Data exploration and visualization
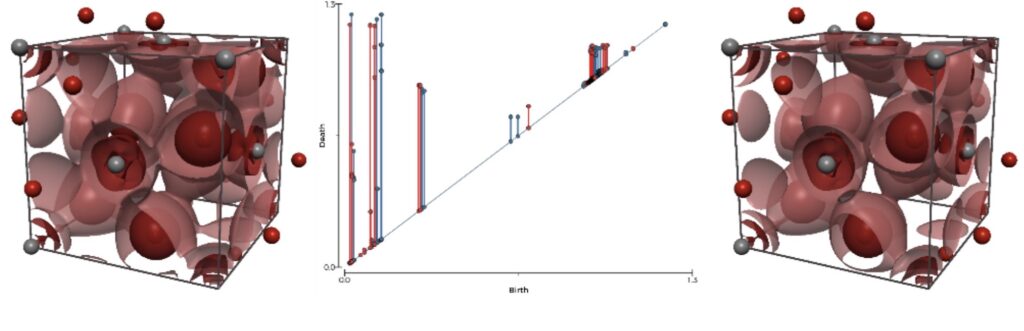
Method development is needed into data exploration and visualization of the generated materials data. This is a new and exciting field of multidisciplinary research where data science meets computational materials physics. Specific activities in this group include:
Theoretical Characterization of Point Defects in Silicon Carbide and Other Materials.
Systematic large-scale study that utilizes the automated workflows and open-data infrastructure in 5.3 to enumerate and theoretically characterize a wide range of point defect properties in SiC, and later extended to other materials. A PhD student supervised by Prof. Abrikosov and co-supervised by Dr. Armiento will be supported by the MCP to address the task.
Data driven materials design with special focus on double perovskites: high-throughput generation of magnetic data for a large set of double perovskites. Analysis and data mining using machine learning techniques. A Postdoc allocated at KTH (Prof. Delin) will be supported by the MCP to address the task and to ensure close collaboration with ML MCP.
Topological fingerprints for detailed visual exploration and generation of feature vectors for automatic learning methods (in collaboration with the visualization MCP): The search for novel materials involves large-scale simulations and experiments to discover possible new material configurations. To fully leverage the potential of the resulting data advanced data analysis methods are essential. This comprises the exploration of the physical properties of the selected material in detail but also to use the gained knowledge to improve the exploration of the large parameter space.
Within this project we plan to exploit methods from topological data analysis (TDA) for this purpose. Topological data analysis provides a powerful concept in reducing data to its structural skeleton based on topological invariants. Such structures have been proven useful to guide visualizations in many applications. However, its special strength are quantitative stability measures of characteristic features. Topological barcodes summarize the topological invariants and their stability and can be interpreted as unique fingerprint of the data. As such they provide an unbiased and mathematically rigorous future vector for the generated fields and are well suited to serve as input for learning methods. Tracing the feature vectors across parameter spaces facilitates interactive visualization of relevant material properties to understand the system dynamics.
While TDA provides a large set of solid concepts for data analysis it specific use and integration depends strongly on the chosen application and the physical properties of interest. Within the DCMD- MCP we will build a topological framework specifically targeted towards the needs of electronic structures simulations. First experiments of applying topological invariants for the analysis of charge density distribution and chemical bonds between atoms have been very encouraging (see Figure). We will therefore focus on exploring the physical interpretation of the TDA applied to electronic density distributions in solids using extensive data collected in materials science data bases and generated in this MCP. We will investigate if the TDA is capable to accelerate applications of the machine learning for materials properties and derivation of materials categories from unsupervised analysis of correlated patterns: (in collaboration with the machine learning MCP). A Postdoc allocated at LiU (supervised by Dr. Armiento, Dr. Herman, Prof. Hotz and Prof. Abrikosov) will be supported by the MCP to address the task.
Fig: Results of topological data analysis of electronic density of FeO2at ultra-high pressure. Calculations by Abrikosovet al., visualization by Hotzet al.