CausalHealthcare subproject: Using principles from Physics to disambiguate the causal direction between variables in passively recorded data
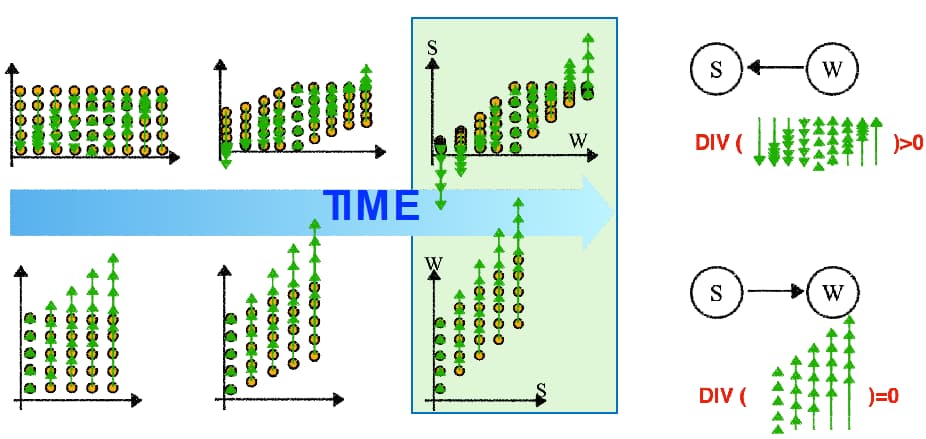
Ruibo Tu, Kun Zhang, Hedvig Kjellström, and Cheng Zhang. Optimal transport for causal discovery. International Conference on Learning Representations, 2022.
Determining cause-effect relationships between underlying variables purely based on passive observations is a challenging machine learning problem with many applications e.g. in medical diagnostics. One method that has been proposed is to make assumptions about the form of the function between these variables, e.g. assuming that one variable is a linear function of the other plus some independent noise; this is called an Additive Noise Model (ANM). However, such model assumptions put a restriction on the range of situation in which it can be used, and are difficult to work with. In this paper, we instead provide an alternative, physics-based approach, and propose a new framework for identifying causal direction in the bivariate case. We first show the connection between FCMs and optimal transport, in short, we determine the underlying dynamical process that would have given rise to the observed cause-effect pair data. It provides a new dimension for describing static causal discovery tasks while enjoying more freedom for modeling the quantitative causal influences. In particular, we show that ANMs correspond to volume-preserving pressureless flows. Consequently, based on the velocity field divergence of the derived dynamical process, we introduce a criterion for determining causal direction. With this criterion, we propose a novel physics-based algorithm for ANMs which is robust to the choice of models. This work was submitted in late 2021 and presented at ICLR in the spring of 2022.