Towards cortex-like neural network architectures for holistic pattern recognition
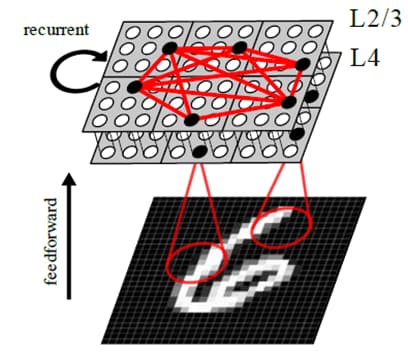
Figure legend: Continuing the major effort in developing a brain-like neural network architecture for holistic pattern recognition the focus has been on evaluating its capability to learn input representations. In particular, building on our work on biologically plausible local Hebbian-like learning and so-called structural plasticity mechanisms, we have devised a feed-forward architecture for unsupervised learning of data representations. Further, we have comprehensively benchmarked the discriminability of the resulting neural codes in a range of machine learning benchmark problems and demonstrated that our approach is on par or compares favorably to the clear majority of emerging brain-like methods and neural networks for unsupervised and semi-supervised learning. This work is intended for a publication in the prestigious Neural Networks journal. In parallel we have developed in a collaboration with the Euro CC National Competence Centre Sweden a new implementation of our algorithms on GPU platforms, which greatly facilitates the simulations on the Euro HPC resources.
We have also started developing a cortex-like neural network architecture for holistic pattern recognition by combining the existing feed-forward network for unsupervised learning of data representations with a recurrent circuitry, considered to underlie canonical associative computations in the brain’s cortical networks. Apart from theoretical advances facilitating the probabilistic interpretation of neural information processing in cortical layers, we have show-cased functional capabilities of such hybrid architectures including pattern completion, prototype extraction and clustering (unsupervised learning) as well as different facets of semi-supervised learning among others. This line of research together with efforts towards deeper cortex-like networks constitute currently our major focus. In parallel, we are also engaged in collaborations that build towards other hardware friendly implementations (memristors, Field Programmable Gate Arrays (FPGAs)) of our algorithms with scalability and energy efficiency in mind
Publications:
Ravichandran, N.B., Lansner, A., Herman, P. (2021a). Brain-Like Approaches to Unsupervised Learning of Hidden Representations – A Comparative Study. In: Farkaš, I., Masulli, P., Otte, S., Wermter, S. (eds) Artificial Neural Networks and Machine Learning – ICANN 2021. Lecture Notes in Computer Science, vol 12895. Springer, Cham.
Ravichandran N. B., Lansner A., Herman P. (2021b). Semi-supervised learning with Bayesian Confidence Propagation Neural Network. 29th European Symposium on Artificial Neural Networks, Computational Intelligence and Machine Learning, 2021. 10.14428/esann/2021.ES2021-156.Wang, D., Xu, J., Stathis, D., Zhang, L., Li, F., Lansner, A., Hemani, A., Yang, Y., Herman, P. and Zou, Z. (2021). Mapping the BCPNN Learning Rule to a Memristor Model. Frontiers in Neuroscience, 15, pp.750458-750458.