Methods for Precision Health (eMPHasis)
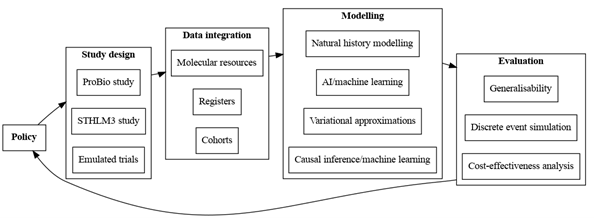
The eMPHasis MCP builds on the previous flagship MCP “eScience for Cancer Prevention and Control” (eCPC). Achievements through eCPC/eMPHasis include: development and commercialisation of the Stockholm3 test for prostate cancer diagnostics; development of artificial intelligence (AI) assisted histopathology for breast and prostate cancer; development of a platform trial for combining novel drug treatments with circulating tumour DNA profiles; development and application of a prostate cancer microsimulation model for planning organised prostate cancer testing; development and estimation of novel breast cancer natural history models; development of new predictive algorithms for breast cancer using novel image analysis features; and, with the Data Science MCP, development of tools based on computer science methodologies for joint models for biomarker and time-to-event data. The eMPHasis MCP has been broadened to include other application domains within precision health, including chronic kidney disease and mental health. The MCP is strategically aligned with other data-driven life sciences activities at Karolinska Institutet (KI), including the Precision Medicine Centre at KI. Importantly, eMPHasis is intended to translate research findings into changes in clinical practice.
Figure: eMPHasis workflow to illustrate the process from study design, data integration modelling, evaluation to policy.
Members
People involved can be found here.
Projects
- Multimodal (next-generation sequencing and imaging) deep learning for prognosis (Martin Eklund, Mattias Rantalainen). This includes methods on artificial intelligence-assisted precision diagnostics for prostate cancer, breast cancer and colorectal cancer.
- Study design, including platform trials for personalised treatment and emulated target trials (Martin Eklund, Erin Gabriel, Els Goetghebeur). This includes methods for the ProBio platform trial (https://www.probiotrial.org/), emulated clinical trials using register-based data, and structural causal models.
- Digital twins for precision health, using individual-based natural history models to investigate AI-assisted disease screening, including effectiveness and cost-effectiveness (Mark Clements, Keith Humphreys). This includes detailed modelling for the natural history of breast cancer, and cost-effectiveness analysis for organised prostate cancer testing.
- Machine learning methods in biostatistics (Mark Clements, Keith Humphreys, Hedvig Kjellström, Erin Gabriel, Els Goetghebeur). This includes causal analysis for precision treatment, the use of variational approximations for joint models, and probabilistic latent variable models.