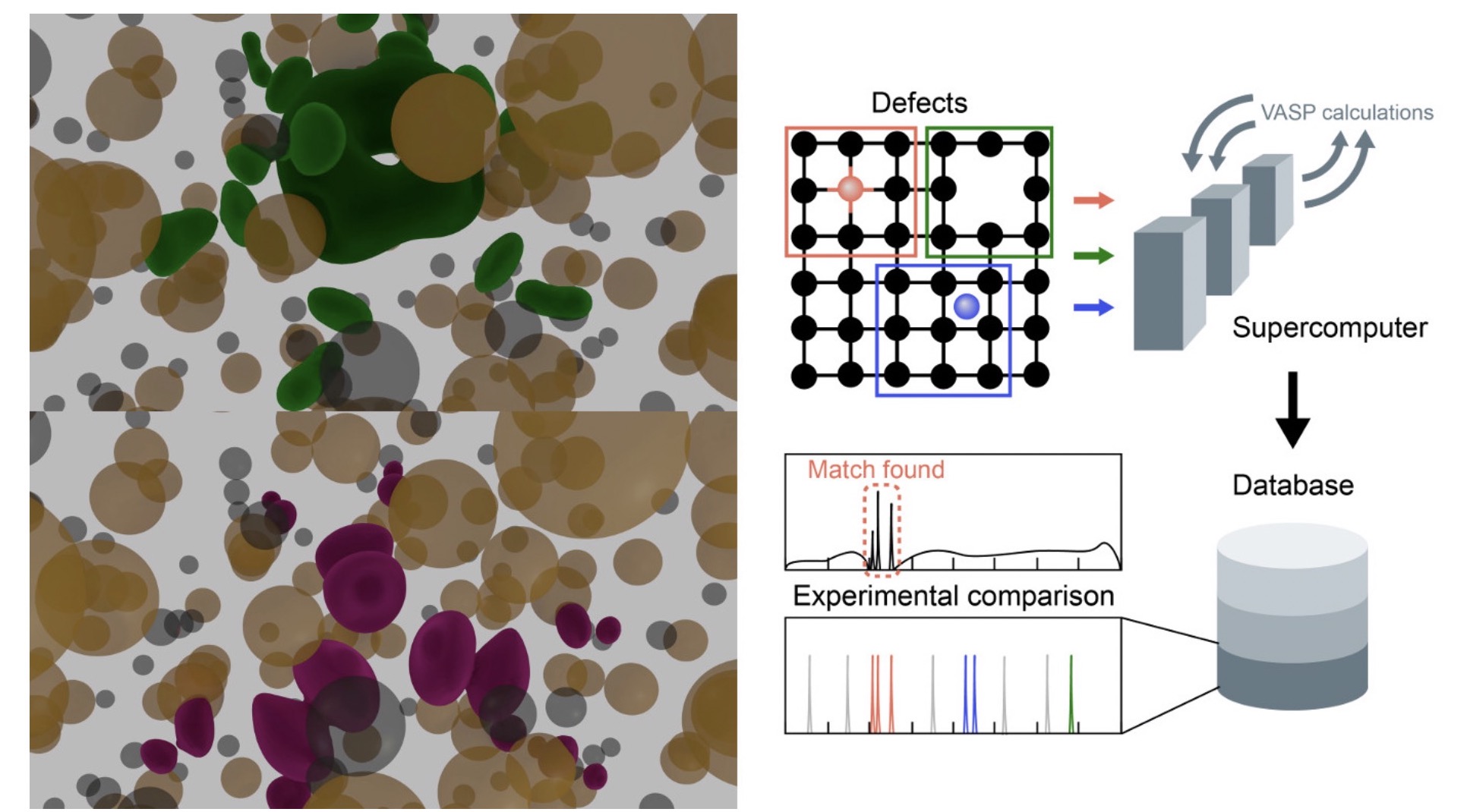
Theoretical Characterization of Point Defects in Silicon Carbide and Other Materials
Silicon carbide (SiC) is a large bandgap semiconductor that is in focus for its potential for applications in quantum information processing. It appears possible to engineer defects in SiC with optical and spin properties that are suitable as single photon sources, and states with long enough lifetime to act as qubit memory.
