Towards systems network-of-networks modelling of memory phenomena and associative memory bindings
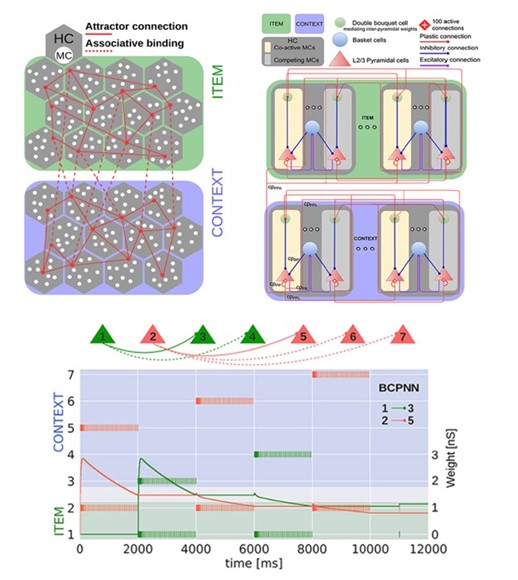
Figure legend: Building on the proposed indexing theory offering a unifying framework for explaining the interactions between short- and long-term memory networks underlying working memory function, we further explored the dynamics of associative binding between networks. This time the major emphasis was on the associative learning that contributes to the formation of long-term episodic memories. In our study we proposed a hypothesis that a well-established psychological effect of memory semantization, i.e. acquisition of semantic memory components from repetitive overlapping episodic memories, could be accounted for synaptic learning described by the Bayesian-Hebbian formalism of our Bayesian Confidence Propagating Neural Network (BCPNN). Consequently, we also studied memory binding underlying olfactory episodic memory in rats, reproducing behavioural results reported in the recent seminal work in the field (not a direct collaborator) and thus offering new mechanistic insights. Both of these computational studies on episodic memory are written up as journal manuscripts.
Finally, we have used the advances on modelling the associative binding between memory networks to provide a simulation framework for the computational work on distributed representations of odor memories. Instead of detailed spiking neural networks we have relied on more abstract models to better capture the nature of experimental data made available in the SeRC collaboration with Jonas Olofsson’s and Maria Larsson’s labs at SU. In order to collect suitable data, a behavioural investigation into the performance of human participants when challenged with an odor naming and identification test has been conducted. In essence, the overarching goal of this collaborative KTH-SU effort is to establish a model that represents the principles of human odor recognition. The empirical data consist mainly of the Swedish National Study on Aging and Cognition – Kungsholmen (SNAC-K) which includes around 2500 individual participants (in collaboration with Erika Jonsson Laukka at Karolinska Institute). These data are complemented by three other datasets. First, a large online survey dataset of about 2000 adult participants who collected ratings about how similar they believed pairs of common odors (e.g. “cinnamon” and “apple”) would be. Second, a psychophysical dataset where 36 participants made detailed ratings of how similar odor pairs (e.g. cinnamon and apple odors) were. The two latter datasets were collected in the lab of Jonas Olofsson and Maria Larsson at SU. Third, a Swedish web corpus where odor-associated words have been identified. The work on selecting odor words in web corpi is led by Thomas Hörberg at SU, following a method developed by Jonas Olofsson, Pawel Herman and colleagues. The datasets can be integrated in order to establish how olfactory naming and identification performance is shaped by long-term memory representations as well as odor perception.
So far, we have found that individual odors are more identifiable and nameable if they have a high intensity, and, provided they can be perceived clearly, unpleasant odors are more distinct and hence more easily remembered. These factors, however, appear to decline in older age, as individual performance data becomes gradually more idiosyncratic and “noisy”. This work is led by Robert Lindroos under the supervision of Jonas Olofsson and Pawel Herman. We have also found that when an odor is mistakenly associated with the wrong label (e.g. cinnamon odor is mistaken for apple), this can be explained by either perceptual similarity, if the label is thought to smell like the odor (as quantified in our survey data), or a semantic similarity, if the odor terms appear in many shared contexts (as determined by the corpus data using a deep learning algorithm). This aspect of the work is led by Rohan Raj under the supervision of Jonas Olofsson and Pawel Herman.
This work is continuously informing the ongoing development of the computational model. At this point, two manuscripts have been completed (see below: Lindroos et al., Raj et al.). Based on this emerging computational framework, we plan to include SNAC-K data on future dementia conversion, such that the model will be able to classify individuals according to their future risk of dementia, based on the odor memory scores, and provide an explanatory framework for this hypothesized relationship.
Publication:
Chrysanthidis, N., Fiebig, F., Lansner, A., & Herman, P. (2021). Semantization of episodic memory in a spiking cortical attractor network model. Journal of Computational Neuroscience, 49(SUPPL 1), S86–S87.
Lindroos, R., Raj, R., Pierzchajlo, S., Hörberg, T., Herman, P., Challma, S., Hummel, T., Larsson, M., Laukka, E., & Olofsson, J.K. (pending revision). Perceptual odor qualities predict successful odor identification in old age. Chemical Senses.
Raj, R., Hörberg, T., Lindroos, R., Larsson, M., Herman, P., Laukka, E., & Olofsson, J.K. (submitted). A new method for understanding age-associated smell loss using perceptual and semantic feature analysis of odor identification errors. Cognition.