Developed methods for discovering cause and effect from medical data
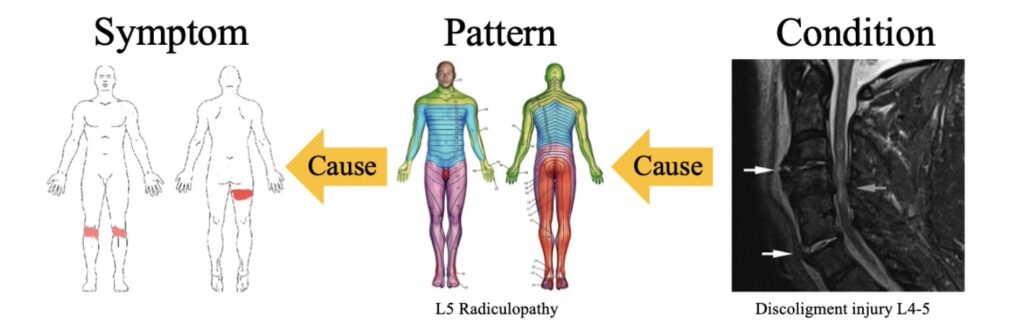
Reasoning about cause and effect is an important aspect of human intelligence, and would thus constitute a valuable part of a medical diagnostics system. Figure 2 shows an example of a causal relationship in pain diagnostics data. However, creating computer methods that learn to differ between cause and effect from just observations is generally impossible. The traditional approach to make causal models is experiments with interventions – introducing different causes explicitly and observing the effect of these. This is often costly and even ethically questionable.
Recently it has been shown that cause-effect relationships can, under certain conditions, indeed be discovered from passively observed data. Based on these findings, SeRC researchers have developed methods for causal discovery from incomplete observations [1] and created models for evaluating and comparing different causal discovery methods [2].
[4] R. Tu, C. Zhang, P. Ackermann, K. Mohan, C. Glymour, H. Kjellström, and K. Zhang. Causal discovery in the presence of missing data. In International Conference on Artificial Intelligence and Statistics, 2019.
[5] R. Tu, K. Zhang, B. C. Bertilson, H. Kjellström, and C. Zhang. Neuropathic pain diagnosis simulator for causal discovery algorithm evaluation. In Neural Information Processing Systems, 2019.
Figure: Cause-effect chain in pain diagnostics