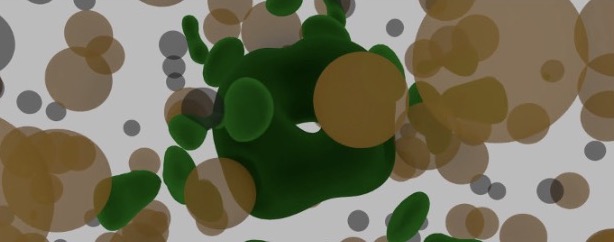
Theoretical Characterization of Point Defects in Silicon Carbide and Other Materials
Effective engineering of materials defects and defect properties on the atomic scale is crucial for creating materials for applications in nanotechnology, i.e., ultra-miniaturization of sensors, storage, processing, and communication.
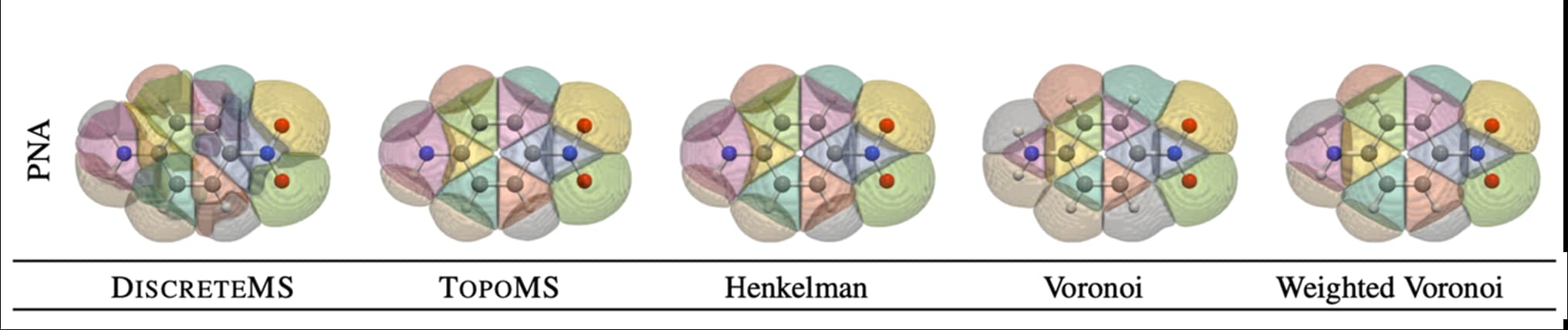
Topological analysis of density fields: an evaluation of segmentation methods for quantum chemistry applications
Topological and geometric segmentation methods provide powerful concepts for analysis and visualization of density fields.
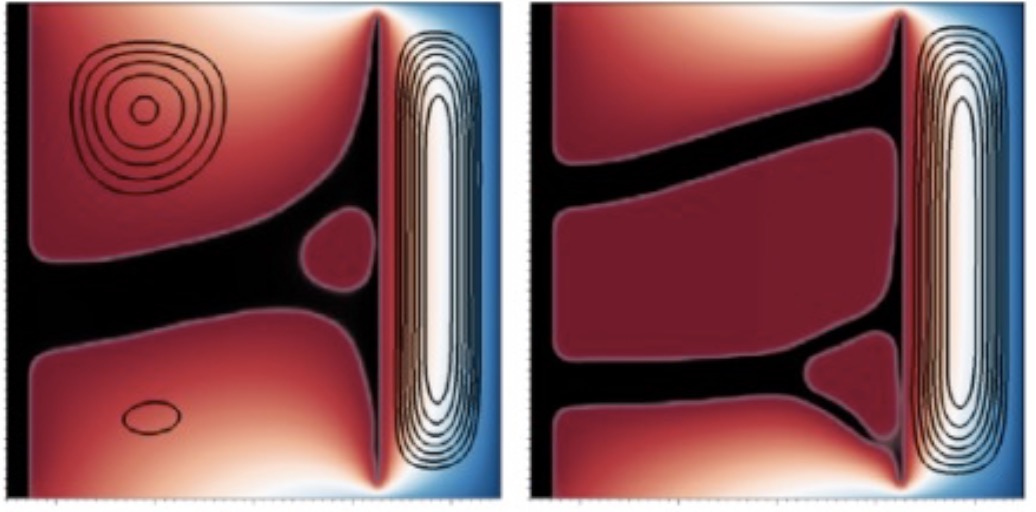
Topology optimization for heat transfer problems
State of the art topology optimization is implemented in our spectral code, to show the potential of performing various types of optimisations using these highly accurate, and scalable codes.
