Pioneered the use of recurrent neural networks for temporal predictions in turbulent flows
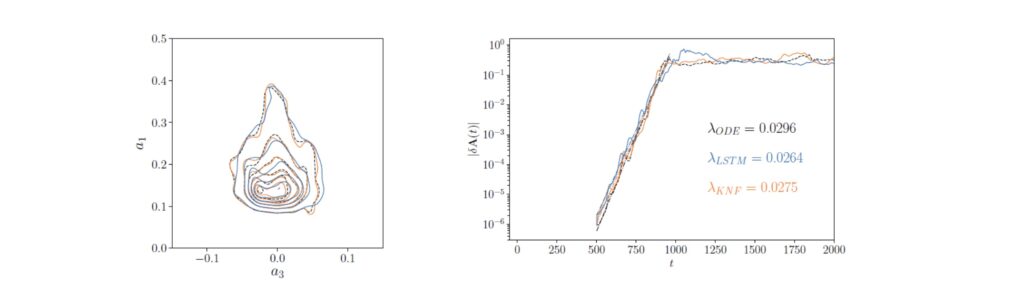
SeRC researchers have assessed the prediction capabilities of long-short-term memory (LSTM) neural networks for predicting the temporal dynamics of a model representing the near-wall cycle of turbulence [1]. Excellent predictions were obtained in terms of turbulence statistics, as well as dynamic behavior characterized through Poincaré maps and Lyapunov exponents (see Figure 1). This has been one of the first studies showing the possibilities of neural networks for predicting turbulence quantities, and it has received quite some attention in the turbulence community. Convolutional neural networks were further applied to estimate flow properties at a certain distance from the wall in an open channel showing improvements over standard linear models [2]. Currently work concerns further developing the prediction framework, and an improved criterion for turbulence-statistics predictions [3] has been established, in addition to assessing the possibilities of using other data-driven approaches to predict the temporal dynamics of turbulence, in particular based on the Koopman operator [3]. The excellent short- and long-term predictions obtained with the latter method paves the way to novel applications in sensing complex flows.
[1] P. A. Srinivasan, L. Guastoni, H. Azizpour, P. Schlatter, and R. Vinuesa. Predictions of turbulent shear flows using deep neural networks. Physical Review Fluids 4:054603, 2019.
[2] L. Guastoni, M. P. Encinar, P. Schlatter, H. Azizpour, and R. Vinuesa. Prediction of wall-bounded turbulence from wall quantities using convolutional neural networks. Preprint, 2019.
[3] H. Eivazi, L. Guastoni, P. Schlatter, H. Azizpour, and R. Vinuesa. Recurrent neural networks and Koopman-based frameworks for temporal predictions in turbulence. Preprint arXiv:2005.02762, 2020.