Riemann metric approach to optimal sampling of multidimensional free-energy landscapes
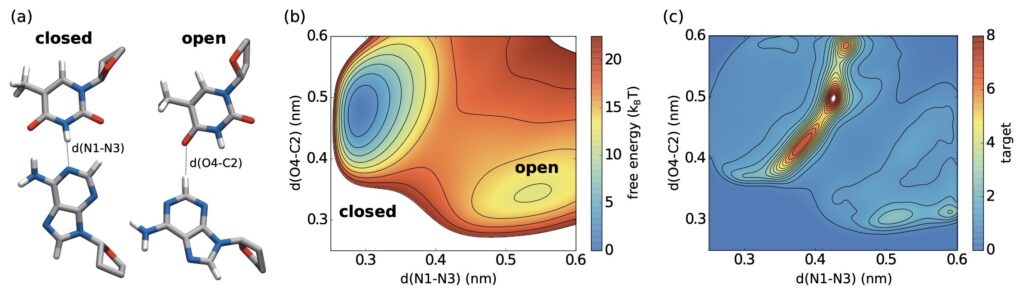
Figure: t DNA base pair opening. The closed, WC state is characterized by low values of d(N1–N3) (left), the distance between the atoms that form the middle WC hydrogen bond. Upon base pair opening, d(N1–N3) increases, which favors a non-WC interaction, characterized by a short distance d(O4–C2). The PMF landscape (b) has two corresponding to the closed state and a second local minimum, corresponding to open conformations. The white region was excluded from sampling by using a free-energy cutoff. The optimized target distribution (c), is sharply peaked in a transition region between the two minima.
Exploring the free-energy landscape along reaction coordinates or system parameters λ is central to many studies of high-dimensional model systems in physics. In simulations this usually requires sampling conformational transitions or phase transitions, but efficient sampling is often difficult to attain due to the roughness of the energy landscape. For Boltz- mann distributions, crossing rates decrease exponentially with free-energy barrier heights. Thus, exponential acceleration can be achieved in simulations by applying an artificial bias along λ tuned such that a flat target distribution is obtained. A flat distribution is however an ambiguous concept unless a proper metric is used, and is generally suboptimal. Here we propose a multidimensional Rie- mann metric, which takes the local diffusion into account, and redefine uniform sampling such that it is invariant under nonlinear coordinate transformations.
We use the metric in combination with the accelerated weight histogram method, a free-energy calculation and sampling method, to adaptively optimize sampling toward the target distribution prescribed by the metric. We demonstrate that for complex problems, such as molecular dynamics simulations of DNA base-pair opening, sampling uniformly according to the metric, which can be calculated without significant computational overhead, improves sampling efficiency by 50–70%.
We investigated the performance, programmability of the tensor cores in the NVIDIA Volta GPU and presented this work at the IPDPS AShES workshop. This work received the best paper award and is receiving a high number of citations.