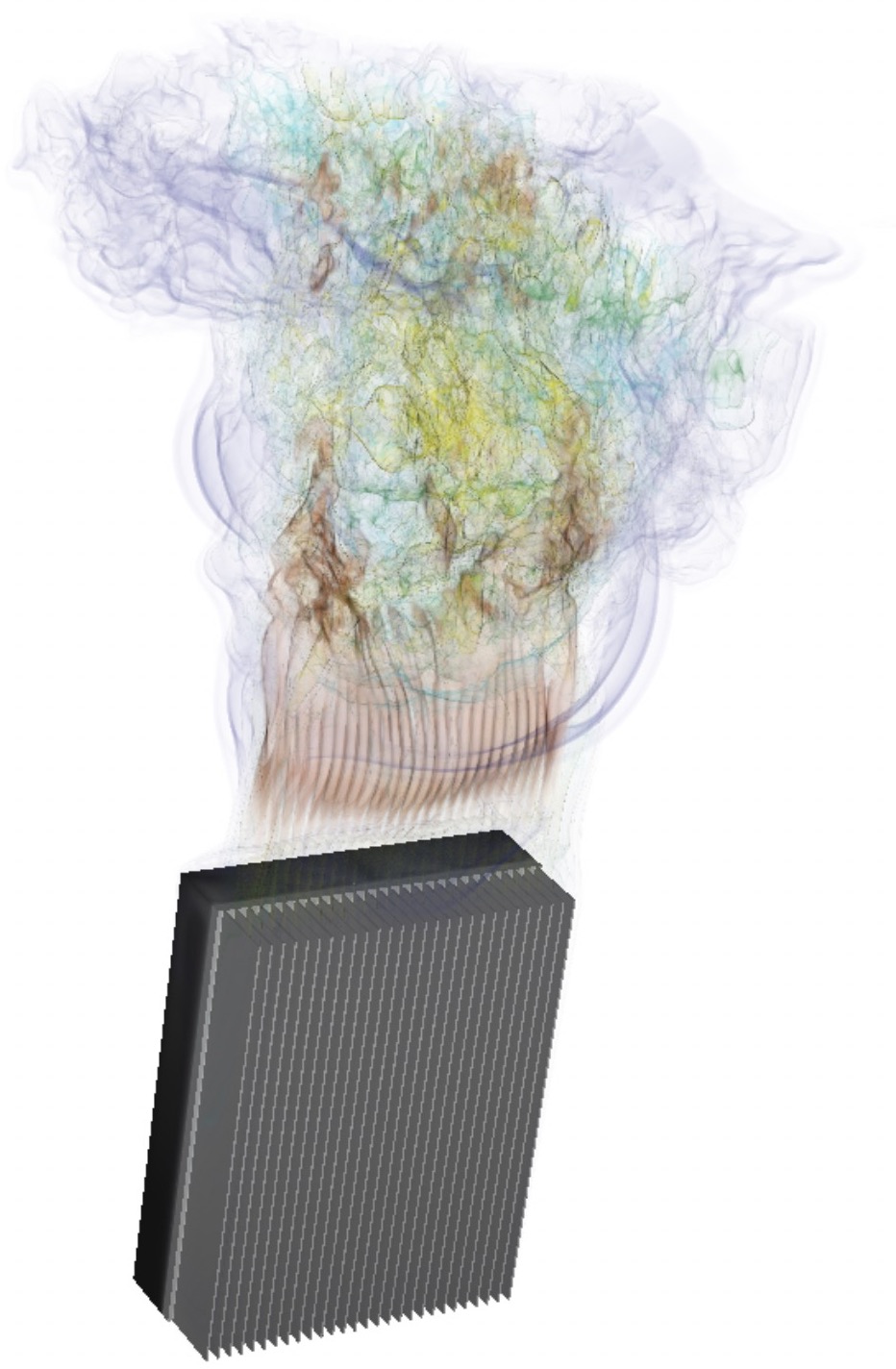
Topology optimization for natural convection flows.
Together with colleagues from Umeå University, we implemented in Nek5000 the possibility to perform high-order accurate topology optimization, useful for flows driven by natural convection.
