Electronic Highlight: Machine Learning Energies of 2 Million Elpasolite (ABC2D6) Crystals
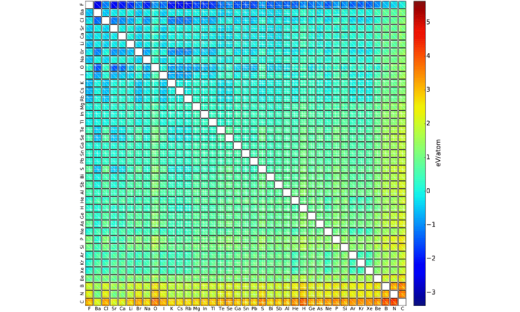
Elpasolite is a common crystal structure. We have developed and trained a machine learning model to predict formation energies of all 2M pristine ABC2D6 elpasolite crystals that can be made up from main-group elements (up to bismuth). The accuracy improves systematically with a larger training set, and reaches the quality of standard quantum-mechanical methods (i.e., density-functional theory) at 10k crystals. The large resulting data set of elpasolite formation energies can be used to examine, e.g., bonding physics accross chemical composition where, e.g., fluoride fits the coordination of the D site and lowers the formation energy whereas the opposite is found for carbon. By combining our data with that available in databases for competing phases, we identified 90 unique structures out of the 2M possibilities to be thermodynamically stable. Among those we found NFAl2Ca6, which has a peculiar stoichiometry and a negative atomic oxidation state for Al.
Matrix of 2M theoretical elpasolite crystals. The y- and x-axes specify two of the four elements in a crystal, which points out a miniature diagram over the remaining two atoms. Every pixel in each miniature then shows the formation energy of that composition on the scale low (blue) to high (red). The formation energy is a key component in determining thermodynamical stability of a material.
References
F. A. Faber, A. Lindmaa, O. A. von Lilienfeld, and R. Armiento, Phys. Rev. Lett. 117, 135502 (2016).