Method Development for Materials Design (MD2)
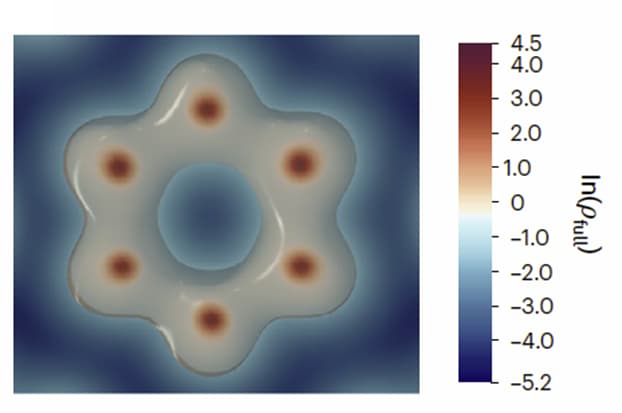
A need for accelerated materials design has brought materials science into the so-called “fourth paradigm” where data-driven methods such as artificial intelligence/machine learning (AI/ML) and knowledge extraction employed to discover new materials and predict their performance in advanced technological applications. Our aim is to enable digital twin modeling in materials design. To make a transformation from simulations to digital twins in materials science, we will develop methodologies that allow to carry out computations at conditions of materials operation in tools and devices, to visualize the results beyond numbers and tables, to couple computational data to real world experimental data in modern databases, and to work out cognitive and visual layers between the twin and a user. We propose to initiate development of this new functionality, ensuring its proof-of-principle status and then moving towards a production-ready feature leveraging the state-of-the art High Performance Computing (HPC) infrastructure accessible within SeRC. These efforts naturally connect to visualization of the obtained results, automatic workflows for robust and reproducible research, and ontology-based normalization of the produced output for improved shareability according to the FAIR principles.
Realistic representations of materials have to account for conditions at which they operate in tools and devices, such as high temperature and stress. The temperature-dependent effective potentials method (TDEP) developed at Linköping University within SeRC will be coupled to advanced visualization of the lattice dynamics, offering an efficient way to introduce temperature effects into a materials digital twin and allowing e.g., for dynamic stability analysis or interactions with physical neutron scattering experiments. Digital twins of magnetic and topological systems are essential for the next generation spintronics. They will account for intriguing properties such as Chern gapped topological fermions, topological Hall effect and a quantum spin liquid state. We will use a biochemical science case to drive a digital twin development in the field. Essential to success are developments facilitating human-steered large-scale data analysis of molecular and electronic structures. We will build up databases of advanced magnetic data such as Heisenberg and Dzyaloshinskii-Moriya interaction parameters, magnetic anisotropy, spin-lattice interaction constants, etc. In collaboration with leading Swedish companies Sandvik Coromant and Seco Tools, as well as VINNOVA supported Competence Centre FunMat-II we will proceed with development of the Hard-coating Alloys DataBase (HADB). We will target our methods to semantically enrich data for storage, search, retrieval, and reasoning to the needs for development and utilization of cognitive materials twins in the MD2 projects.
Figure 1. Visualization of the all-electron charge density calculated from first principles reveals the existence of unexpected aromatic hexazine [N6]4- anion in the complex structure of the high-pressure potassium nitrogen compound K9N56. From D. Laniel, et al., Nature Chem. (2023). https://doi.org/10.1038/s41557-023-01148-7.
Projects
- Digital twins for solid and molecular materials
- New tools for modeling and visualization of lattice dynamics .
- New tools for modeling and visualization of spin dynamics
- New tools for modeling and visualization for biochemical applications
- FAIR databases for solid and molecular materials data
- Database for magnetic properties
- Database of materials properties relevant for industrial partners
- Cognitive layer for Digital Twins for solid and molecular materials
Members
People involved can be found here.