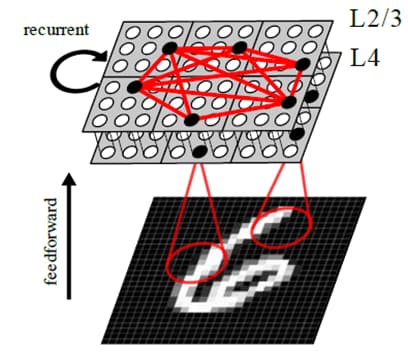
Towards cortex-like neural network architectures for holistic pattern recognition
Figure legend: Continuing the major effort in developing a brain-like neural network architecture for holistic pattern recognition the focus has been on evaluating its capability to learn input representations.
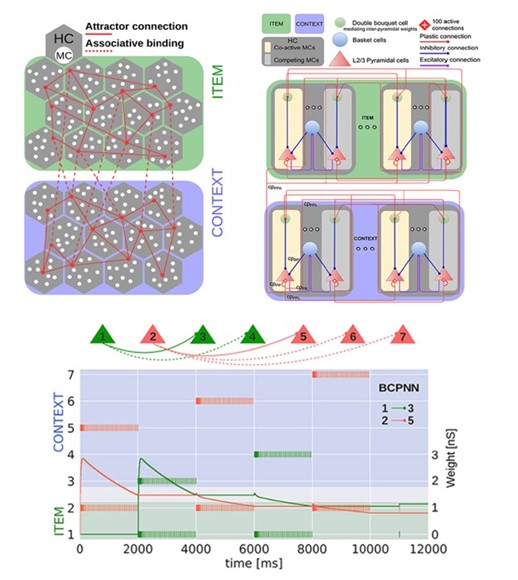
Towards systems network-of-networks modelling of memory phenomena and associative memory bindings
Figure legend: Building on the proposed indexing theory offering a unifying framework for explaining the interactions between short- and long-term memory networks underlying working memory function, we further explored the dynamics of associative binding between networks.
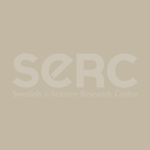
Translational bioinformatics: Statistical learning for patient stratification
Translational bioinformatics was introduced into eCPC during 2014, when formal collaborations with eSSENCE and the SciLifeLab Clinical Diagnostics facility in Uppsala were also initiated.
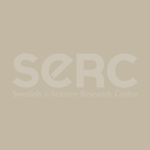
Trial design for prediction
Experimental design is an under-appreciated aspect of data science, where better design leads to more efficient parameter estimation and possibilities to address causality. We will contribute to two new studies:
- the STHLM3-MRI study to assess the combination of the S3M test with magnetic resonance imaging (MRI), and
- the ProBio randomised treatment trial for men with metastatic prostate cancer.
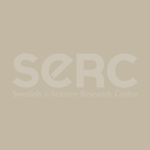
Uncertainty quantification and sensitivity analysis in brain modelling
Uncertainty quantification and sensitivity analysis are important aspects of computational modelling, due to the need to assess the validity and precision of model predictions.
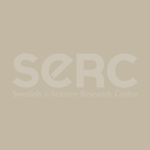
Unifying Memory and Storage with MPI Windows
Computing nodes of next-generation supercomputers will include different memory and storage technologies. We propose a novel use of MPI windows to hide the heterogeneity of the memory and storage subsystems by providing a single common programming interface for data movement across these layers.
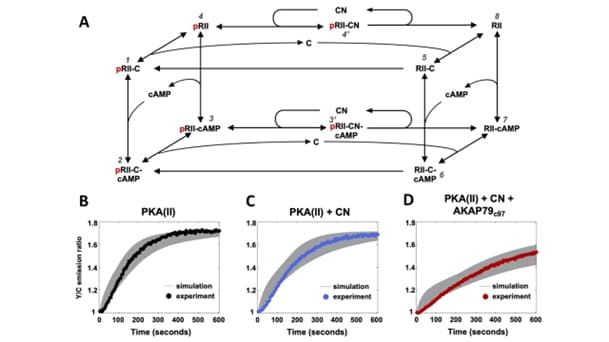
Used the subcellular model building, calibration and analysis toolbox to elucidate the regulatory role of calcineurin for PKA activation
Figure legend: We have built a biochemical model of protein kinase A (PKA) activation by cAMP and its inhibition by calcium activated phosphatases such as calcineurin (CN) using our workflow for modeling and data-driven calibration and analyses of systems biology models.
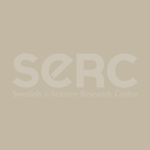
Variational approximations in the medical sciences
In this new sub-project we will develop machine learning models and tools for Gaussian variational approximations (GVAs) and apply those models to health applications.
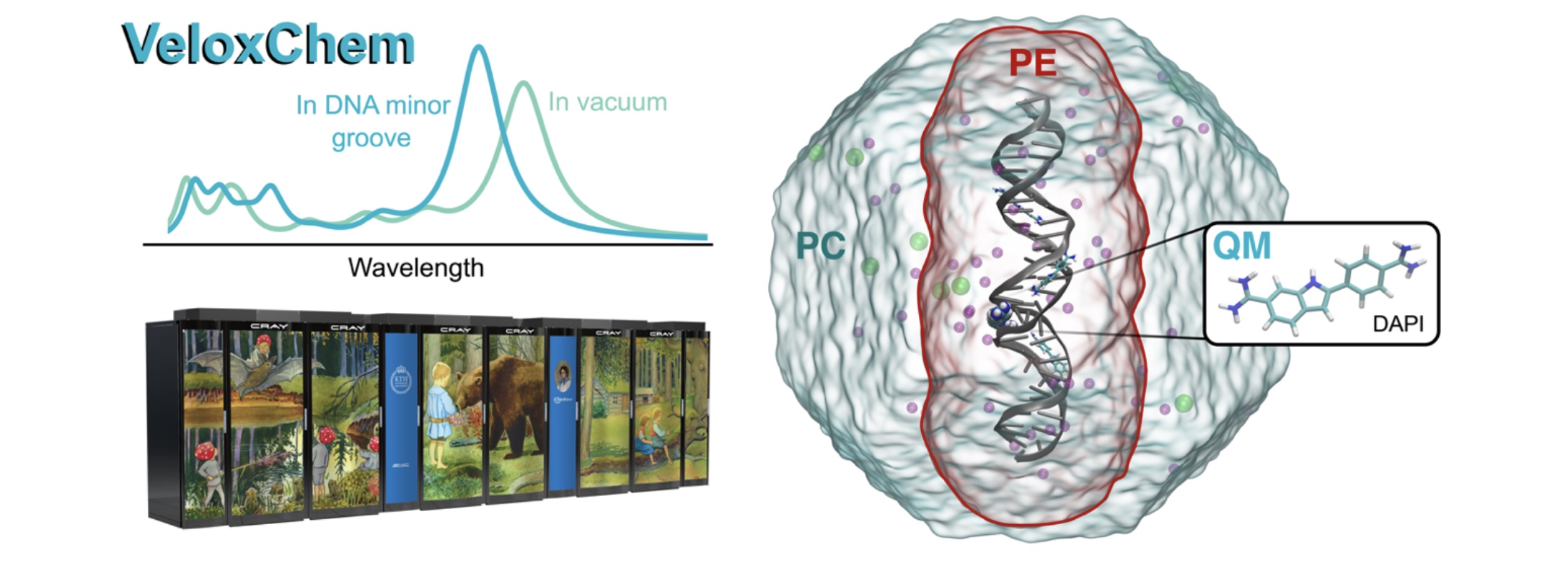
VeloxChem: Enabling Quantum Molecular Modeling in High-Performance Computing Environments
An open-source program named VeloxChem has been developed for the calculation of electronic real and complex linear response functions at the levels of Hartree–Fock and Kohn– Sham density functional theories.
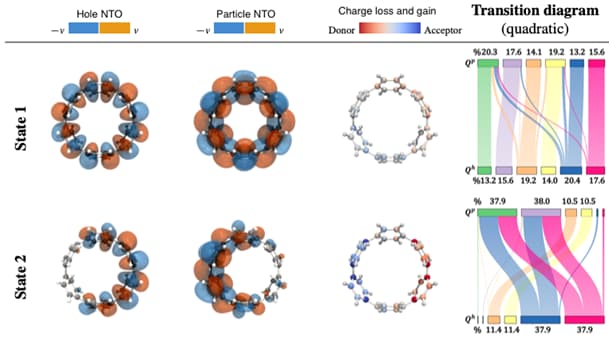
Visual Analysis of Electronic Densities and Transitions in Molecules
The study of electronic transitions within a molecule connected to the absorption or emission of light is a common task in the process of the design of new materials.
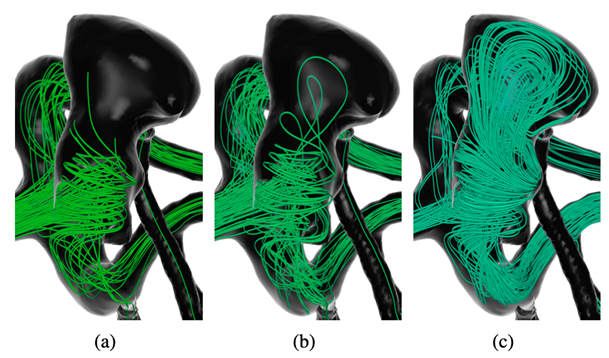
Visual exploration of intracranial aneurysm blood flow used for clinical research
Rupture risk assessment is a key to devising patient-specific treatment plans for cerebral aneurysms.
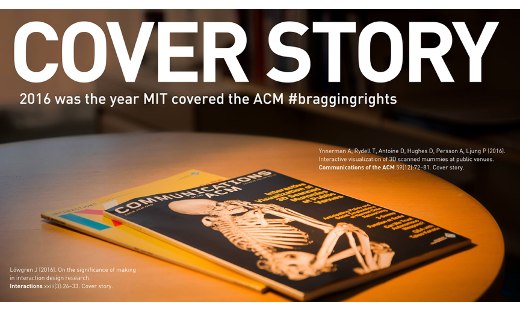
Visualization Highlight: Cover story: Interactive Visualization of 3D Scanned Mummies at Public Venues
By combining visualization techniques with interactive multi-touch tables and intuitive user interfaces, visitors to museums and science centers can conduct self-guided tours of large volumetric image data. …
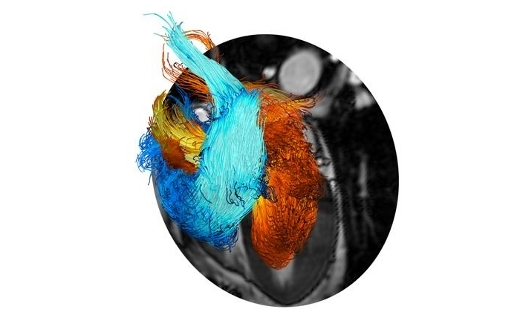
Visualization Highlight: ERC Starting Grant: HEART4FLOW
The objective of the HEART4FLOW project is to develop the next generation of methods for the non-invasive quantitative assessment of cardiac diseases and therapies. …
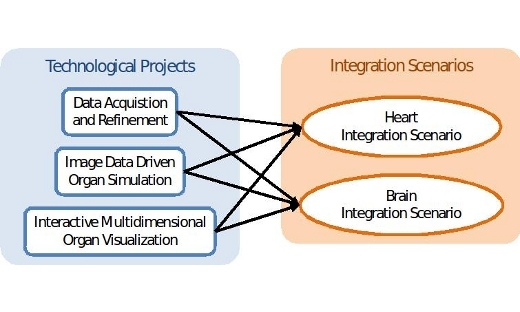
Visualization Highlight: KAW Research Grant: Seeing Organ Function
More recent medical imaging modalities support acquisition of patient-specific functional data embedded in a high-resolution spatial context. …
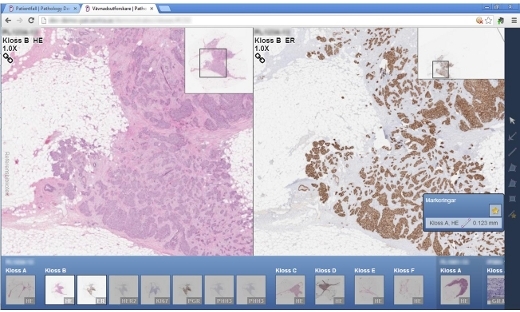
Visualization Highlight: Vinnova Framework Grant: Digital Pathology
Within pathology there is an urgent need for substantially increased efficiency in parallel with continued improvements in quality of care. …
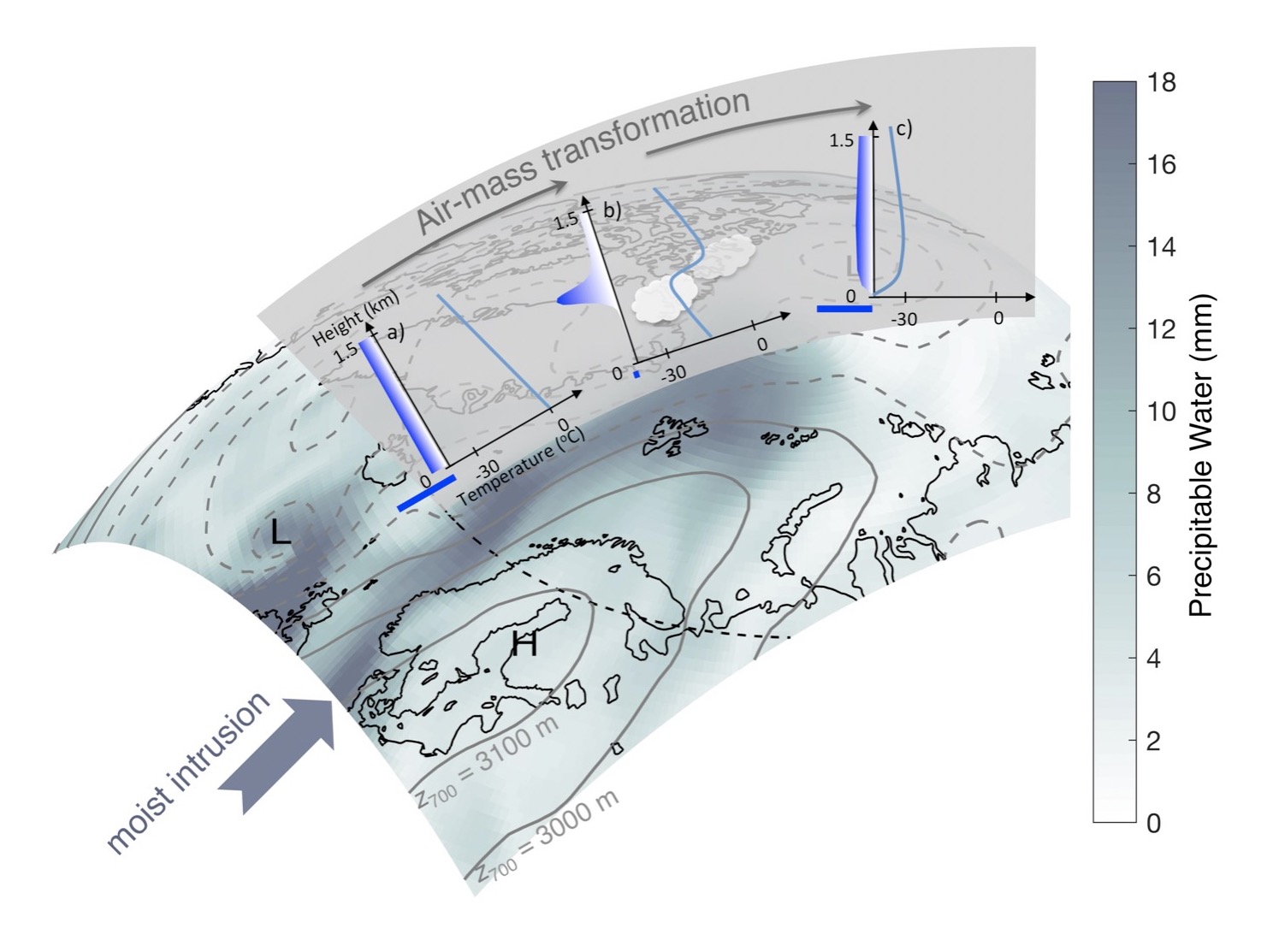
Workshop on Arctic airmass transformations
A workshop organized by Gunilla Svensson, SU, and Felix Pithan, Alfred Wegener Institute, Germany, was held at MISU 6-9 November 2017. The theme of the workshop was how to improve the understanding of air mass transformations in the Arctic by observational and modelling strategies.